Das neue, kompakte Werk des DOTNET-DOKTORs zu .NET 8.0 richtet sich an Umsteigerinnen und Umsteiger von .NET 7.0.
Viele Entwicklerinnen und Entwickler werden in Bezug auf ihre tägliche Arbeitszeit abwertend als "nine-to-five" bezeichnet. Ist das wirklich fair oder sinnvoll?
Das HTML-Rendern mit Razor-Komponenten ist in .NET 8.0 auch außerhalb von Blazor-Anwendungen möglich, beispielsweise für HTML-formatierte E-Mails.
Fast alle Unternehmen setzen auf das Daily als Meetingformat. Und fast alle Dailys sind eine Katastrophe: Langweilig, ineffizient und überflüssig. Warum?
Auf Basis der Klasse TimeProvider kann man sich in .NET 8.0 einen eigenen TimeProvider erstellen, der in Tests Uhrzeiten vorgaukelt.
In den vergangenen Tagen sind wir mit sehr viel Glück dem wohl größten Fiasko in der Geschichte des Internets gerade so entgangen. Wie konnte das passieren?
In .NET 8.0 existiert mit der abstrakten Klasse TimeProvider ein einfacher Weg, Zeitangaben inklusive Zeitzone beliebig im Rahmen von Tests vorzutäuschen.
Microsoft hat in .NET 8.0 die Methoden Parse() und TryParse() in der Klasse System.Net.IPNetwork ergänzt.
Der Dependency-Injection-Container unterstützt nun auch die Angabe von Schlüssel zur Unterscheidung von Instanzen.
Kürzlich wollte ich zu einer bestehenden Tabelle zwei neue Felder hinzufügen. Die Ausführung brach mit einer Fehlermeldung ab. Zusätzlich hat sich die Performance des gesamten Rechners verschlechtert. Aber alles der Reihe nach.
Beim Ausführung einer ALTER TABLE-Query kam in der MySQL Workbench zu folgendem Fehler:
Error Code: 2013. Lost connection to MySQL server during query
Damit wurde nicht angezeigt, dass die Query in ein Timeout läuft, sondern, dass eventuell ein schwerwiegenderes Problem besteht. Die Zeitspanne, nach der diese Meldung kam (30 Sekunden), ließ aber dennoch auf ein Timeout hinweisen.
Nun kann man sich mit dem nachfolgenden Befehl die aktuellen Prozesse auflisten lassen:
show full processlist;
Hier wurde angezeigt, dass die Query noch läuft und Daten in eine Temp-Tabelle kopiert werden. Das sollte meiner Meinung nach, beim Erweitern um zwei Attribute (ohne spezielle Berechnungen etc.) so nicht sein. Zudem lief die Query auch nach 30 Minuten noch. Die Tabelle selbst hat ca. 300.000 Einträge – nicht viel.
Ein
show create table TABELLEN_NAME;
zeigte, dass sich ein Volltext-Index auf der Tabelle befindet. Das lenkte meine Aufmerksamkeit auf sich. Im nächsten Schritt habe ich den Index entfernt:
alter table TABELLEN_NAME drop index INDEX_NAME;
Nun habe ich erneut das ALTER TABLE probiert und siehe da, die lange Ausführungszeit hat sich auf ein Minimum verkürzt.
Daher mein Tipp: Volltext-Index vor einem ALTER TABLE abdrehen, die notwendige Aktualisierung vornehmen und danach den Index wieder neu generieren.
Der Beitrag MySQL: ALTER TABLE extrem langsam erschien zuerst auf Norbert Eder.
If you start developing a ASP.NET Core application you will notice that your site is running under “http s ://localhost:1234” and that your browser is happy to accept it - so there are some questions to be asked.
Why HTTPS on your local dev box?
The first question might be: Why is HTTPS on your local dev box even needed?
At least two reasons for this (from my perspective):
- Browsers love HTTPS nowadays. There are some features, like websockets, that refuse to work with HTTP. I’m not 100% aware of all the problems, but running a webapp under HTTP in 2024 is painful (and rightfully so!).
- Integration with other services is mostly forbidden. If you rely on a 3rd party authentication system (e.g. Microsoft/Facebook/Google/Apple Login) they might accept “localhost” as a reply address, but might deny HTTP addresses.
I wouldn’t count “security” as an issue here, because you are developing on your own system. If there is something on your machine HTTPS won’t help you at that point.
How does ASP.NET Core issues a valid & trusted cert?
I’m not exactly sure when this happens, as it was already installed on my development machine.
Either when you install the Visual Studio workload for ASP.NET Core or if you create your very first ASP.NET Core application the dev cert for localhost will be issued.
But how?
The .NET SDK ships with a CLI tool called dotnet dev-certs https
and this tool issues the certificate.
The output of this command will look like this if a valid and trusted certificate is found::
PS C:\Users\muehsig> dotnet dev-certs https
A valid HTTPS certificate is already present.
dev-certs https
There are other options available:
PS C:\Users\muehsig> dotnet dev-certs https --help
Usage: dotnet dev-certs https [options]
Options:
-ep|--export-path Full path to the exported certificate
-p|--password Password to use when exporting the certificate with the private key into a pfx file or to encrypt the Pem exported key
-np|--no-password Explicitly request that you don't use a password for the key when exporting a certificate to a PEM format
-c|--check Check for the existence of the certificate but do not perform any action
--clean Cleans all HTTPS development certificates from the machine.
-i|--import Imports the provided HTTPS development certificate into the machine. All other HTTPS developer certificates will be cleared out
--format Export the certificate in the given format. Valid values are Pfx and Pem. Pfx is the default.
-t|--trust Trust the certificate on the current platform. When combined with the --check option, validates that the certificate is trusted.
-v|--verbose Display more debug information.
-q|--quiet Display warnings and errors only.
-h|--help Show help information
What happens when the cert is no longer valid?
This is an interesting one, because I had this experience just this week (and that’s the reason for this blogpost).
A certificate needs to be in the certification store to be considered trusted. That means your “localhost”-dev cert will be stored in your personal certification store (at least on Windows):
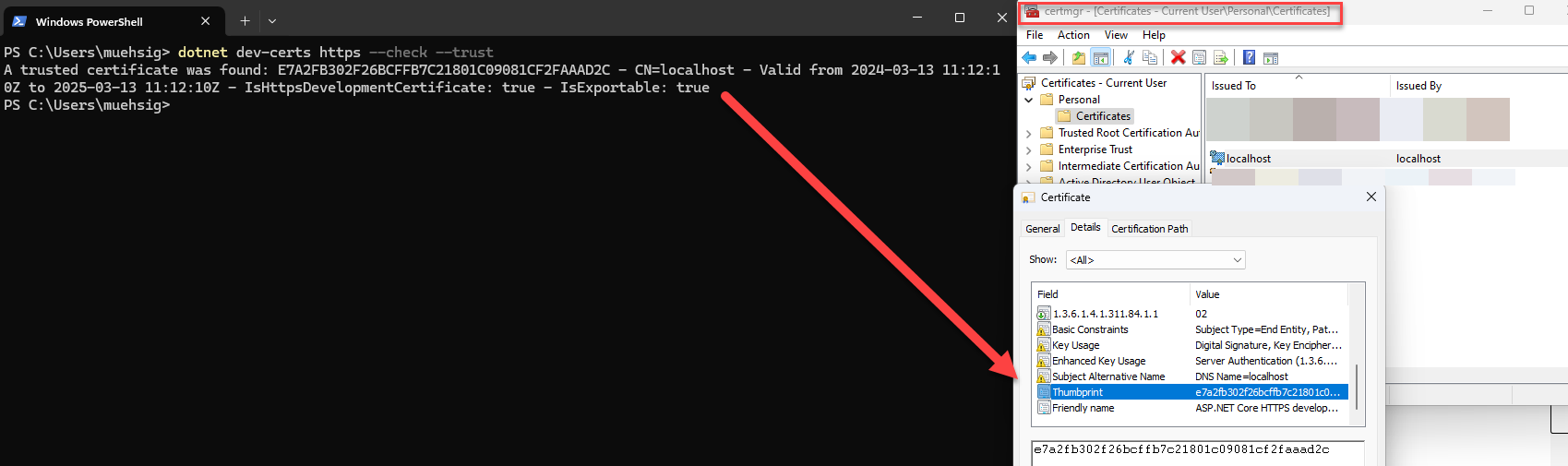
As you can see, the command dotnet dev-certs https --check --trust
will return something like this:
A trusted certificate was found: E7A2FB302F26BCFFB7C21801C09081CF2FAAAD2C - CN=localhost - Valid from 2024-03-13 11:12:10Z to 2025-03-13 11:12:10Z - IsHttpsDevelopmentCertificate: true - IsExportable: true
If the certificate is stale, then your browser won’t accept it anymore and your web application will start, but can’t be viewed because your browser will refuse it.
How to repair invalid certificates?
Use the two commands and it should work again:
dotnet dev-certs https --clean
…which will remove the old certification and…
dotnet dev-certs https --trust
… to issue a new cert and invoke the trust dialog from Windows.
If it works…
There are some more options, e.g. to export the certificate, which can be useful in certain scenarios, but if you can use HTTPS on your local development machine and everything works you shouldn’t have to bother.
If you want to learn more, checkout the dotnet dev-certs documentation.
Hope this helps!
.NET 8.0 bietet neue Hilfsmethoden zum Auslösen von Fehlern bei falschen Parametern.
The Formula 1 season continues its excitement with the Jeddah Grand Prix in Saudi Arabia’s coastal city. This event follows the season opener in Bahrain and precedes the race in Melbourne, Australia. The night race at the Jeddah Corniche Circuit promises to be a highlight of the 2023 season.
Jeddah Corniche Circuit: A Marvel of Engineering
Stretching 6.175 kilometers along the Red Sea coast, this street circuit with its 27 turns is a test of skill for drivers, ranking as the second longest in the Formula One calendar. The track, set against a backdrop of iconic structures like the Jeddah Tower and King Fahd Fountain, seamlessly integrates into the city’s landscape. However, the future might see Formula 1 shifting to Riyadh, with the Qiddiya Entertainment Complex slated to become the new venue from 2024, hosting various motorsport and entertainment events.
Champions of the Jeddah Grand Prix
In its third year, the Jeddah Grand Prix has seen notable winners:
- 2021: Lewis Hamilton (Mercedes)
- 2022: Max Verstappen (Red Bull)
- 2023: Sergio Perez (Red Bull)
Formula 1’s Ties with Saudi Arabia: A Blend of Progress and Controversy
Saudi Arabia’s foray into Formula One, while marked with significant investments and sponsorships, has not been free of controversy. The nation’s conservative Islamic culture juxtaposes with the sport’s global appeal. Nonetheless, the huge investments, including a $900 million deal for a ten-year hosting agreement, showcase Saudi Arabia’s commitment to making its mark in Formula One.
Beyond the Racetrack: Human Rights and Change
Amidst the glamour of Formula One in Saudi Arabia, concerns over human rights persist. Figures like Lewis Hamilton have used their influence to bring attention to these issues, advocating for movements like LGBT rights. The impact of Formula One in driving social change in Saudi Arabia remains an open question.
Formula 1 in Jeddah: An Intriguing Evolution
Saudi Arabia’s journey in the world of Formula One is multi-faceted. With the Jeddah Corniche Circuit and the upcoming Qiddiya Entertainment Complex, the country is redefining motorsport standards. Yet, the integration of sports with the broader social context in Saudi Arabia is a complex and evolving narrative.
Exploring Jeddah: A City of Wonder
Jeddah, a major Saudi Arabian city, offers a blend of historical, cultural, and modern attractions. Highlights include the scenic Jeddah Corniche, the record-breaking King Fahd Fountain, the under-construction Jeddah Tower, the historical Al-Balad district, Nasseef House, the Floating Mosque, Jeddah Aquarium, King Fahd Mosque, the expansive Red Sea Mall, Fakieh Aquarium, Atallah Happy Land Park, and the pioneering King Abdullah Economic City.
These attractions contribute to Jeddah’s unique allure, blending tradition with modernity.
Formula 1 Fans: Watch the Race for Free
For Formula 1 enthusiasts eager to catch the action, including the Saudi Arabian race, free F1 live streaming is possible. By using a simple trick, fans can enjoy the races live without any cost.
The post Jeddah GP: A New Chapter in Formula 1, Saudi Arabia appeared first on xplatform.rocks.
The Formula 1 Miami Grand Prix, set to illuminate the Florida skies from May 5-7, 2023, marks the event’s second appearance on the F1 calendar. This thrilling night race, held in the vibrant state of Florida, USA, is not just a testament to the region’s growing significance in Formula 1 but also a showcase of high-octane excitement.
From Baku to Miami: The F1 Journey Continues
Following the Baku Grand Prix in Azerbaijan, Formula 1 teams embarked on a lengthy journey to Miami, a routine part of their global travels. After the Miami event, the entourage will proceed to the Italian Grand Prix in Imola, maintaining the fast-paced schedule of the Formula 1 season.
Miami Grand Prix’s Heartbeat: The Miami International Autodrome
At the core of the Miami Grand Prix’s excitement lies the Miami International Autodrome. This track, encircling the Miami Dolphins’ Hard Rock Stadium, temporarily transforms the NFL arena into a racing spectacle, offering an adrenaline-pumping experience for Formula One enthusiasts.
The Thrilling Circuit: Challenging and Fast-Paced
Known as the Hard Rock Stadium Circuit, this 5.41 km track with its 19 demanding turns demands precision and strategic mastery from the drivers. With top speeds reaching 320 km/h and a counterclockwise direction, it guarantees a thrilling racing adventure.
Safety First: F1’s Commitment to Secure Racing
Developed to meet FIA’s stringent safety standards, the Hard Rock Stadium Circuit ensures a secure yet exhilarating racing experience. Close coordination between race organizers and security teams guarantees optimal safety for both drivers and spectators.
A Decade of Racing: The Long-term Vision
The ten-year contract with Formula One heightens the anticipation, offering drivers, both veteran and emerging, ample opportunities to master the track and showcase their prowess.
Beyond Racing: Miami Grand Prix’s Cultural Impact
The Miami Grand Prix goes beyond racing, highlighting the Hard Rock Stadium’s versatility as a venue for diverse events, from the Miami Dolphins’ games to the ATP Tennis Miami Open and major concerts, embodying Miami’s dynamic cultural scene.
Miami: A City of Diverse Attractions
Miami, a city of stark contrasts, captivates visitors worldwide with its stunning beaches, vibrant nightlife, and rich culinary landscape. From art galleries and luxury shopping to lush parks and a myriad of activities, Miami is a city where excitement and relaxation seamlessly merge.
Discover Miami: A City Full of Surprises
While the Miami Grand Prix is a highlight, Miami’s charm extends beyond the race track. The city offers a rich tapestry of experiences ranging from iconic beaches to cultural landmarks and natural wonders.
Miami’s Must-Visit Destinations:
- South Beach: Famous for its white sands, turquoise waters, and lively vibe, plus the iconic Art Deco architecture.
- Art Deco Historic District: Houses a vast collection of 1920s and 1930s Art Deco buildings.
- Little Havana: The heart of Cuban culture in Miami with authentic experiences.
- Vizcaya Museum and Gardens: A former industrialist’s estate, now a European art museum.
- Wynwood Walls: A neighborhood celebrated for its vibrant street art.
- Miami Seaquarium: A chance to interact with marine life.
- Jungle Island: A combination of a zoo and botanical garden with exotic wildlife.
- Everglades National Park: A unique ecosystem offering airboat tours and alligator sightings.
- Miami Design District: Known for luxury shopping, art galleries, and trendy dining.
- Bayfront Park: A public park hosting various events and offering scenic bay views.
- Coral Gables: Notable for Mediterranean architecture and the historic Biltmore Hotel.
- Perez Art Museum Miami (PAMM): Showcases modern and contemporary art.
- Zoo Miami: Florida’s largest zoo, emphasizing natural habitats.
- Coconut Grove: A quaint area with charming shops, cafes, and galleries.
For those planning to attend the Miami Grand Prix, the city is well-connected, with multiple transport options including flights, trains, buses, and cars. Visitors should anticipate warm weather, typical of Miami, but also be prepared for occasional rain.
Experience the Miami Grand Prix from Anywhere
For fans unable to witness the race in person, the entire Formula One season, including the Miami Grand Prix, is available for streaming online. This allows enthusiasts worldwide to partake in the excitement from the comfort of their homes.
The post F1 Miami Grand Prix: A Dazzling Event in the Sunshine State appeared first on xplatform.rocks.
Following a brief pause in 2020 and 2021, owing to the worldwide coronavirus crisis, the Australian Melbourne Grand Prix made a triumphant return to the racing schedule in 2022. The 2023 event is poised for an early April launch, a mere fortnight after the Jiddah Grand Prix in Saudi Arabia. Enthusiasts eagerly anticipate the subsequent racing spectacle at the Azerbaijan GP in Baku.
Melbourne Grand Prix: Exploring the Albert Park Circuit
Set to take place at the Albert Park Circuit in Melbourne, this race features one of Formula One’s quicker tracks. Famed for its numerous high-speed stretches and 16 challenging corners, some forming acute 90-degree angles, the circuit demands exceptional precision in steering and acceleration from the drivers.
This 5.303-kilometer track, notorious for its rough surfaces causing top drivers to lose crucial points and standings, runs clockwise and spans 58 laps, totaling a race distance of 307.5 kilometers.
Melbourne Grand Prix – Records and Rivalries
Michael Schumacher’s 2004 record of 1:24.125 remains the fastest race lap, while Lewis Hamilton’s 2019 lap at 1:20.486 stands as the quickest ever. The circuit’s layout results in high fuel consumption, with over two-thirds of the lap at full throttle, increasing tire and brake wear. Additionally, Melbourne’s unpredictable weather adds an extra layer of complexity, testing the mettle of drivers and their technical teams.
The Melbourne Grand Prix: A Retrospective
Since its inception in 1985, the Australian Grand Prix has witnessed numerous iconic moments, particularly in 1994 and 1995 when Michael Schumacher clinched back-to-back world titles. The Melbourne Grand Prix boasts an impressive roster of victors, with Charles Leclerc winning in 2022, and no races held in 2020 and 2021 due to the pandemic.
Celebrated Champions of the Melbourne Grand Prix:
Year | Winner | Location |
---|
2022 | Charles Leclerc Ferrari | |
2021 | No Grand Prix race | Melbourne GP |
2020 | No Grand Prix race | Melbourne GP |
2019 | Valtteri Bottas Mercedes | Melbourne GP |
2018 | Sebastian Vettel Ferrari | Melbourne GP |
2017 | Sebastian Vettel Ferrari | Melbourne GP |
2016 | Nico Rosberg Mercedes | Melbourne GP |
2015 | Lewis Hamilton Mercedes | Melbourne GP |
2014 | Nico Rosberg Mercedes | Melbourne GP |
2013 | Kimi Räikkönen Lotus Renault | Melbourne GP |
2012 | Jenson Button McLaren Mercedes | Melbourne GP |
2011 | Sebastian Vettel Red Bull Renault | Melbourne GP |
2010 | Jenson Button McLaren Mercedes | Melbourne GP |
2009 | Jenson Button McLaren Mercedes | Melbourne GP |
2008 | Lewis Hamilton McLaren Mercedes | Melbourne GP |
2007 | Kimi Räikkönen Ferrari | Melbourne GP |
2006 | Fernando Alonso Renault | Melbourne GP |
2005 | Giancarlo Fisichella Renault | Melbourne GP |
2004 | Michael Schumacher Ferrari | Melbourne GP |
2003 | David Coulthard McLaren Mercedes | Melbourne GP |
2002 | Michael Schumacher Ferrari | Melbourne GP |
2001 | Michael Schumacher Ferrari | Melbourne GP |
2000 | Michael Schumacher Ferrari | Melbourne GP |
1999 | Eddie Irvine Ferrari | Melbourne GP |
1998 | Mika Häkkinen McLaren Mercedes | Melbourne GP |
1997 | David Coulthard McLaren Mercedes | Melbourne GP |
1996 | Damon Hill Williams Renault | Melbourne GP |
1995 | Damon Hill Williams Renault | Adelaide GP |
1994 | Nigel Mansell Williams Renault | Adelaide GP |
1993 | Ayrton Senna McLaren Ford | Adelaide GP |
1992 | Gerhard Berger McLaren Honda | Adelaide GP |
1991 | Ayrton Senna McLaren Honda | Adelaide GP |
1990 | Nelson Piquet Benetton Ford | Adelaide GP |
1989 | Thierry Boutsen Williams Renault | Adelaide GP |
1988 | Alain Prost McLaren Honda | Adelaide GP |
1987 | Gerhard Berger Ferrari | Adelaide GP |
1986 | Alain Prost McLaren Porsche | Adelaide GP |
1985 | Keke Rosberg Williams Honda | Adelaide GP |
Melbourne and its Must-Visit Attractions
Melbourne, known for its eclectic culture and architecture, offers a plethora of attractions. Highlights include the vibrant Federation Square, the lush Royal Botanic Gardens, the iconic Melbourne Cricket Ground (MCG), the historic National Gallery of Victoria (NGV), and the poignant Shrine of Remembrance. The Eureka Tower offers stunning city views, while the Melbourne Zoo and Aquarium provide unique wildlife experiences. The bustling Queen Victoria Market, scenic Great Ocean Road, and artistic laneways add to the city’s charm, with Phillip Island being a haven for wildlife enthusiasts.
- Federation Square: This bustling public square stands as a central venue for cultural events and social gatherings, pulsating with life and activity.
- Royal Botanic Gardens: Nestled in the city’s heart, this expansive garden boasts a stunning collection of flora, offering a serene escape amidst nature.
- Melbourne Cricket Ground (MCG): Renowned worldwide, the MCG is steeped in sporting history, hosting numerous legendary sporting events.
- National Gallery of Victoria (NGV): As Australia’s oldest art gallery, the NGV houses an awe-inspiring array of artworks, a testament to the nation’s rich artistic heritage.
- Shrine of Remembrance: This solemn war memorial honors the valor and sacrifice of Australians who served in wars, a poignant reminder of the country’s history.
- Eureka Tower: Dominating Melbourne’s skyline, this skyscraper offers stunning panoramic city views, with its skydeck being a major attraction.
- Melbourne Zoo: A family favorite, the zoo is home to a diverse range of wildlife, providing an engaging and educational experience for all ages.
- Melbourne Museum: Showcasing the region’s history, culture, and natural science, this modern museum captivates visitors with its informative and interactive exhibits.
- St. Kilda Beach: A popular coastal spot, known for its vibrant promenade, array of restaurants, and lively atmosphere.
- Melbourne Aquarium: Dive into an underwater journey here, featuring a rich variety of marine life, including the majestic sharks and playful penguins.
- Queen Victoria Market: A bustling hub offering an eclectic mix of fresh food, apparel, and souvenirs, reflecting Melbourne’s vibrant market culture.
- Great Ocean Road: A scenic drive just outside Melbourne, renowned for its breathtaking coastal views and natural beauty, perfect for a day trip.
- Flinders Street Railway Station: An architectural icon and a major transportation hub, this station is a central point in Melbourne’s bustling city life.
- Laneways and Arcades: Explore Melbourne’s unique character through its artistic laneways, brimming with cozy cafes, boutique shops, and vibrant street art.
- Phillip Island: Famous for its penguin parade, this island offers a chance to encounter koalas, seals, and other native wildlife in their natural habitat.
- Yarra River: Winding through the city, the river provides a picturesque setting for boat rides and picnics, adding to the city’s charm.
- State Library Victoria: More than just a library, this institution boasts rich history and stunning architecture, making it a landmark of knowledge and beauty.
- Lygon Street: The heart of Italian culture in Melbourne, filled with delectable restaurants and cafes, perfect for food enthusiasts.
- Chapel Street: A go-to destination for shopping and nightlife, offering a diverse range of stores and entertainment options.
- Dandenong Ranges: Close to Melbourne, these ranges offer outdoor adventures like hiking and picnicking, including rides on the historic Puffing Billy steam train.
These attractions showcase Melbourne’s vibrant mix of culture, history, art, gastronomy, and nature, making it a captivating destination to explore.
Experience Formula 1 Live Without Subscriptions
For those keen on experiencing the full Formula 1 season live without a paid Sky subscription, there are alternative, subscription-free methods. This option serves as a budget-friendly alternative to costly pay-TV services, allowing fans to enjoy the thrill of Formula 1 racing without added expense.
The post F1 Melbourne – Grand Prix Australia appeared first on xplatform.rocks.
As the Formula One season unfolds with thrilling races, the Baku Grand Prix in Azerbaijan, situated along the Caspian Sea, is set to capture the world’s attention in late April. Following the exhilarating race in Melbourne, the Formula One journey continues, leading up to another exciting event – the Miami Grand Prix. Fans of the sport are in for a succession of spectacular races.
Baku Grand Prix: A Blend of History and Speed
The Baku City Circuit, the official name of Baku’s racetrack, made its memorable debut in 2017. This track stands out in the Formula One circuit for its sheer beauty and challenge. Daniel Ricciardo clinched a remarkable win in his Red Bull in its inaugural year, while Lewis Hamilton, despite starting in pole position, finished without a victory.
Traversing through Baku’s heart, the track passes by significant landmarks like the Maiden’s Tower and weaves through the Old Town. It stretches along the seafront, ending at Government Square. This 6.006-kilometer circuit, with its narrowest point being just 7 meters wide, is often cited as one of the most demanding in the sport. The limited overtaking opportunities, especially in turns 7 and 16, place a premium on starting positions in the 51-lap race.
Baku: A City of Stunning Contrasts
Baku, Azerbaijan’s capital, is a tapestry of old and new, a blend of diverse cultures and eras. Situated on the western shore of the Caspian Sea, it serves as the country’s political, economic, and cultural nucleus. This city captivates visitors with its mix of ancient heritage and modern vibrancy, offering something for everyone – from Formula 1 enthusiasts to history and architecture lovers.
A Journey Through Time and Architecture
Baku’s history is rich and varied, dating back to the first millennium BC. Influences from various empires, including the Persians, Romans, and the Soviet Union, are evident in its architecture and culture. The Old City, or İçəri Şəhər, a UNESCO World Heritage Site, houses landmarks like the Maiden’s Tower and the Palace of the Shirvanshahs.
In the realm of contemporary architecture, Baku is equally impressive. The Flame Towers, designed to resemble flickering flames, dominate the city’s skyline. The Heydar Aliyev Center, a creation of the famed Zaha Hadid, is another architectural gem with its flowing, futuristic design.
Economic Growth and Cultural Fusion
Baku’s economy thrives primarily on its oil and gas industry, propelling it into a significant global energy hub. This economic boom has spurred growth in sectors like technology, tourism, finance, and sports, including Formula One.
The cultural scene in Baku is a fascinating fusion of Eastern and Western influences. From traditional Azerbaijani music and dance to European art and literature, the city is a hub of cultural diversity. It boasts an array of museums, galleries, and theaters showcasing both local and international works.
A Tourist’s Delight
Baku attracts a global audience with its blend of historical and modern attractions. The city offers a wide range of experiences, from the quaint cobbled streets of the old city to contemporary shopping and dining options. Key tourist spots include the Tezepir Mosque, Gobustan National Park with its ancient petroglyphs, and the scenic waterfront.
Climate Patterns
Baku experiences a semi-arid climate with significant temperature variations between summer and winter. Summers are typically warm, averaging around 26°C in June, while winters are generally mild.
Recapping the Baku Grand Prix History
The Baku Grand Prix has seen a variety of winners since its inception, for example:
- 2017: Daniel Ricciardo (Red Bull-TAG Heuer)
- 2018: Lewis Hamilton (Mercedes)
- 2019: Valtteri Bottas (Mercedes)
- 2021: Sergio Pérez (Red Bull Racing-Honda)
- 2022: Max Verstappen (Red Bull Racing-Honda)
Experience the Baku Grand Prix from Home
For fans eager to catch the Baku Grand Prix and other F1 races live, there’s a way to enjoy it for free. By using a reliable VPN, you can access live streams of all the Formula One races. This method, which I have relied on for years, brings the excitement of the race track right into your home.
The post The Thrill of F1 in Azerbaijan: Baku Grand Prix appeared first on xplatform.rocks.
The Imola Grand Prix, also known as the Emilia-Romagna Grand Prix, is set to exhilarate fans for the fourth time this year. Previously celebrated as the San Marino Grand Prix, the 2023 event will unfold in mid-May, following the Miami Grand Prix in the USA. The iconic Italian race in Imola will precede the prestigious Monaco GP in Monte Carlo.
A Glimpse into the Past of the Imola Grand Prix
This year marks the return of the Imola GP to the Formula One calendar, a race historically known as the San Marino Grand Prix. In earlier times, each country was limited to hosting just one Formula One race, leading to its assignment to the small, Italy-encircled state of San Marino, nestled between Emilia-Romagna and the Marches.
The Autodromo Imola, a mere 80 km from the Scuderia headquarters in Maranello, is often referred to as Ferrari’s home race, adding to its prestige and significance in the racing world.
The Legendary Imola Circuit: Dino e Enzo Ferrari
The circuit, officially known as the Autodromo Enzo e Dino Ferrari, has been a Formula One venue since 2020. Known as the San Marino Grand Prix until 2006, it was temporarily dropped from the calendar due to its then-outdated facilities.
Historically, the Imola circuit was notorious for its danger, highlighted by the tragic accidents that claimed the lives of Ayrton Senna and Roland Ratzenberger in 1994. Over the years, significant changes were made to the Tamburello and Tosa corners, and the Variante Alta and Variante Bassa chicanes, greatly enhancing safety. These modifications have allowed the track to reclaim its spot in Formula One as the Emilia-Romagna Grand Prix since 2020.
Imola Grand Prix Circuit: By the Numbers
The Autodromo Dino e Enzo Ferrari, a counterclockwise circuit, stretches over 4.909 km and includes 17 turns. The race spans 63 laps, covering a total distance of 309.049 km. The lap record is held by Lewis Hamilton (Mercedes) with an impressive time of 1:15.484.
Recent winners include Lewis Hamilton (Mercedes, 2020) and Max Verstappen (Red Bull Honda, 2021 and 2022).
Emilia-Romagna: Italy’s Gem
The region of Emilia-Romagna in Italy is a treasure trove of experiences, from its celebrated Italian cuisine to breathtaking natural landscapes and historical sites. Known for balsamic vinegar, Parmesan cheese, pasta, tortellini, and prosciutto di Parma, the region is a food lover’s paradise. Motorsport enthusiasts can revel in the Formula 1 race at the Imola circuit and visit the renowned Ferrari Museum in Maranello. The region also boasts natural beauty, historical ruins, cultural events, and festivals, making it an attractive destination for travelers worldwide.
The Emilia-Romagna region in Italy is a mosaic of delightful experiences and attractions:
- Culinary Delights: Celebrated for its exquisite Italian cuisine, Emilia-Romagna is the birthplace of culinary treasures such as balsamic vinegar, Parmesan cheese, as well as popular foods like pasta, tortellini, and prosciutto di Parma.
- Formula 1 Racing: This region is also a haven for Formula 1 enthusiasts, hosting the thrilling Imola Grand Prix at the renowned Autodromo Enzo e Dino Ferrari in Imola.
- Ferrari Museum: For automotive fans, the Ferrari Museum in Maranello is a must-visit. It offers a deep dive into the storied history and captivating designs of the iconic Ferrari brand.
- Natural Beauty: Emilia-Romagna boasts a diverse landscape, with its picturesque rivers, lakes, and mountains, making it a perfect destination for nature lovers to explore through hiking, biking, and other outdoor activities.
- Historical Sites: Rich in history, the region is dotted with numerous historical landmarks, including ancient ruins and UNESCO World Heritage Sites. A notable example is the Roman amphitheater in Parma.
- Cultural Events: The region is also a hub for cultural richness, hosting a variety of events, festivals, and functions that draw visitors from across the globe, offering a glimpse into its vibrant cultural tapestry.
Free Viewing of the Emilia-Romagna Formula 1 Race
Did you know that all Formula 1 races, including the Imola Grand Prix, can be streamed live for free? With a stable internet connection (minimum 5 Mbps) and a reliable VPN, you can enjoy the thrill of Formula 1 racing from anywhere in the world.
The post F1 Italy in Imola GP – Emilia Romana appeared first on xplatform.rocks.
Eine Menge des Typs FrozenSet gewinnt beim tausendmaligen Aufruf von Contains() gegenüber anderen Objektmengen.
Monte Carlo Grand Prix: The Formula One season’s seventh round is set to dazzle in Monaco from May 26 to 28, 2023. In a notable update, Sebastian Vettel, Germany’s long-anticipated world champion, has opted out of the race this year. Vettel, the esteemed driver from Heppenheim, had previously declared in 2022 that he wouldn’t be competing in another Formula 1 season.
Monte Carlo Grand Prix: The Circuit Explored
The Monaco circuit, a temporary track, snakes through the scenic locales of Monte Carlo and La Condamine in Monaco. Famous for hosting the Monaco Grand Prix, this track sees various city streets transformed annually into a racing haven. This transformation is no small feat, involving the removal of traffic signs and flower pots. The track, spanning 3.337 kilometers, is equipped with pit lanes, guardrails, movable fences, and impressive grandstands. It features 19 challenging turns, adding to the race’s excitement. Racers will complete 78 laps during the event, covering a total distance of 260 kilometers.
This street circuit is known for its limited run-off areas, making it one of the most perilous in the Formula One roster, despite lower average speeds compared to other tracks. Former world champion Nelson Piquet likened racing here to “flying a helicopter in your living room,” while David Coulthard, a two-time winner at Monaco, called it “pure madness.” Winning in Monaco is a testament to a driver’s precision, bravery, and ability to push boundaries.
A unique aspect of the Monaco circuit is its pit lane. Due to Monaco’s specific constraints, the pit lane wasn’t initially planned and is quite narrow, challenging teams to manage their equipment effectively. The pit building is adjacent to the start-finish straight, and since 2004, the pit lane has run behind it. This layout demands exceptional adaptability and skill from teams and drivers during pit stops.
The Circuit de Monaco’s Storied Past
Debuting in 1929 for the Monaco Grand Prix, organized by the Automobile Club de Monaco, the Circuit de Monaco boasts a rich history. Formula One engines roared through Monaco’s streets for the first time in 1950, marking the principality’s inclusion in the Formula One calendar. Argentine driver Juan Manuel Fangio triumphed in the inaugural race. However, it wasn’t until 1955 that the second Formula One Monaco Grand Prix took place. Since then, this event has been a constant in the racing calendar.
What’s Next After Monte Carlo?
Following the thrill of the Monte Carlo Grand Prix, Formula One teams will have a week to gear up for the next Grand Prix at the Circuit de Barcelona-Catalunya. This follows their recent participation in the Grand Prix in Italy-Imola.
Formula 1 GP Monte Carlo – Winners
Year | Racer | Team |
---|
2023 | Max Verstappen | Red Bull |
2022 | Sergio Perez | Red Bull |
2021 | Max Verstappen | Red Bull |
2020 | no race because of Covid-19 | |
2019 | Lewis Hamilton (GBR) | Mercedes |
2018 | Daniel Ricciardo (AUS) | Red Bull |
2017 | Sebastian Vettel (D) | Ferrari |
2016 | Lewis Hamilton (GBR) | Mercedes |
2015 | Nico Rosberg (GER) | Mercedes |
2014 | Nico Rosberg (GER) | Mercedes |
2013 | Nico Rosberg (GER) | Mercedes |
2012 | Mark Webber (AUS) | Red Bull Racing |
2011 | Sebastian Vettel (GER) | Red Bull Racing |
2010 | Mark Webber (AUS) | Red Bull Racing |
2009 | Jenson Button (GBR) | Brawn |
2008 | Lewis Hamilton (GBR) | McLaren |
2007 | Fernando Alonso (ESP) | McLaren |
2006 | Fernando Alonso (ESP) | Renault |
2005 | Kimi Räikkönen (FIN) | McLaren |
2004 | Jarno Trulli (ITA) | Renault |
2003 | Juan Pablo Montoya (COL) | Williams |
2002 | David Coulthard (GBR) | McLaren |
2001 | Michael Schumacher (GER) | Ferrari |
2000 | David Coulthard (GBR) | McLaren |
1999 | Michael Schumacher (GER) | Ferrari |
1998 | Mika Häkkinen (FIN) | McLaren |
1997 | Michael Schumacher (GER) | Ferrari |
1996 | Olivier Panis (FRA) | Ligier |
1995 | Michael Schumacher (GER) | Benetton |
1994 | Michael Schumacher (GER) | Benetton |
1993 | Ayrton Senna (BRA) | McLaren |
1992 | Ayrton Senna (BRA) | McLaren |
1991 | Ayrton Senna (BRA) | McLaren |
1990 | Ayrton Senna (BRA) | McLaren |
1989 | Ayrton Senna (BRA) | McLaren |
1988 | Alain Prost (FRA) | McLaren |
1987 | Ayrton Senna (BRA) | Lotus |
1986 | Alain Prost (FRA) | McLaren |
1985 | Alain Prost (FRA) | McLaren |
1984 | Alain Prost (FRA) | McLaren |
1983 | Keke Rosberg (FIN) | Williams |
1982 | Riccardo Patrese (ITA) | Brabham |
1981 | Gilles Villeneuve (CAN) | Ferrari |
1980 | Carlos Reutemann (ARG) | Williams |
1979 | Jody Scheckter (RSA) | Ferrari |
1978 | Patrick Depailler (FRA) | Tyrrell |
1977 | Jody Scheckter (RSA) | Wolf |
1976 | Niki Lauda (AUT) | Ferrari |
1975 | Niki Lauda (AUT) | Ferrari |
1974 | Ronnie Peterson (SWE) | Lotus |
1973 | Jackie Stewart (GBR) | Tyrrell |
1972 | Jean-Pierre Beltoise (FRA) | BRM |
1971 | Jackie Stewart (GBR) | Tyrrell |
1970 | Jochen Rindt (AUT) | Lotus |
1969 | Graham Hill (GBR) | Lotus |
1968 | Graham Hill (GBR) | Lotus |
1967 | Denny Hulme (NZL) | Brabham |
1966 | Jackie Stewart (GBR) | BRM |
1965 | Graham Hill (GBR) | BRM |
1964 | Graham Hill (GBR) | BRM |
1963 | Graham Hill (GBR) | BRM |
1962 | Bruce McLaren (AUS) | Cooper |
1961 | Stirling Moss (GBR) | Lotus |
1960 | Stirling Moss (GBR) | Lotus |
1959 | Jack Brabham (AUS) | Cooper |
1958 | Maurice Trintignant (FRA) | Cooper |
1957 | Juan Manuel Fangio (ARG) | Maserati |
1956 | Stirling Moss (GBR) | Maserati |
1955 | Maurice Trintignant (FRA) | Ferrari |
1950 | Juan Manuel Fangio (ARG) | Alfa Romeo |
Is Free Viewing of Formula One Races Possible?
GP Monte Carlo: Exciting news for Formula 1 enthusiasts! There’s a savvy way to watch all Formula 1 races without shelling out for a costly TV subscription. This involves leveraging the power of a VPN service, a method I’ve been personally using for years with great success. Not only is this approach cost-effective, but it also ensures top-notch broadcast quality. So, for those passionate about Formula 1 and eager to catch every race live, this VPN method is definitely worth exploring.
The post F1 GP Monte Carlo – Circuit de Monaco appeared first on xplatform.rocks.
Die the native web GmbH veranstaltet ab kommender Woche insgesamt zwölf Webinare zu den Themen Go, TypeScript, skalierbare APIs und moderne, native Web-UIs.
For those facing the challenge of migrating their .NET Framework-based application to the modern .NET stack, Microsoft’s “Upgrade Assistant” is highly recommended:
What is the “Upgrade Assistant”?
The “Upgrade Assistant” is a tool that can integrate into Visual Studio or be accessed via CLI.
If you install the extension for Visual Studio you will have a new option “Upgrade project” available in your Solution Explorer.
.NET Framework to “new” and more…
Its main use case is upgrading .NET Framework-based WPF, WinForms, class libraries, or web applications to the newest .NET version. Besides this, the tool offers some other migration paths as well, e.g. from UWP to WinUI 3.
You even can use the tool to migrate from an older .NET Core version to a newer version (but - to be honest: those upgrades are quite easy in contrast to the .NET Framework to .NET Core migration).
Depending on the project type, the assistant allows for an “In-Place Upgrade,” “Side-by-Side,” or “Side-by-Side Incremental” upgrade.
- “In-Place Upgrade” means that the existing code is directly modified.
- “Side-by-Side” means that a new project is created and migration to the latest version is based on a copy.
- “Side-by-Side Incremental,” to my knowledge, is only available for ASP.NET web applications. Here, a new .NET Core project is added in parallel, and a sort of “bridge” is built in the original .NET project. This seems to me to be clever on the one hand but also very risky on the other.
You can see those upgrade methods in the video above.
Is it good?
We have used (or at least tested) the Assistant for upgrading WPF and class libraries to .NET Core and it helps to identify problems (e.g. if a NuGet package or any “old” framework code is not compatible).
My verdict: If you need to upgrade your code, you should give it a try.
In a more complex code base, it will sometimes mess with the code, but it still helps to give directions.
Hope this helps!
Mark your calendars for an exhilarating motorsport event! The Grand Prix of Spain is set to rev up from June 2 to June 4, 2023, in Barcelona. This highly anticipated race follows the iconic Monaco Grand Prix and precedes the Canadian Grand Prix in Montreal. Motorsport enthusiasts are in for a treat as the Formula One circuit continues its global journey.
Spotlight on the Circuit de Barcelona-Catalunya
Nestled in the heart of Montmeló, just north of Barcelona, the Circuit de Barcelona-Catalunya stands as a testament to Spain’s love for motorsports. The circuit’s inception was driven by the desire to bring the Spain Grand Prix back to Barcelona. Since its grand opening in September 1991, this circuit has proudly hosted the Spain Grand Prix.
Beyond hosting prestigious races, the Circuit de Barcelona-Catalunya serves as a key testing ground for Formula 1 and motorbike teams. The region’s mild winter and spring temperatures make it an ideal location for pre-season training. Over the years, the circuit has undergone several modifications, featuring long, sweeping corners that challenge tyres and favour cars with superior aerodynamics.
One of the circuit’s most unforgettable moments was in 1996, when Michael Schumacher clinched his first victory for Scuderia Ferrari amidst a torrential downpour. In 2013, the circuit embraced its current name, Circuit de Barcelona-Catalunya, following a sponsorship agreement with the city of Barcelona.
Exploring the Intricacies of the Circuit
Renowned for its challenging layout, the Circuit de Barcelona-Catalunya boasts a total length of 4.655 kilometers, featuring 16 turns with a mix of 9 right and 7 left turns. The Spain Grand Prix here is a test of endurance and skill, with a race length of 307.362 km, spread over 66 laps.
One of the circuit’s most notable features is turn 10, also known as La Caixa, positioned on the picturesque Montjuic hill. This turn is particularly challenging for drivers, coming right after a long straight where speeds can soar up to 300 km/h.
The circuit isn’t just a haven for Formula 1; it also plays host to the Motorcycle Grand Prix (MotoGP) annually. This event draws a diverse crowd of motorsport aficionados and motorbike enthusiasts from around the globe, all eager to witness high-speed action and create lasting memories at this iconic circuit.
The best of the Barcelona GP
Year | Winner | Team / Racing car |
---|
2023 | M. Verstappen | Red Bull / Honda |
2022 | M. Verstappen | Red Bull / Honda |
2021 | L. Hamilton | Mercedes-AMG / Mercedes |
2020 | L. Hamilton | Mercedes-AMG / Mercedes |
2019 | L. Hamilton | Mercedes-AMG / Mercedes |
2018 | L. Hamilton | Mercedes-AMG / Mercedes |
2017 | L. Hamilton | Mercedes-AMG / Mercedes |
2016 | M. Verstappen | Red Bull / TAG Heuer |
2015 | N. Rosberg | Mercedes-AMG / Mercedes |
2014 | L. Hamilton | Mercedes-AMG / Mercedes |
2013 | F. Alonso | Ferrari / Ferrari |
2012 | P. Maldonado | Williams / Renault |
2011 | S. Vettel | Red Bull / Renault |
2010 | M. Webber | Red Bull / Renault |
2009 | J. Button | Brawn GP / Mercedes |
2008 | K. Räikkönen | Ferrari / Ferrari |
2007 | F. Massa | Ferrari / Ferrari |
2006 | F. Alonso | Renault / Renault |
2005 | K. Räikkönen | McLaren / Mercedes |
2004 | M. Schumacher | Ferrari / Ferrari |
2003 | M. Schumacher | Ferrari / Ferrari |
2002 | M. Schumacher | Ferrari / Ferrari |
2001 | M. Schumacher | Ferrari / Ferrari |
2000 | M. Häkkinen | McLaren / Mercedes |
1999 | M. Häkkinen | McLaren / Mercedes |
1998 | M. Häkkinen | McLaren / Mercedes |
1997 | J. Villeneuve | Williams / Renault |
1996 | M. Schumacher | Ferrari / Ferrari |
1995 | M. Schumacher | Benetton / Renault |
1994 | D. Hill | Williams / Renault |
1993 | A. Prost | Williams / Renault |
1992 | N. Mansell | Williams / Renault |
1991 | N. Mansell | Williams / Renault |
Barcelona’s Charm: Beyond the Track
Barcelona, the pulsating heart of Catalonia in Spain, is renowned globally for its breathtaking art and architecture. This city offers much more than just the thrill of the Spain Grand Prix.
Must-See Attractions in Barcelona Beyond the Grand Prix
While in Barcelona, there are several must-visit attractions that add to the charm of this vibrant city:
- Explore the Masterpieces of Gaudí: Marvel at the architectural genius of Antoni Gaudí, particularly the iconic Sagrada Família church.
- Wander Through the Barri Gòtic: Immerse yourself in the historic ambiance of the Gothic Quarter.
- Stroll Down Rambla Street: Experience the lively atmosphere of this famous street.
- Unwind at Barceloneta Beach: Relax on the sands of this popular urban beach.
- Indulge at Mercat de la Boqueria: Savor the flavors at this bustling public market.
Delve into Barcelona’s Culinary Delights
Barcelona’s culinary scene is a hidden gem, often found in the most unassuming places. The city’s best eateries often dazzle with their simplicity, opting for modest decor over grandeur. It’s in these low-key establishments, often with wooden or plastic chairs, where you’ll find the most authentic and delicious meals.
These cozy spots, frequently run by local families, serve traditional dishes like tapas, offering a genuine taste of Barcelona. Here, you’ll mingle with locals and experience the city’s culinary heart. So, venture into these lesser-known eateries – the experience is truly rewarding.
How to Watch the F1 Spain Grand Prix for Free
For fans unable to make it to Barcelona, there’s good news. You can watch all the Formula 1 races, including the Spain Grand Prix, from the comfort of your home for free. All you need is a reliable VPN service and stable internet. This way, you won’t miss a moment of the high-speed action, no matter where you are.
The post F1 in Barcelona – Spain Grand Prix appeared first on xplatform.rocks.
This year, the much-anticipated Formula 1 Canadian Grand Prix is set to take place in Montreal from June 16 to 18. Prior to this event, Formula 1 teams competed at the Circuit de Barcelona-Catalunya in Spain for the Barcelona Grand Prix. Following the excitement in Canada, the next stop will be the Red Bull Ring in Spielberg, Austria.
Circuit Gilles Villeneuve: Montreal’s Pride in F1 History
The Formula One Canadian Grand Prix has a rich history dating back to its inaugural race in 1967 at Circuit Mont-Tremblant, Quebec. This first race saw the victory of the legendary Jack Brabham, a two-time world champion at that time. Over the years, the event moved between various locations before settling at its current home, the Circuit Gilles Villeneuve in Montreal, in 1978.
Named in memory of Gilles Villeneuve, a Canadian racing icon who sadly passed away in a 1982 Belgian Grand Prix accident, the Circuit Gilles Villeneuve has been synonymous with the Canadian Grand Prix since then. Known for its high-speed stretches, tight turns, and demanding layout, the circuit tests the mettle of the world’s best drivers.
One of the most notable moments in the circuit’s history was in 1994, when Michael Schumacher, who would go on to become a record-holding world champion, clinched his first Formula 1 victory here. Schumacher’s affinity with this track is evident as he won the Canadian Grand Prix seven times, marking his extraordinary career.
The Canadian Grand Prix faced cancellations in 2020 and 2021 due to the global Corona pandemic.
Exploring the Gilles Villeneuve Circuit
Situated on Île Notre-Dame in Montreal, the Circuit Gilles-Villeneuve is a temporary motor racing track named on June 12, 1982, in honor of Gilles Villeneuve. The circuit, set on the man-made Île Notre-Dame in the St. Lawrence River, doubles as a public road when not hosting races.
Featuring long straights and fast sections due to its location on the elongated island, the course spans 4.361 km and is raced in a clockwise direction. Over 70 laps, drivers cover a total distance of 305.270 km.
Since its establishment in 1978, the circuit has seen minimal changes. Major alterations include moving the start-finish line and pit lane in 1987 to the end of the Droit du Casino straight, rather than behind the Épingle des Stands hairpin. In 2002, the pit exit was modified, now leading into the Virage de Senna hairpin instead of the first corner.
Champions of the Canadian Grand Prix
Over the years, the Canadian Grand Prix has celebrated numerous winners, each leaving their mark on this historic circuit.
Year | Winner |
---|
2022 | Max Verstappen |
2021 | No race |
2020 | No race |
2019 | Lewis Hamilton (Mercedes) |
2018 | Sebastian Vettel (Ferrari) |
2017 | Lewis Hamilton (Mercedes) |
2016 | Lewis Hamilton (Mercedes) |
2015 | Lewis Hamilton (Mercedes) |
2014 | Daniel Ricciardo (Red Bull-Renault) |
2013 | Sebastian Vettel (Red Bull-Renault) |
2012 | Lewis Hamilton (McLaren-Mercedes) |
2011 | Jenson Button (McLaren-Mercedes) |
2010 | Lewis Hamilton (McLaren-Mercedes) |
2009 | No race |
2008 | Robert Kubica (BMW Sauber) |
2007 | Lewis Hamilton (McLaren-Mercedes) |
2006 | Fernando Alonso (Renault) |
2005 | Kimi Räikkönen (McLaren-Mercedes) |
2004 | Michael Schumacher (Ferrari) |
2003 | Michael Schumacher (Ferrari) |
2002 | Michael Schumacher (Ferrari) |
2001 | Ralf Schumacher (Williams-BMW) |
2000 | Michael Schumacher (Ferrari) |
1999 | Mika Häkkinen (McLaren-Mercedes) |
1998 | Michael Schumacher (Ferrari) |
1997 | Michael Schumacher (Ferrari) |
1996 | Damon Hill (Williams-Renault) |
1995 | Jean Alesi (Ferrari) |
1994 | Michael Schumacher (Benetton-Ford Cosworth) |
1993 | Alain Prost (Williams-Renault) |
1992 | Gerhard Berger (McLaren-Honda) |
1991 | Nelson Piquet (Benetton-Ford Cosworth) |
1990 | Ayrton Senna (McLaren-Honda) |
1989 | Thierry Boutsen (Williams-Renault) |
1988 | Ayrton Senna (McLaren-Honda) |
1987 | No race |
1986 | Nigel Mansell (Williams-Honda) |
1985 | Michele Alboreto (Ferrari) |
1984 | Nelson Piquet (Brabham-BMW) |
1983 | René Arnoux (Ferrari) |
1982 | Nelson Piquet (Brabham-BMW) |
1981 | Jacques Laffite (Ligier-Matra) |
1980 | Alan Jones (Williams-Ford Cosworth) |
1979 | Alan Jones (Williams-Ford Cosworth) |
1978 | Gilles Villeneuve (Ferrari) |
1977 | Jody Scheckter (McLaren-Ford Cosworth) |
1976 | James Hunt (McLaren-Ford Cosworth) |
1975 | No race |
1974 | Emerson Fittipaldi (McLaren-Ford Cosworth) |
1973 | Peter Revson (McLaren-Ford Cosworth) |
1972 | Jackie Stewart (Tyrrell-Ford Cosworth) |
1971 | Jackie Stewart (Tyrrell-Ford Cosworth) |
1970 | Jacky Ickx (Ferrari) |
1969 | Jacky Ickx (Brabham-Ford Cosworth) |
1968 | Denis Hulme (McLaren-Ford Cosworth) |
1967 | Jack Brabham (Brabham-Repco) |
Stellar Performances at the Gilles-Villeneuve Circuit
The Canadian Grand Prix at Montreal’s Gilles-Villeneuve Circuit has witnessed some remarkable achievements over the years, particularly from two of Formula 1’s most celebrated drivers.
When it comes to securing pole positions at the Canadian Grand Prix, Michael Schumacher from Germany and Lewis Hamilton from the United Kingdom have both demonstrated exceptional prowess. Each has claimed the pole position six times, showcasing their skill and consistency on this challenging track.
In terms of victories, Schumacher and Hamilton again stand out, each having emerged as champions seven times at the Montreal GP. This remarkable feat places them jointly at the top for the most wins in the history of the Canadian Grand Prix, reflecting their dominance in the sport.
The circuit’s current lap record is held by the Finnish racing talent, Valtteri Bottas. In 2019, driving a Mercedes, Bottas delivered an extraordinary performance, clocking a lap time of 1:13.078.
However, the fastest lap ever recorded at the Gilles Villeneuve Circuit belongs to Germany’s Sebastian Vettel. During the qualifying round in 2019, Vettel pushed his Ferrari to the limits, setting an unparalleled record time of 1:10.240.
Access to Formula 1: No Pay-TV Required?
For fans seeking to watch Formula 1 races, there’s a convenient and cost-effective way to enjoy all the action from home. Without the need for a pay-TV subscription, you can access live streams of Formula 1 races. This method, which I’ve personally been using for years, offers excellent broadcast quality. It’s a great option for enthusiasts who want to stay up-to-date with all the thrilling races.
The post The F1 Canadian Grand Prix – GP Montreal appeared first on xplatform.rocks.
In the thrilling world of Formula 1, the British Grand Prix is slated to be held at its birthplace – the Silverstone Circuit – from July 7 to 9 this year. This race follows closely on the heels of the Austrian Grand Prix at Spielberg, which takes place a week earlier. Post-Silverstone, the action moves to the Hungaroring in Budapest.
Silverstone: A Circuit Steeped in History
The Silverstone Grand Prix stands as one of the most iconic and storied events in motorsport history. Tracing its origins back to the nascent days of Formula One, the first British Grand Prix was hosted in 1950 at this very circuit, then a repurposed military airfield, and marked the inception of the Formula One World Championship.
Over the years, the Silverstone circuit has evolved from its original, lengthier airstrip format to a more compact, safer, and exhilarating 5.891 km track. This transformation has made Silverstone a benchmark for modern racing circuits.
Silverstone Today: The Pinnacle of the F1 Season
Now, the Silverstone Circuit is renowned as a highlight of the Formula 1 calendar. The current configuration offers a 52-lap race, totaling 306.3 kilometers, where high-tech cars zoom past an audience of up to 135,000 spectators. Max Verstappen holds the record for the fastest lap in this circuit with a time of 1:27.097 in his Red Bull racer.
The Legacy of Silverstone’s Grand Prix
The Silverstone Grand Prix has consistently delivered riveting races and dramatic moments for decades. Racing legends like Jim Clark,
Jackie Stewart, Nigel Mansell, and Lewis Hamilton have etched their names in its history with multiple victories, reinforcing the British legacy in racing.
In 2010, the circuit underwent significant renovations to adapt to the advanced needs of modern Formula One racing. This upgrade included redesigning the pit areas, the starting and finishing straights, and various corners.
The Grand Prix at Silverstone has maintained its essential place in the Formula One roster for over seventy years. In 2020, its 70th anniversary was celebrated with a special race, aptly named the “70th Anniversary Grand Prix,” to compensate for the numerous cancellations caused by the COVID-19 pandemic. Interestingly, Silverstone, alongside Monza, has the distinction of being one of the only two F1 circuits to have consistently hosted races since 1950.
Champions of the Silverstone Grand Prix
The history of the British Grand Prix at Silverstone is marked by an illustrious list of winners from various teams, showcasing the competitive spirit and evolution of the sport over the years.
Year | Pilot | Team |
---|
2023 | Max Verstappen | Red Bull |
2022 | Carlos Sainz jr. | Ferrari |
2021 | Lewis Hamilton | Mercedes |
2020 | Max Verstappen | Red Bull |
2020 | Lewis Hamilton | Mercedes |
2019 | Lewis Hamilton | Mercedes |
2018 | Sebastian Vettel | Ferrari |
2017 | Lewis Hamilton | Mercedes |
2016 | Lewis Hamilton | Mercedes |
2015 | Lewis Hamilton | Mercedes |
2014 | Lewis Hamilton | Mercedes |
2013 | Nico Rosberg | Mercedes |
2012 | Mark Webber | Red Bull Renault |
2011 | Fernando Alonso | Ferrari |
2010 | Mark Webber | Red Bull Renault |
2009 | Sebastian Vettel | Red Bull Renault |
2008 | Lewis Hamilton | Mercedes |
2007 | Kimi Räikkönen | Ferrari |
2006 | Fernando Alonso | Renault |
2005 | Juan Pablo Montoya | McLaren Mercedes |
2004 | Michael Schumacher | Ferrari |
2003 | Rubens Barrichello | Ferrari |
2002 | Michael Schumacher | Ferrari |
2001 | Mika Häkkinen | McLaren Mercedes |
2000 | David Coulthard | McLaren Mercedes |
1999 | David Coulthard | McLaren Mercedes |
1998 | Michael Schumacher | Ferrari |
1997 | Jacques Villeneuve | Williams Renault |
1996 | Jacques Villeneuve | Williams Renault |
1995 | Johnny Herbert | Benetton Renault |
1994 | Damon Hill | Williams Renault |
1993 | Alain Prost | Williams Renault |
1992 | Nigel Mansell | Williams Renault |
1991 | Nigel Mansell | Williams Renault |
1990 | Alain Prost | Ferrari |
1989 | Alain Prost | McLaren Honda |
1988 | Ayrton Senna | McLaren Honda |
1987 | Nigel Mansell | Williams Honda |
1985 | Alain Prost | McLaren Porsche |
1983 | Alain Prost | Renault |
1981 | John Watson | McLaren Ford |
1979 | Clay Regazzoni | Williams Ford |
1977 | James Hunt | McLaren Ford |
1975 | Emerson Fittipaldi | McLaren Ford |
1973 | Peter Revson | McLaren Ford |
1971 | Jackie Stewart | Tyrrell Ford |
1969 | Jackie Stewart | Matra Ford |
1967 | Jim Clark | Lotus Climax |
1965 | Jim Clark | Lotus Climax |
1963 | Jim Clark | Lotus Climax |
1960 | Jack Brabham | Cooper Climax |
1958 | Peter Collins | Ferrari |
1956 | Juan Manuel Fangio | Ferrari |
1954 | José Froilán González | Ferrari |
1953 | Alberto Ascari | Ferrari |
1952 | Alberto Ascari | Ferrari |
1951 | José Froilán González | Ferrari |
1950 | Giuseppe Farina | Alfa Romeo |
Accessing Formula 1 Excitement Live and Free
For fans wanting to experience the entire Formula 1 season, there are options to watch every race live for free using VPN services. This method, which I have personally used for several years, ensures you don’t miss a single moment of racing action due to transmission issues, all while saving money.
The post F1 British Grand Prix at Silverstone appeared first on xplatform.rocks.
The Austrian Grand Prix, set amidst the picturesque Red Bull Ring in Spielberg, is a thrilling event for Formula 1 fans. Scheduled to take place from June 30 to July 2, it follows two weeks after the Canadian Grand Prix in Montreal. This event promises an adrenaline-packed weekend, leading up to another racing highlight – the British Grand Prix at Silverstone Circuit.
The Legacy and Evolution of the Spielberg Grand Prix/Austrian GP
The journey of Formula One in Spielberg began in 1970, with races being a staple at the Österreichring, nestled in the landscapes of Spielberg and Flatschach. Originally spanning 5.911 kilometers, the track underwent an extension to 5.942 kilometers in 1977, a response to the tragic accident involving Mark Donohue. Throughout its early years, the race lengths varied, oscillating between 52 and 54 laps, a slight decrease from the initial 60 laps in its inaugural season.
Known for its blistering speeds, the Österreichring frequently witnessed new records in average lap speeds, earning a reputation as a high-speed battleground for racers.
The 1987 race marked a turning point, with two severe accidents prompting a decade-long pause for Formula One in Austria, as the track no longer met modern standards. The 1990s saw a transformative redesign of the circuit, enhancing safety, introducing runoff zones, and narrowing corners. This modernization reduced the track’s length to 4.319 kilometers and ushered in a new era under the name A1-Ring, with Formula One returning from 1997 to 2003.
In 2004, Dietrich Mateschitz acquired the site, transforming it into a state-of-the-art motorsports center, now known as the Red Bull Ring. This marked a new chapter in the track’s history.
By 2014, Formula One made a triumphant return to Spielberg, with the first race of this new era held on June 22. Further solidifying its place in the F1 calendar, a contract extension in March 2023 announced races until at least the 2027 season.
The Modern Marvel: Red Bull Ring’s Design and Experience
Hermann Tilke, a renowned track architect, was tasked with designing the contemporary Red Bull Ring. A unique aspect of this track is its striking altitude variations, offering clear visibility and a distinct racing experience.
Spanning 4.318 kilometers for Formula 1 races, the Red Bull Ring features ten turns and an elevation of 677 meters, creating an exhilarating drive. It boasts a 65-meter elevation change, with steep gradients adding to the challenge.
The circuit starts with a demanding right turn, leading into a steep uphill straight. The hairpin, a prime spot for overtaking, slows cars from over 320 km/h to just 70 km/h. Following this is a downhill right turn, leading into quick left turns in the infield, culminating in the final bends.
Nestled in Upper Styria’s Aichfeld, the Red Bull Ring is cradled by slopes on three sides, some serving as natural grandstands. This not only provides spectators with panoramic views but also integrates the track seamlessly into the picturesque landscape, marrying the thrill of racing with the tranquility of nature.
Austrian GP – List of Winners
Year | Pilot | Team |
---|
2022 | Charles Leclerc | Ferrari |
2021 | Max Verstappen | Red Bull Honda |
2020 | Lewis Hamilton | Mercedes |
2020 | Valtteri Bottas | Mercedes |
2019 | Max Verstappen | Red Bull Honda |
2018 | Max Verstappen | Red Bull Renault |
2017 | Valtteri Bottas | Mercedes |
2016 | Lewis Hamilton | Mercedes |
2015 | Nico Rosberg | Mercedes |
2014 | Nico Rosberg | Mercedes |
2003 | Michael Schumacher | Ferrari |
2002 | Michael Schumacher | Ferrari |
2001 | David Coulthard | McLaren Mercedes |
2000 | Mika Häkkinen | McLaren |
1999 | Eddie Irvine | Ferrari |
1998 | Mika Häkkinen | McLaren Mercedes |
1997 | Jacques Villeneuve | Williams |
1987 | Nigel Mansell | Williams Honda |
1986 | Alain Prost | McLaren Porsche |
1985 | Alain Prost | McLaren Porsche |
1984 | Niki Lauda | McLaren Porsche |
1983 | Alain Prost | Renault Renault |
1982 | Elio de Angelis | Lotus Ford |
1981 | Jacques Laffite | Ligier Matra |
1980 | Jean-Pierre Jabouille | Renault |
1979 | Alan Jones | Williams Ford |
1978 | Ronnie Peterson | Lotus Ford |
1977 | Alan Jones | Shadow Ford |
1976 | John Watson | Penske Ford |
1975 | Vittorio Brambilla | March Ford |
1974 | Carlos Reutemann | Brabham Ford |
1973 | Ronnie Peterson | Lotus Ford |
1972 | Emerson Fittipaldi | Lotus Ford |
1971 | Joseph Siffert | B.R.M. |
1970 | Jacky Ickx | Ferrari |
Enjoy Every Race from Home: Free and Easy Streaming
Are you an F1 enthusiast looking for a way to catch all the action without the hefty subscription fees? Great news: You can watch every F1 race live, and it’s completely free. No subscriptions, no hidden costs. If this sounds too good to be true, I encourage you to read our detailed guide. It’s a method I’ve personally used for years, saving substantial amounts of money while enjoying seamless, high-quality broadcasts. Don’t miss out on this fantastic opportunity to stay connected with every thrilling moment of the races, all from the comfort of your home.
The post F1 Austria GP – Red Bull Ring in Spielberg appeared first on xplatform.rocks.
.NET 8.0 bietet zwei neue Objektmengen, bei denen man nach dem Erstellen die Elemente nicht ändern, ergänzen oder löschen kann.
In my last post “First steps with WinUI 3” I already mentioned the “WinUI 3 Gallery”-App, but I missed mentioning two great resources.
If you take a deeper look at the “Home” page, you will spot the Community Toolkit Gallery (another app) and the “Template Studio for WinUI”.
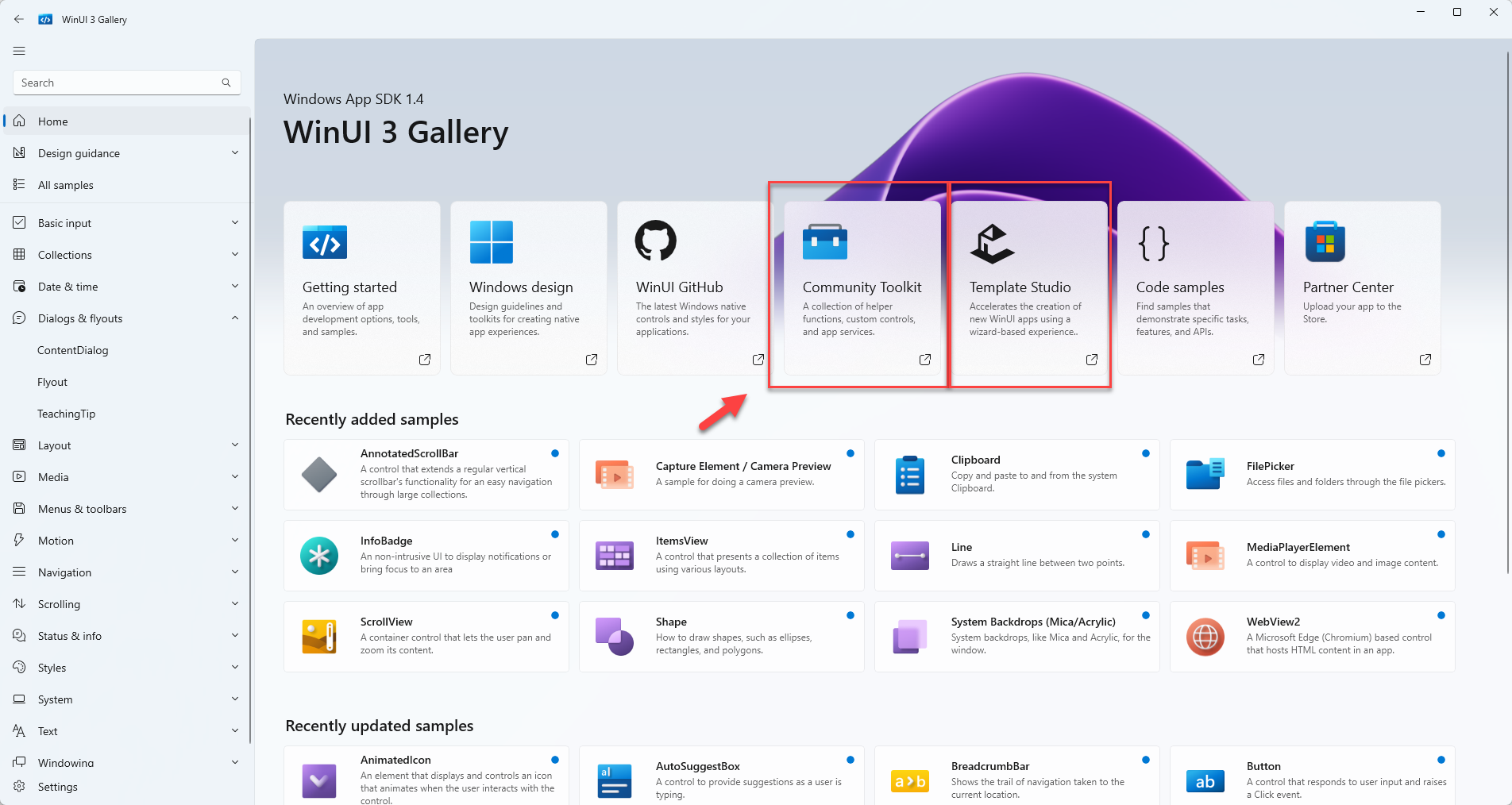
The Community Toolkit is a community-driven collection of components and other helpers.
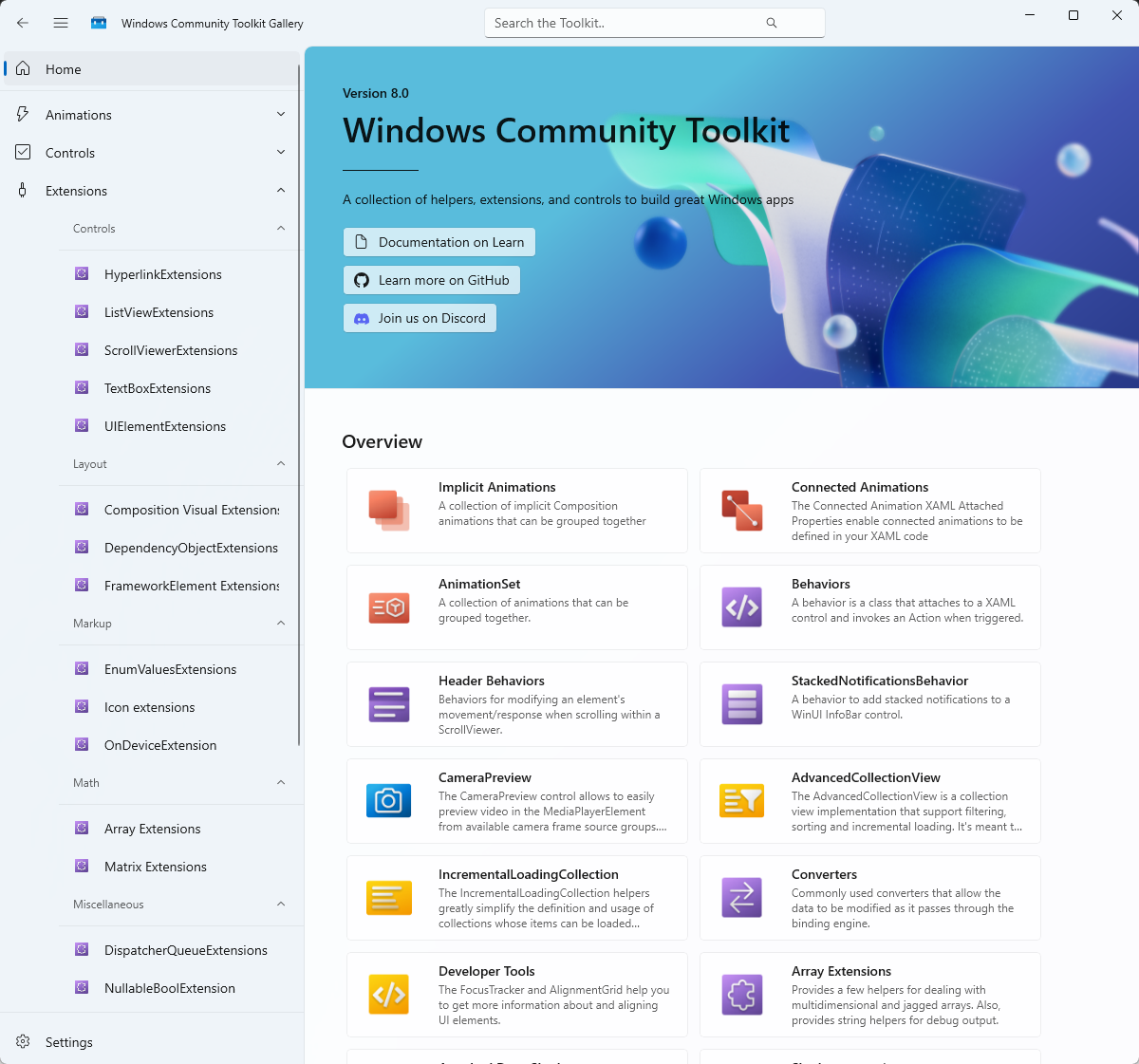
The “home” of the Community Toolkit can be found on GitHub
As of today, the Community Toolkit seems “alive” with recent commits in February 2024.
Interesting fact: The controls from the toolkit seems to work with the Uno Platform as well.
What is the Template Studio?
Template Studio is an addin for Visual Studio and can be installed from the Marketplace.
This adds the ‘Template Studio for WinUI’ template to Visual Studio:
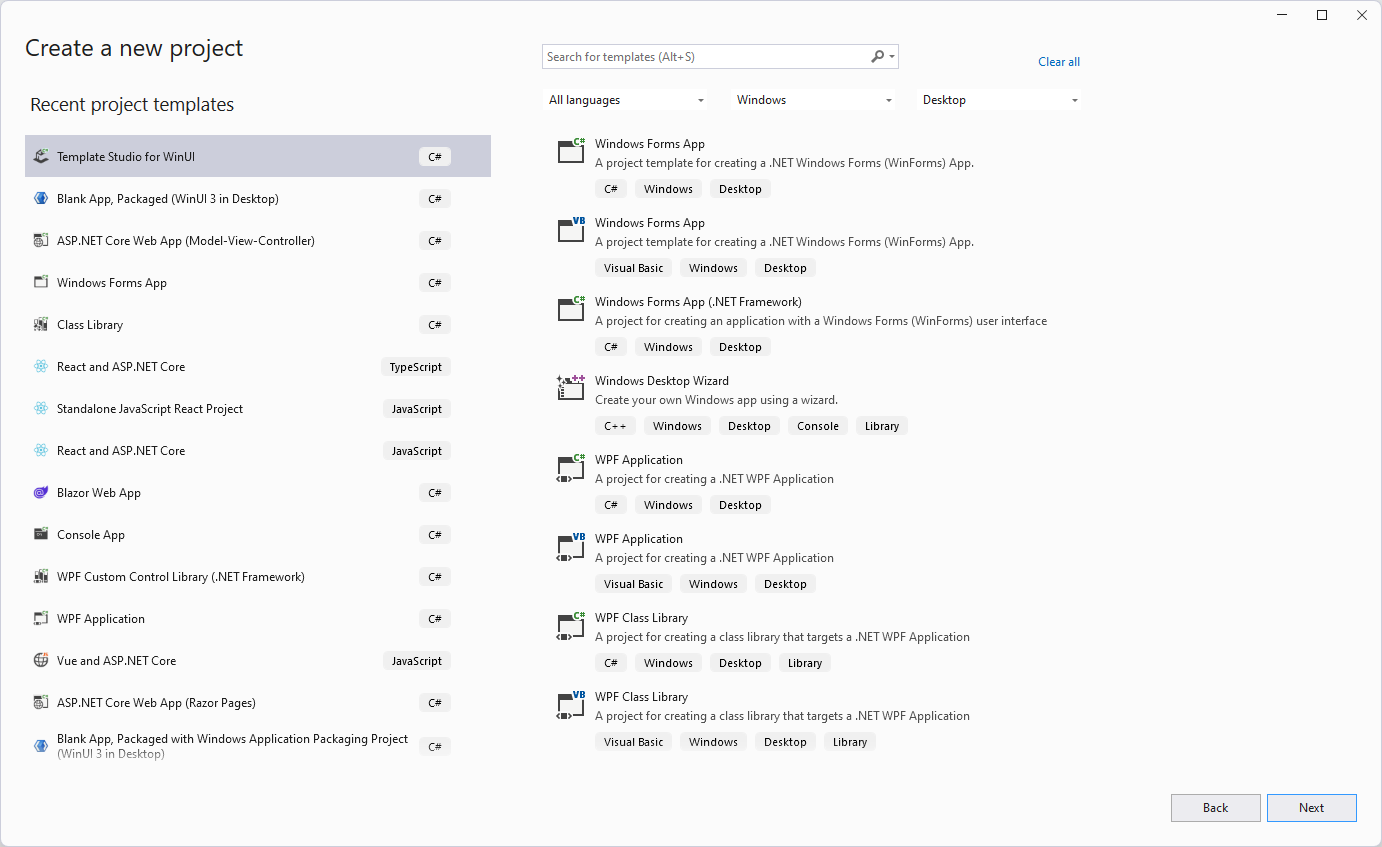
After the usual “pick a name and location” you will be greeted with this Wizard:
The first step is to select a “Project type”:
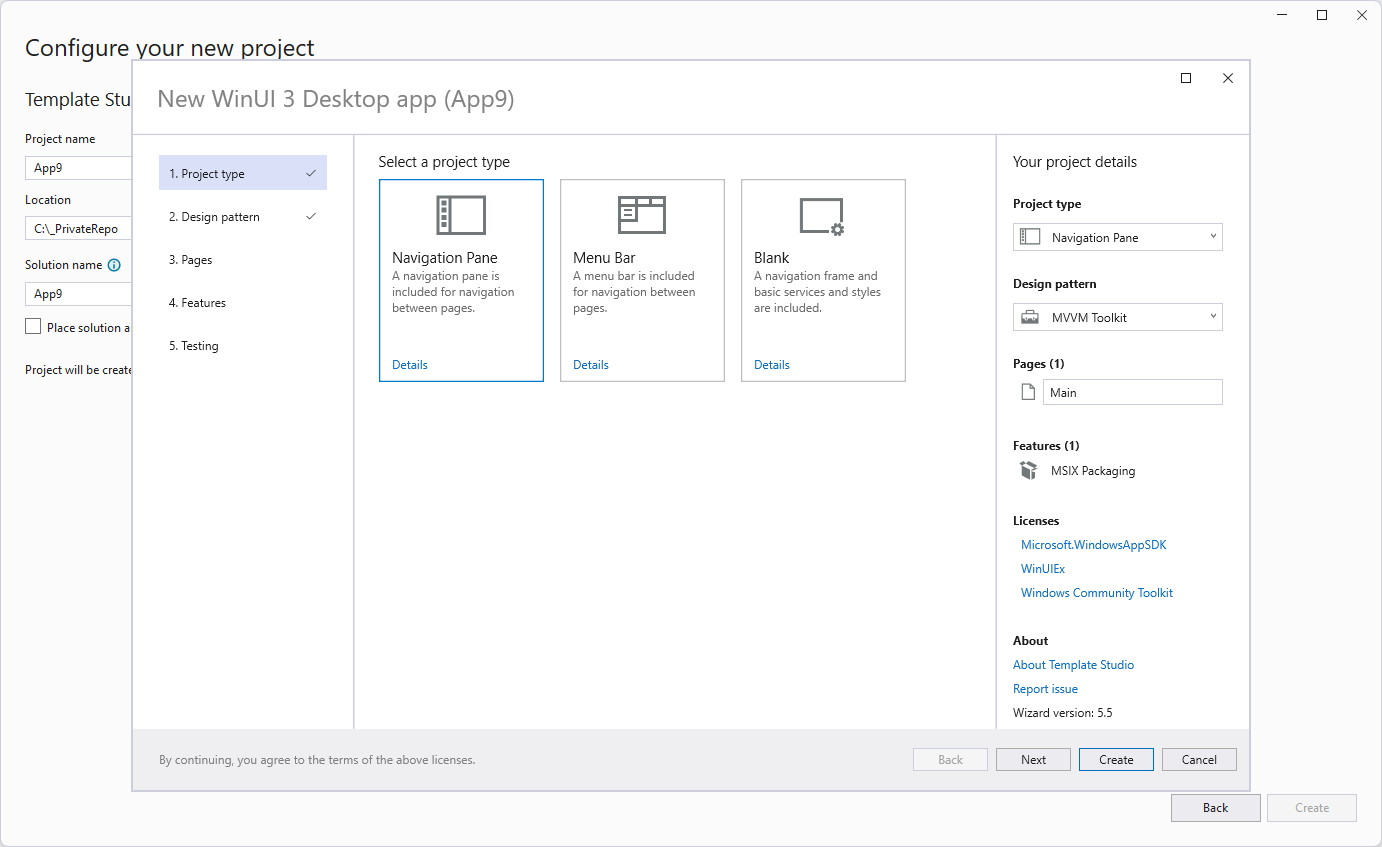
In the next step you choose a “Design pattern” - which has only one item… well.
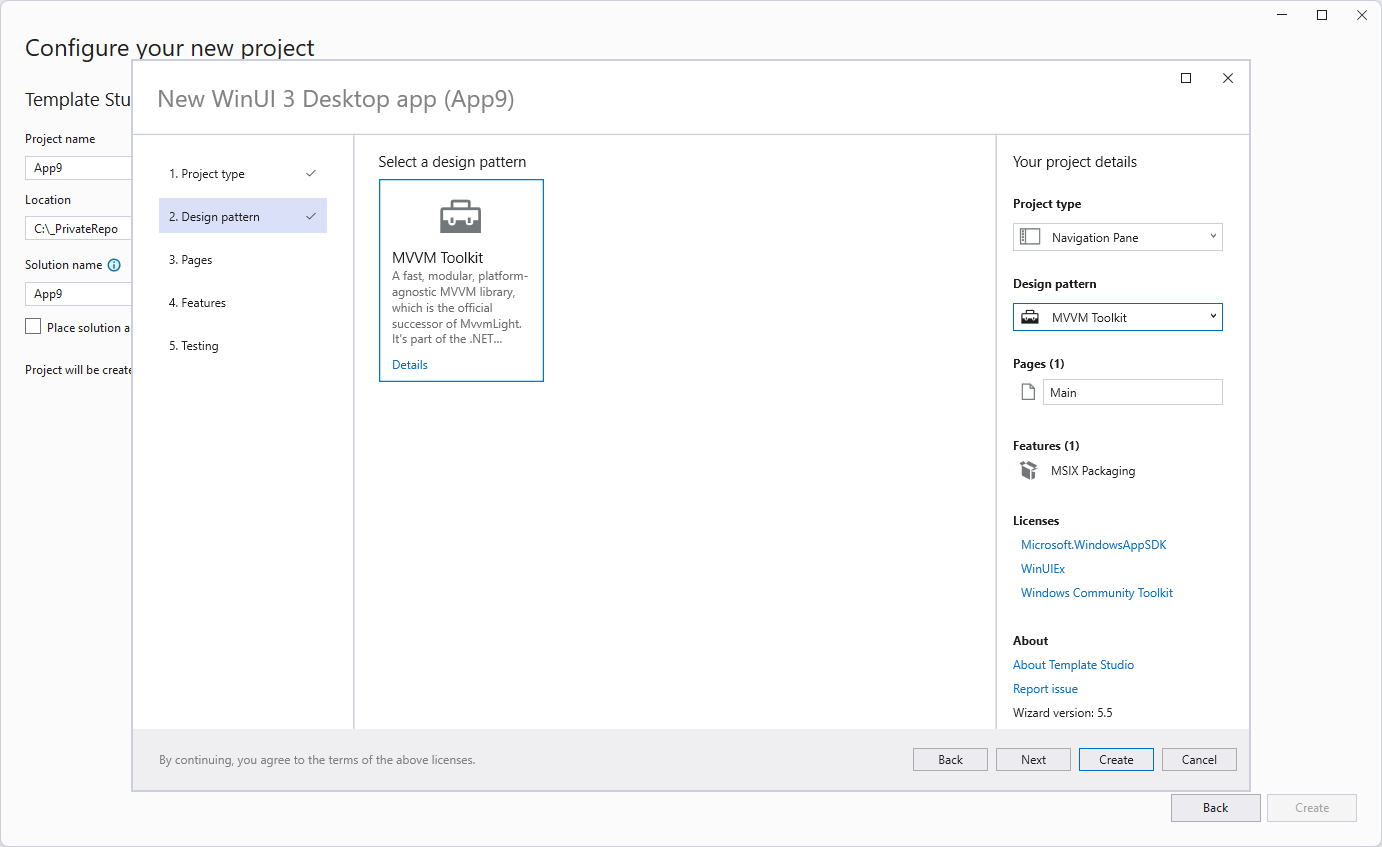
In “Pages” you can create your “views/pages” based on a given layout:
Some pages can only be added once (e.g. the “Settings”), but most pages can be added multiple times.
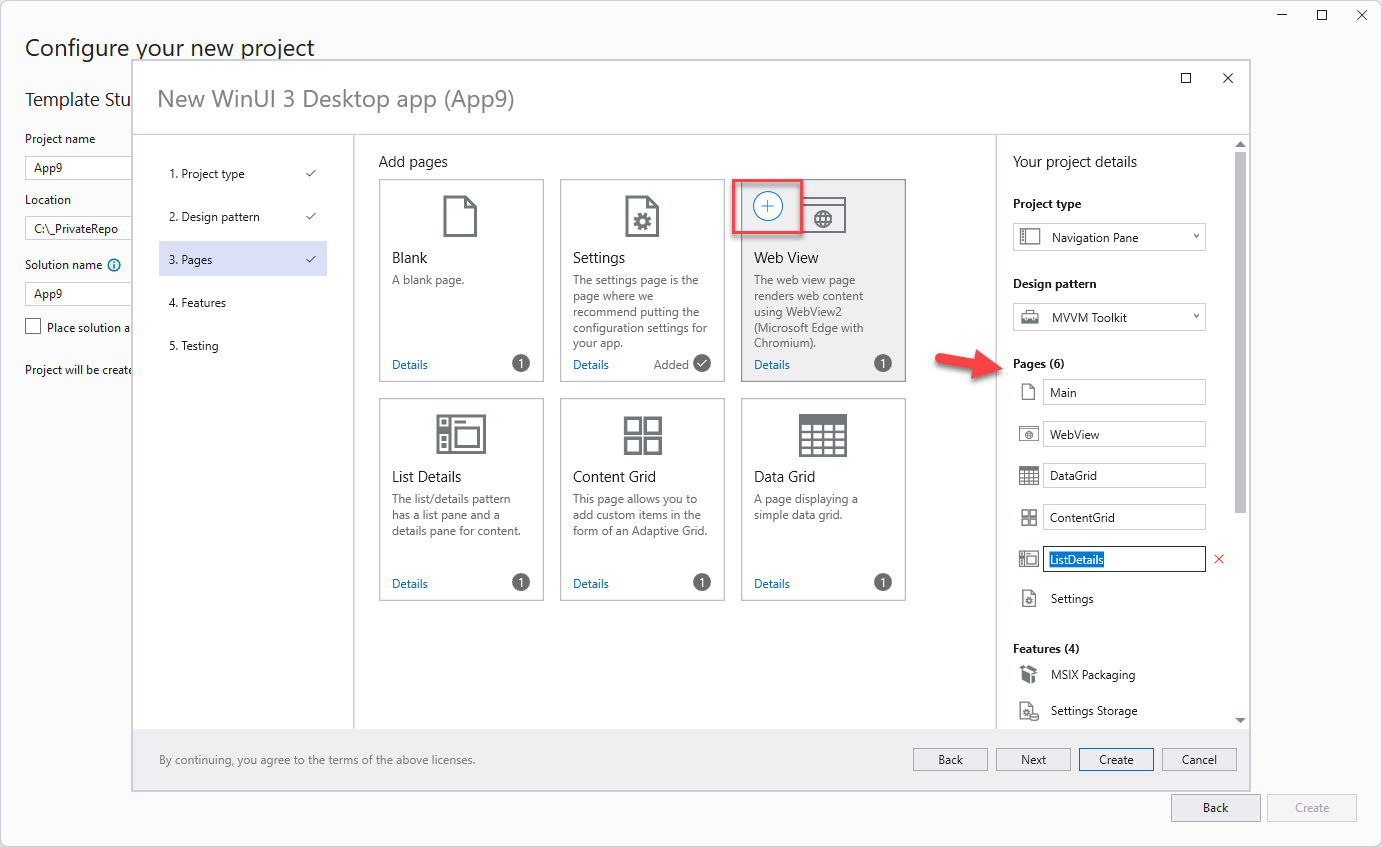
In “Features” you can add some WinUI 3 related features:
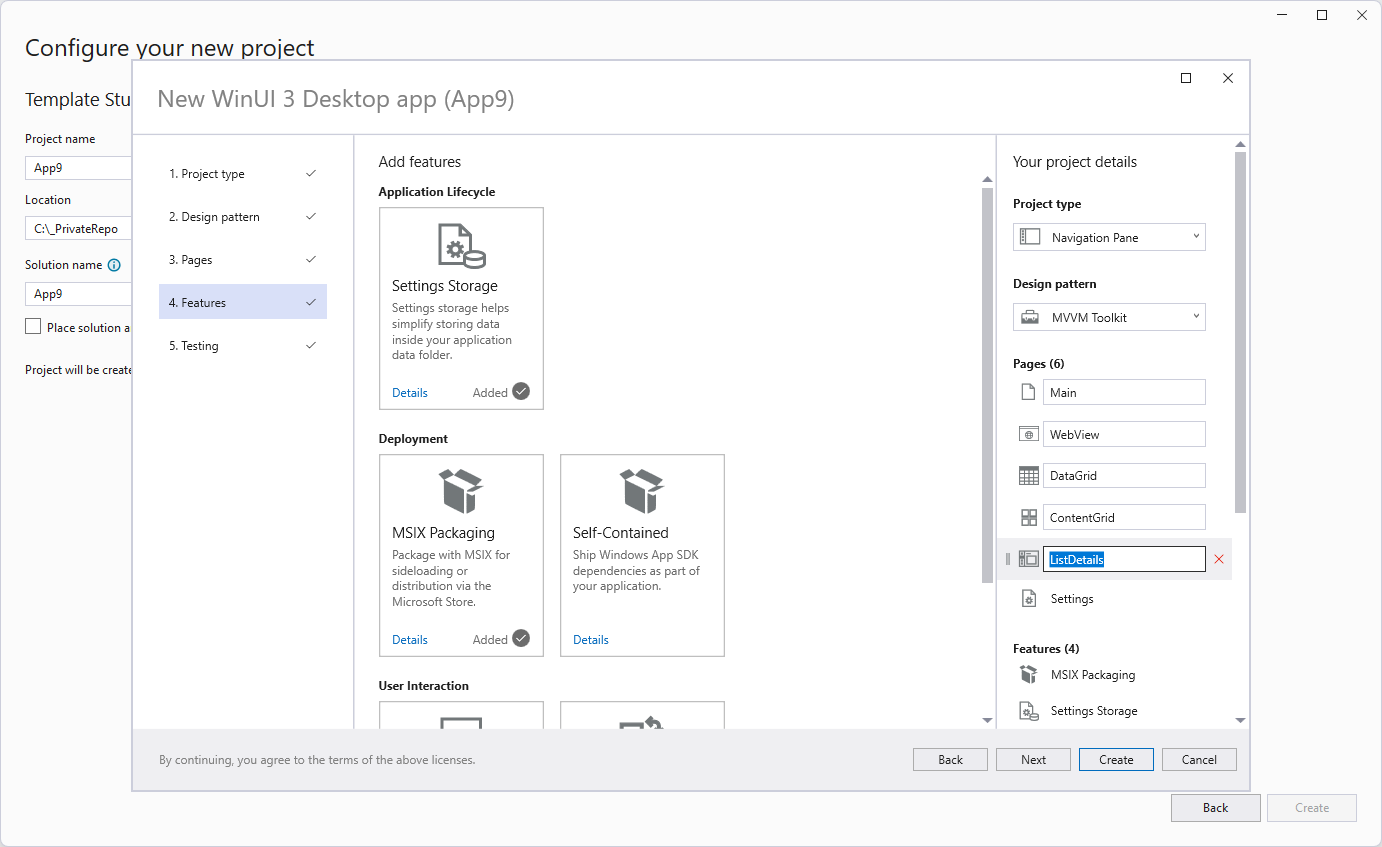
In the last setting you can decide if you want to add an MSTest project as well:
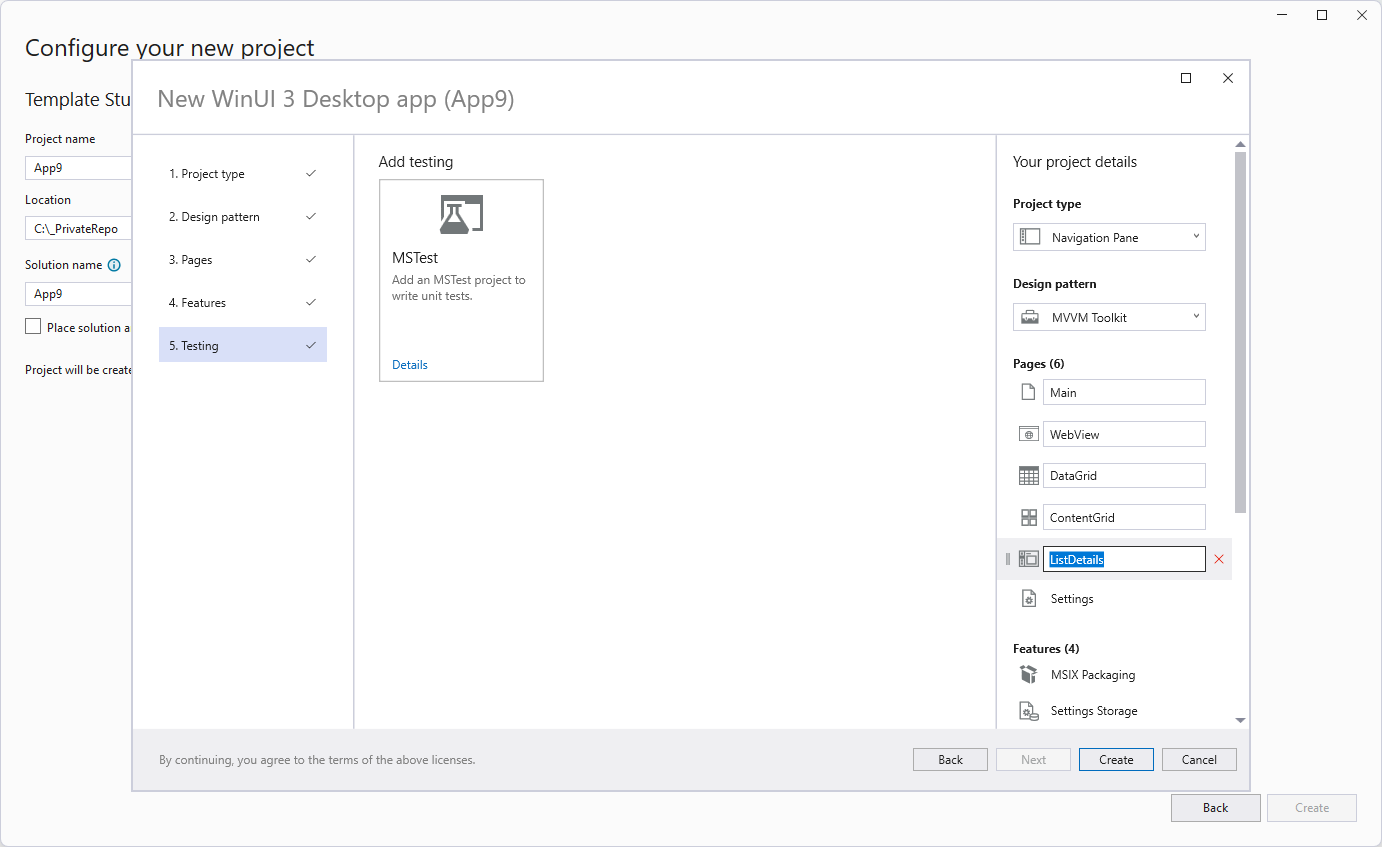
The result is the following Visual Studio solution, which includes two projects and a number of TODO
items:
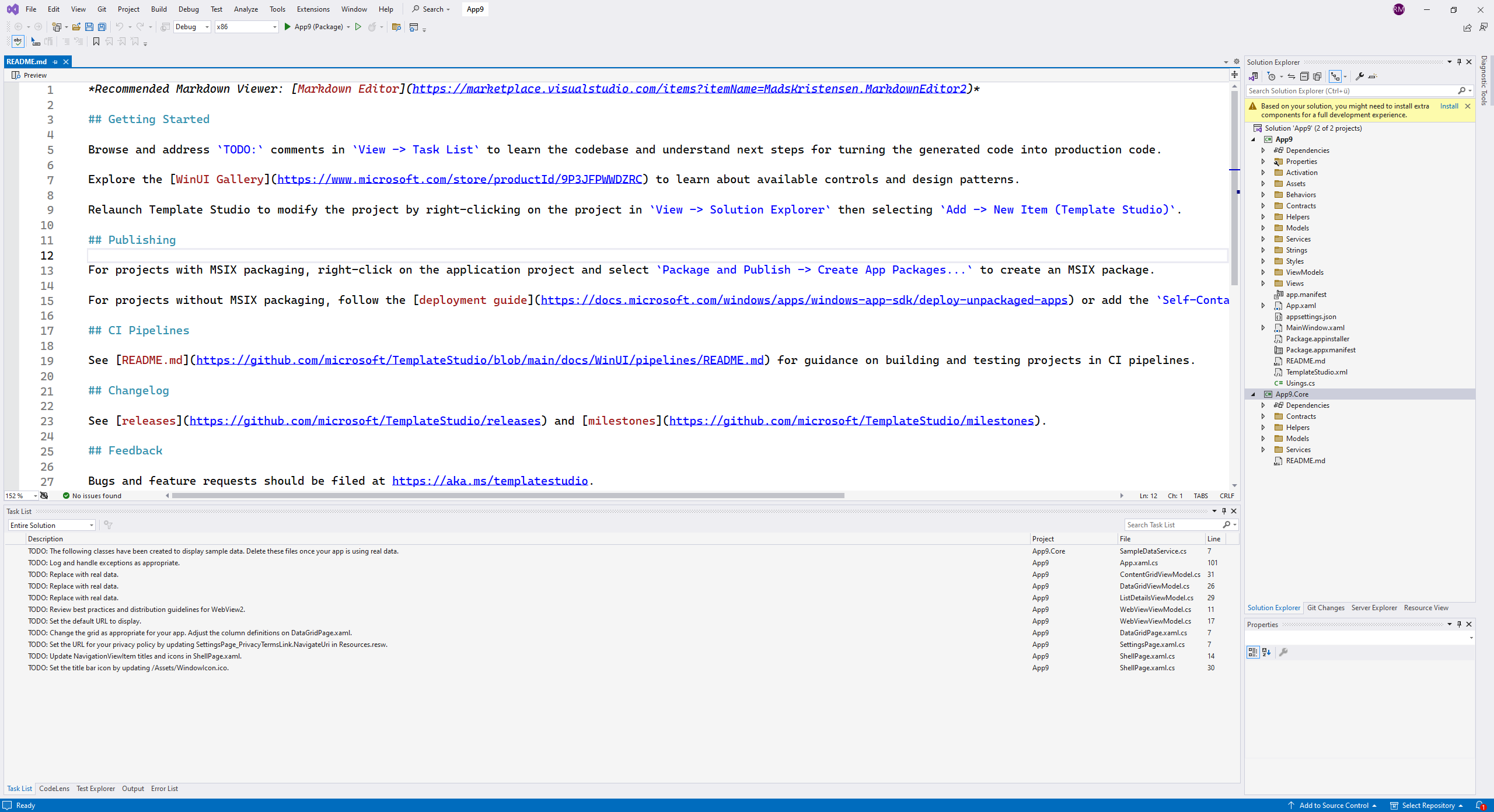
If you run the code a pretty simple app with your configured pages will be found:
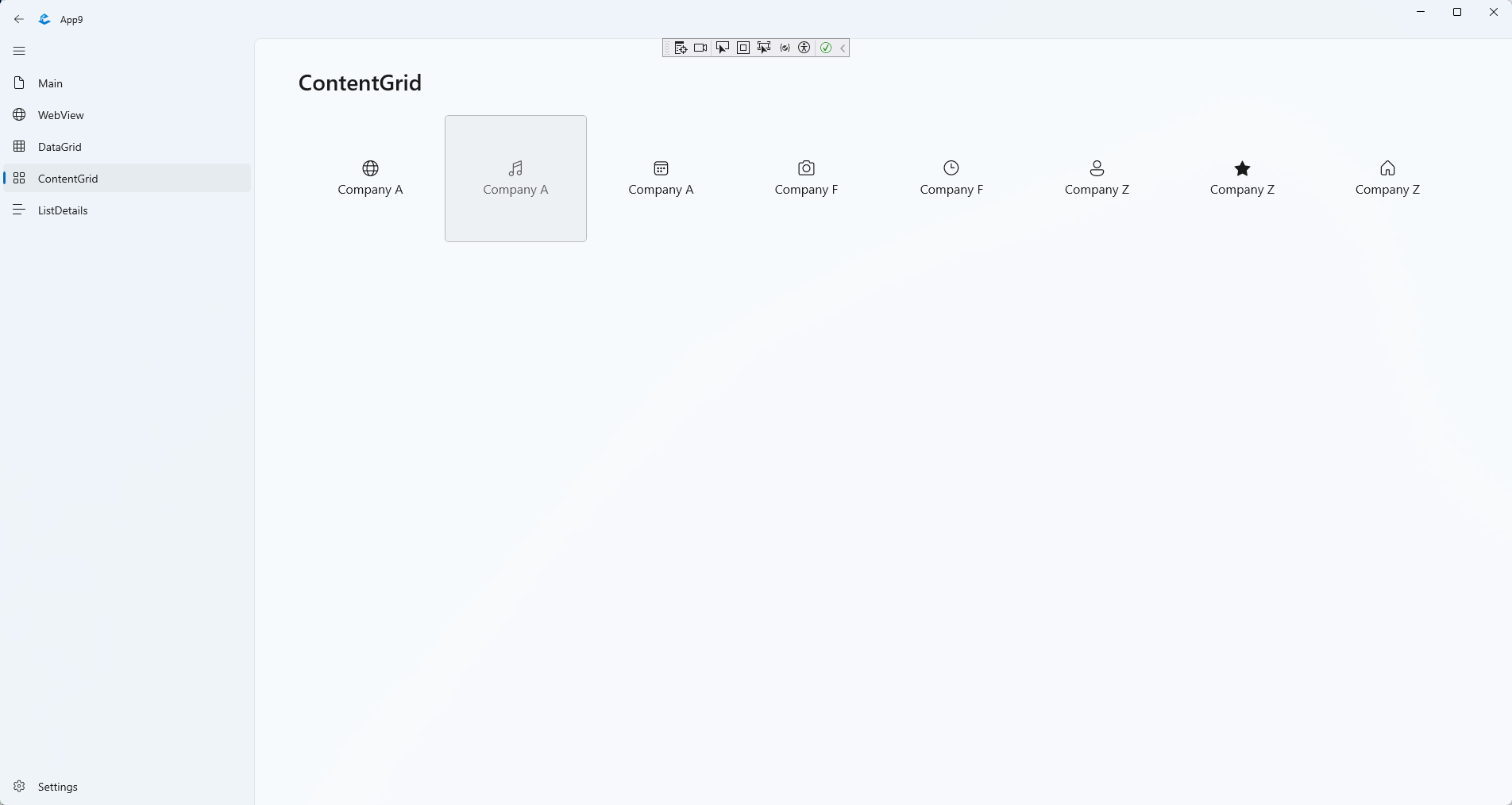
Warning: Such code generators might be a good starting point, but (as always with such generators) the code might be “too stupid” or “too complicated” - depending on your needs.
Any other useful resource?
I’m a newbie with WinUI 3. The Community Toolkit looks promising and even the Template Studio looks good - at least from a few minutes playtime. If anyone has other useful resource: Please let me know (e.g. in the comments or via email).
Hope this helps!
In der Klasse System.Random gibt es nun eine Zufallsreihenfolge mit Random.Shared.Shuffle() und Zufallsauswahl via Random.Shared.GetItems().
Die einen lieben HTMX, die anderen hassen es. Was ist dran am Hype um HTMX, was spricht für und was gegen die Bibliothek?
At the .NET Conf 2023, Microsoft announced a kind of toolset to build cloud-native applications. That announcement was kind of hidden in a talk done by Glenn Condron and David Fowler about building cloud-native applications using .NET 8, which was also announced at that conference. This talk actually was about .NET Aspire, which I will quickly introduce with this post.
Let's start first by answering a question.
When I did a talk about .NET Aspire recently at the .NET user group in Basel (CH), one individual in the audience asked me the following question:
What is a cloud-native application?
Let's ask the internet to find the right answer:
Amazon:
"Cloud native is the software approach of building, deploying, and managing modern applications in cloud computing environments. Modern companies want to build highly scalable, flexible, and resilient applications that they can update quickly to meet customer demands. To do so, they use modern tools and techniques that inherently support application development on cloud infrastructure. These cloud-native technologies support fast and frequent changes to applications without impacting service delivery, providing adopters with an innovative, competitive advantage."
(https://aws.amazon.com/what-is/cloud-native/)
Google:
"A cloud-native application is specifically designed from the ground up to take advantage of the elasticity and distributed nature of the cloud. "
(https://cloud.google.com/learn/what-is-cloud-native)
RedHat:
"Cloud-native applications are a collection of small, independent, and loosely coupled services."
(https://www.redhat.com/en/topics/cloud-native-apps)
Oracle:
"The term cloud native refers to the concept of building and running applications to take advantage of the distributed computing offered by the cloud delivery model. Cloud-native apps are designed and built to exploit the scale, elasticity, resiliency, and flexibility the cloud provides."
(https://www.oracle.com/cloud/cloud-native/what-is-cloud-native/)
Microsoft:
"Cloud-native architecture and technologies are an approach to designing, constructing, and operating workloads that are built in the cloud and take full advantage of the cloud computing model."
(https://learn.microsoft.com/en-us/dotnet/architecture/cloud-native/definition)
Cloud Native Computing Foundation (CNCF):
"Cloud native technologies empower organizations to build and run scalable applications in modern, dynamic environments such as public, private, and hybrid clouds. Containers, service meshes, microservices, immutable infrastructure, and declarative APIs exemplify this approach.
These techniques enable loosely coupled systems that are resilient, manageable, and observable. Combined with robust automation, they allow engineers to make high-impact changes frequently and predictably with minimal toil."
(https://github.com/cncf/toc/blob/main/DEFINITION.md)
Every answer is a little different. Basically, it means a cloud-native application is built for the cloud and uses the services the cloud provides to be scalable and resilient.
What is .NET Aspire doing?
.NET Aspire helps with tooling in VS and the CLI to create and interact with .NET Aspire apps. It also brings some project templates to create new .NET Aspire apps. .NET Aspire helps with orchestrating, means running and connecting to multi-project applications and their dependencies. It also provides components that connect to cloud dependencies like queues, caches, databases, or even prebuild containers. All those components can be orchestrated and connected to your applications using C#. .NET Aspire creates a deployment-ready development environment. Using the Azure Development CLI (azd) you can easily deploy your cloud native application to Azure.
.NET Aspire is made for local development and it is made for Microsoft Azure. Developments and deployments to other clouds might be possible in the future with the support of the developer community. In the first stage, Microsoft will not support other cloud providers. Which makes sense since Azure is the number one platform for Microsoft.
.NET Aspire uses Docker Desktop to run your cloud-native application. When you press F5 in VS, your apps will be deployed to containers and will run on Docker Desktop locally. When you deploy your cloud-native application, a Bycep script will be created and your apps will be deployed to a new Azure Resource Group inside Azure Container Apps. App Service Containers are not supported yet. AKS is only supported via the community tool Aspirate.
Currently, .NET Aspire is Preview 3. Which means some features might not work or are not yet implemented.
But those limitations are absolutely fine for the moment.
Why is .NET Aspire needed?
Actually, it is not needed. There are good tools out there to set up the local development environment the way you can develop cloud-native applications locally. There are also tools that set up your development environment inside the cloud to develop in the same environment where your application will live. This is great and super helpful. Unfortunately, these possibilities are sometimes hard to set up and some teams can't use it for some reason. The easiest way to set up an environment locally for me as a developer on Windows using .NET was to use Docker Compose or to load or emulate the services I needed locally or to be connected to the cloud environment all the time and to use the cloud services directly. Both options are not perfect.
So, you see that .NET Aspire is not needed. But it is super helpful for me as a C# developer.
Let's have a quick look at .NET Aspire in action
Therefore, I created a frontend app using the new Blazor Web App and a backend that provides me with the data via a Web API endpoint. Both apps are just the default templates with the weather data demos. I just did a small modification: Instead of generating the weather data in the front end, it now loads them from the API.
When doing right-click on one of the projects and select "Add", you will see two new entries in the context menu:
- ".NET Aspire Component..."
- ".NET Aspire Orchestration Support..."
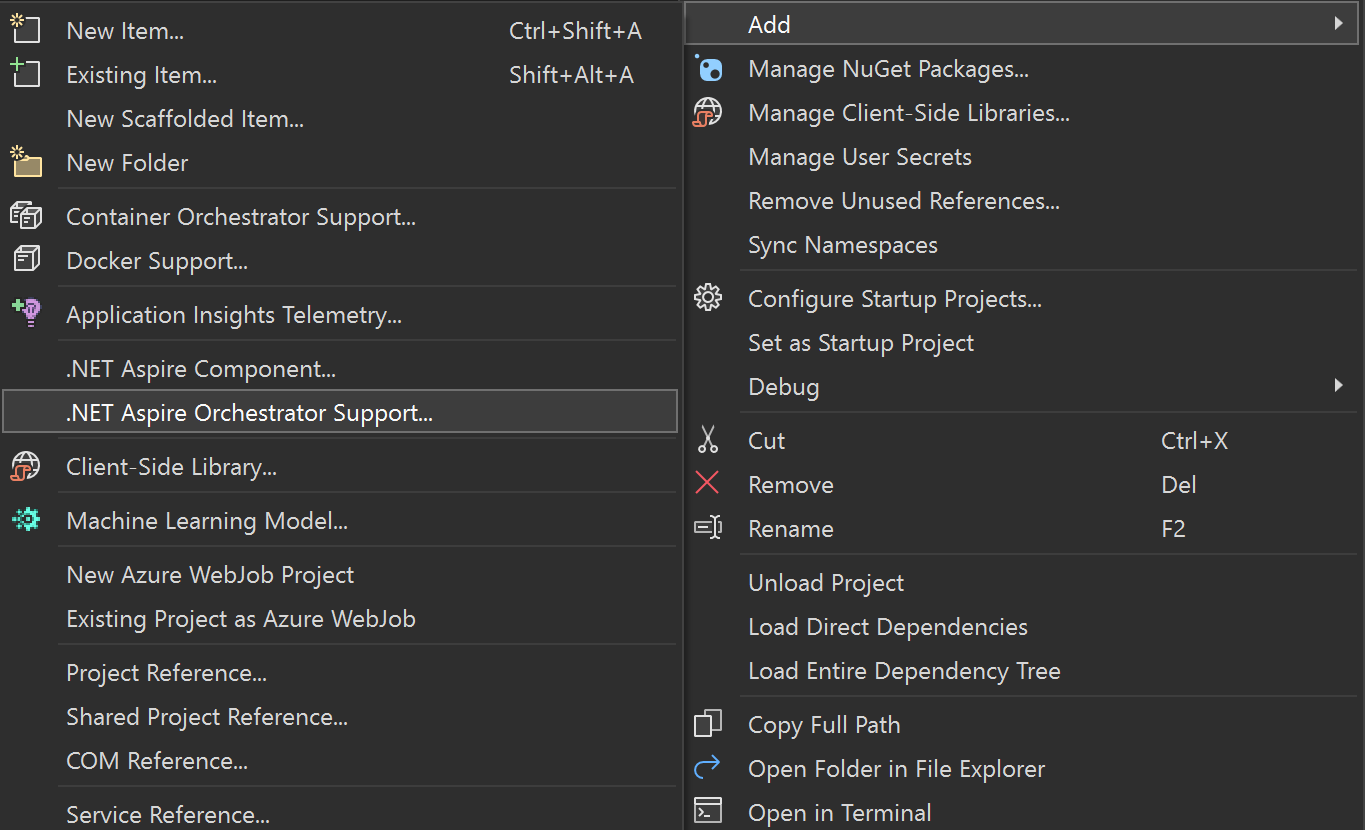
Selecting ".NET Aspire Orchestration Support...", it creates two new projects in your solution:
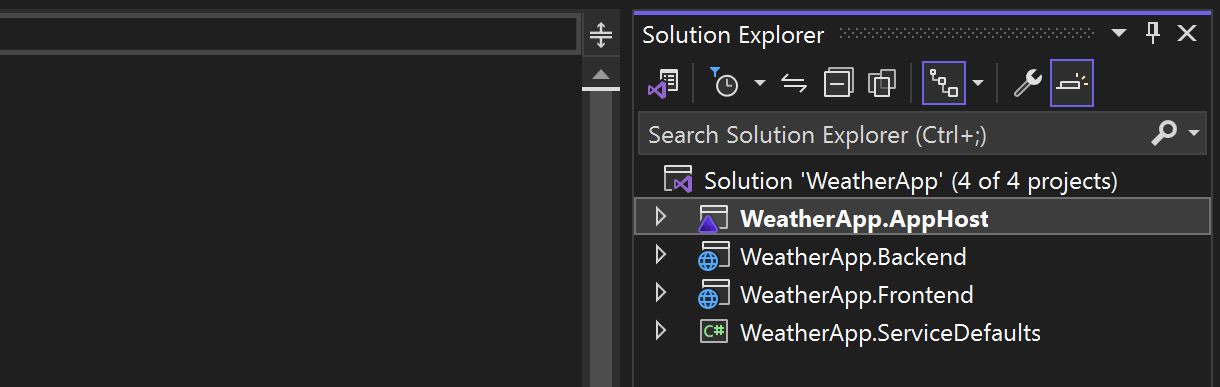
The AppHost is the project where you will do the actual composition, we will have a more detailed look later. The ServiceDefaults contains one single code file with extension methods that configure default services and Middlewares the actual projects need to use. Mainly Telemetry and HelthChecks. Actually, these service defaults are added to the actual projects when adding the Aspire Orchestration support. The following code shows the usage of the default in lines 5 and 17:
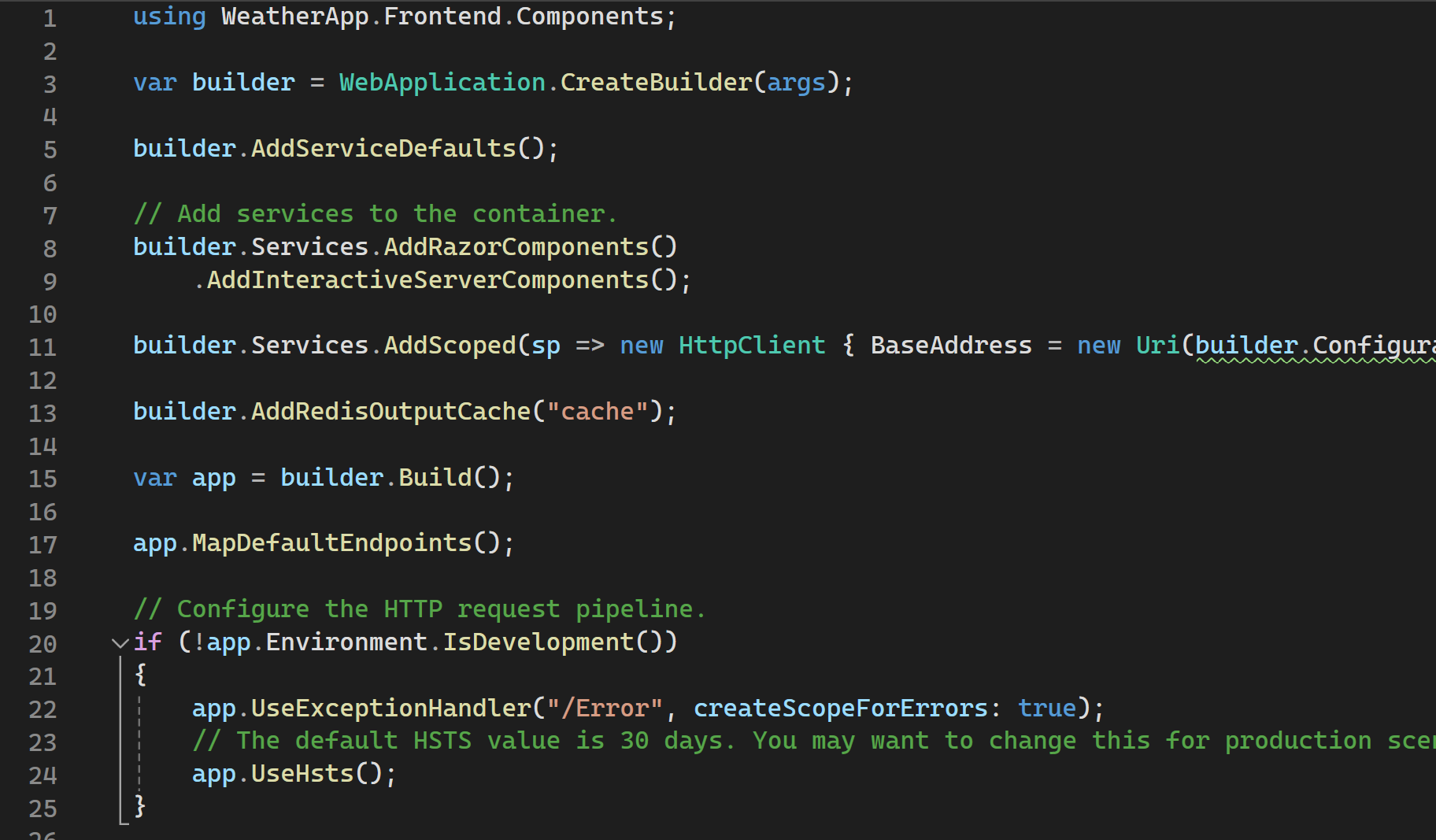
As you can see, I also configured a HttpClient that connects to the backend API.
I also added the Aspire orchestration support to the backend API and the service defaults are added to that project as well. In this project, I configured a distributed Redis cache in line 14:
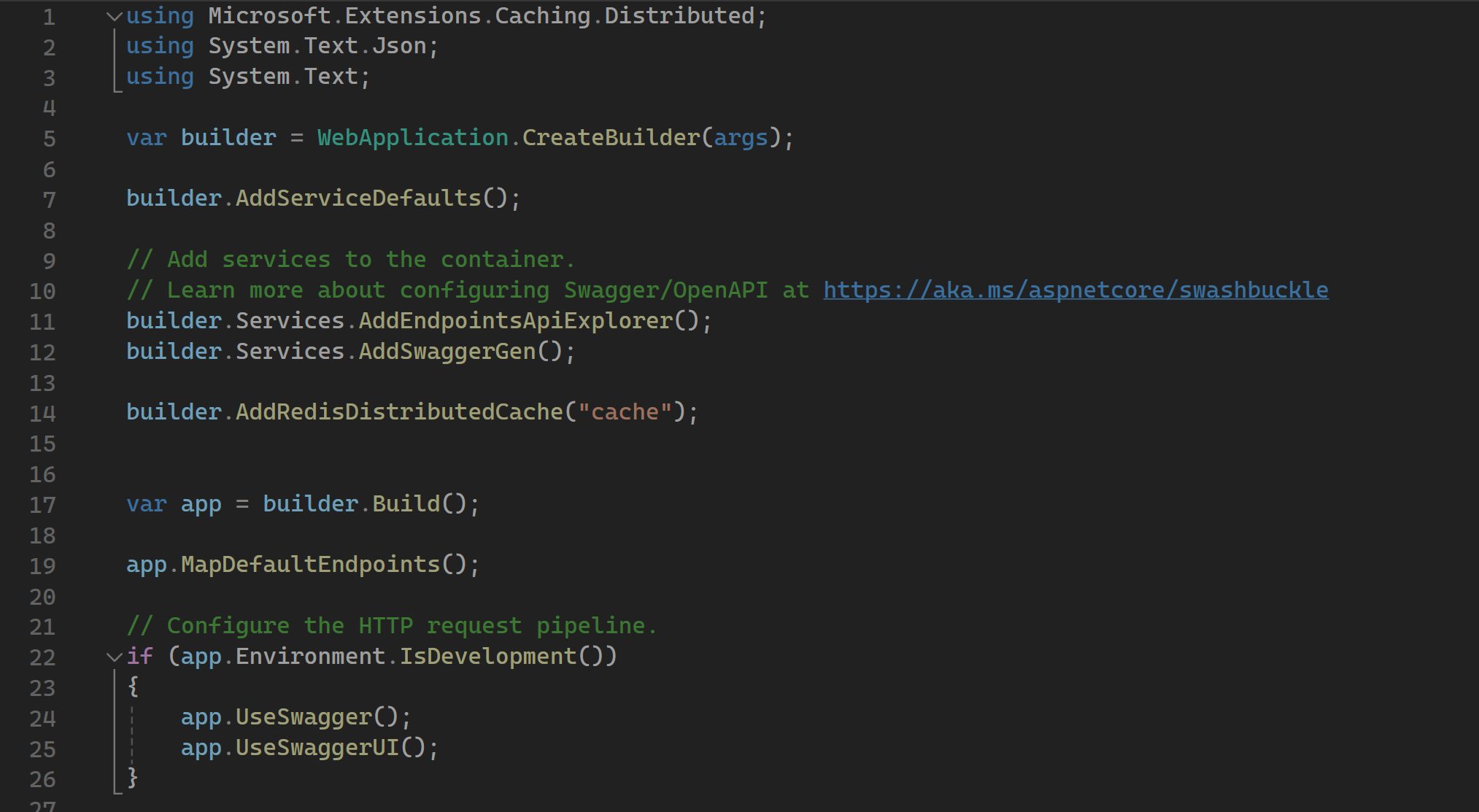
This application contains three components: A frontend which is a Blazor web app, a backend which is a minimal API and a Redis cache. These three components need to be orchestrated to run and debug it locally. The problem is, that I don't have a local instance of Redis yet.
This is where Aspire can help us. Let's have a look into the Program.cs
of the AppHost Project:
var builder = DistributedApplication.CreateBuilder(args);
var cache = builder.AddRedis("cache");
var backend = builder.AddProject<Projects.WeatherApp_Backend>("backend")
.WithReference(cache)
.WithReplicas(2);
builder.AddProject<Projects.WeatherApp_Frontend>("frontend")
.WithReference(backend);
builder.Build().Run();
This looks pretty similar to a regular minimal API without any ASP.NET Core stuff. The first line defines a DistributedApplicationBuilder which is the orchestrator.
Line 3 adds Redis to the orchestration with the name "cache". Remember that we configured the distributed cache with the exact same name in the backend project.
Line 5 adds a project reference to the orchestration with the name backend. It references the cache and it should start two instances of the backend.
Line 9 adds a project reference to the front end. This one needs the backend and adds it as a reference.
How does the frontend know the backend address when the apps are running in orchestration? I do have the same problem when I use docker-compose to orchestrate apps. In this case, i just need to read the endpoint URL from the environment variables:
IIbuilder.Services.AddScoped(sp => new HttpClient
{
BaseAddress = new Uri(builder.Configuration.GetValue<string>("services:backend:0"))
});
You will see why this is working a little later.
Let's start the application but ensure Docker Desktop is running first. Since it is all in preview at the moment, you may need to start the application two times. Once the app is started you'll see the URL in the console that pops up. In case no browser opens automatically copy the URL and open it in a browser.:
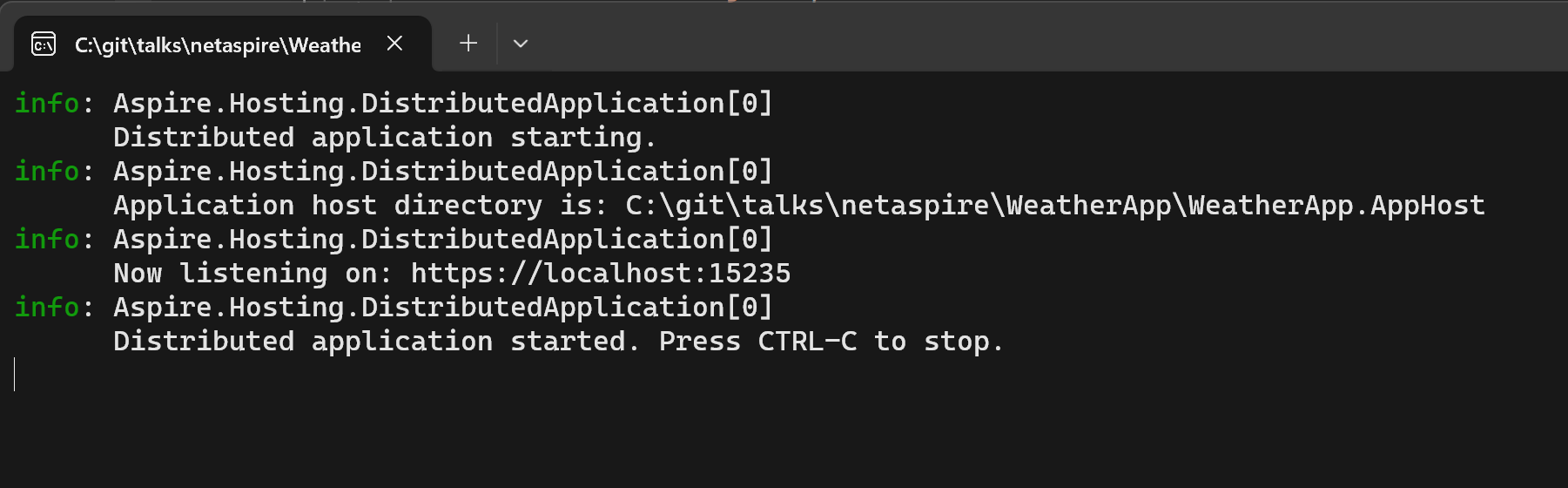
You will see the really cool Aspire portal in the browser that shows you all the running apps:
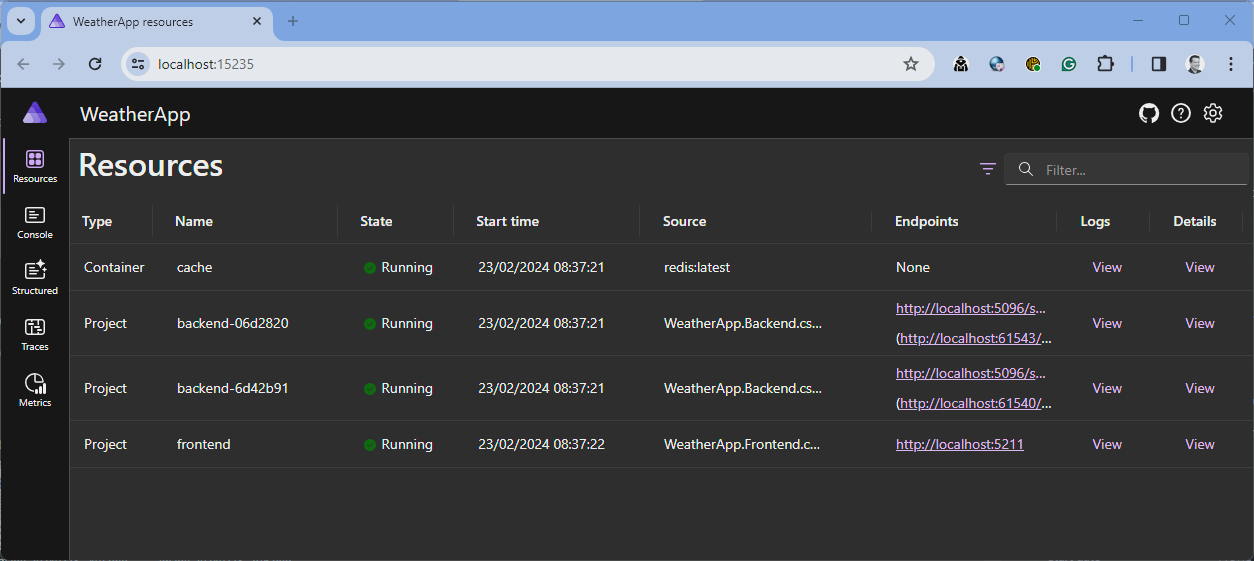
This Portal is built with the new ASP.NET Core Blazor web.
On the start screen, you see all the running services. Two instances of the backend app and one instance of the frontend app. You will also recognize the instance of the Redis cache. This is coming from a docker image that got pulled by Aspire and is running as a container now. You will also see that the backends have two endpoint URLs. One is equal to both instances and the other one is the individual URL for that specific container. The one that is equal to both is routed through a kid of a proxy.
This portal doesn't show you only the running services. Because of the Service defaults that got injected into the apps, it can read the health states, the logs, and the telemetry information of your apps. This will help you to debug your locally running apps. Just click through the portal to see the logs, the traces, and the metrics.
When you click on the details link of a specific running service, you can also see the environment variables that got passed to the service. In the next screenshot, you can see that the URL of the backend app will be passed as an environment variable to the frontend. This is the environment variable we used in the frontend to connect to the backend:
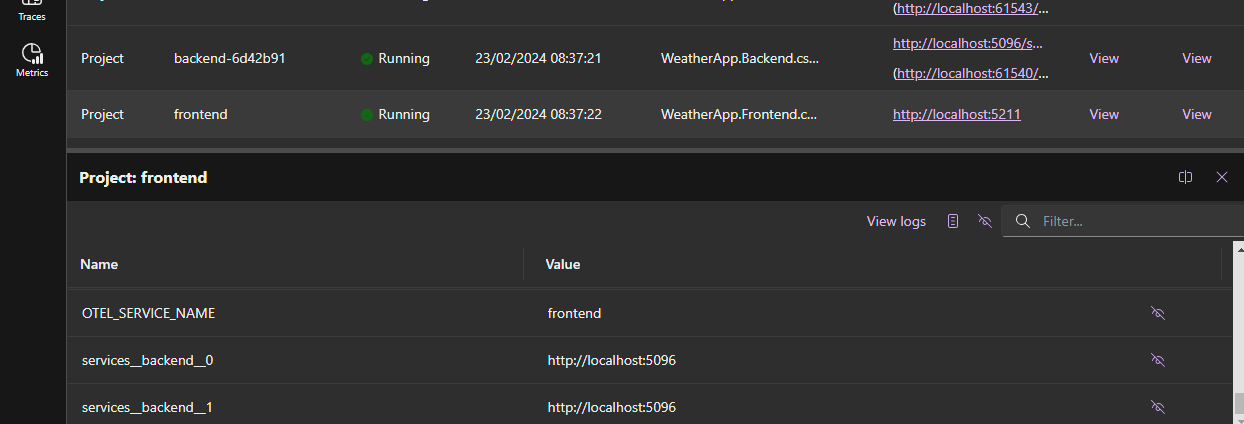
The orchestration makes the services to know each other this way. The backend gets the connection string to Redis via the environment variable. This is why the services can interact. So there is almost no magic here. Just C# to orchestrate and environment variables to connect the services to each other.
Deployment
As mentioned your cloud-native app will be orchestrated to be cloud-ready. You can easily deploy your application to your Azure subscription. The tool that helps you with that is the Azure Developer CLI (azd
). This CLI is super easy to use prepares your app for you and can do the deployment. After the installation of azd
you just use it.
With the console of your choice, cd to your solution folder and type azd login
. This will open up a browser that you can use to log in with your Azure account.
The following command will prepare your application to be ready for deployment:
azd init
It creates some configuration files and a Bycep
script to set up your environment on Azure. Take a look at it to learn about Bycep
.
The next command does the deployment:
azd up
If you own more than one subscription you are asked which one to use. The CLI is super awesome. It is an interactive one that guides you through the entire deployment. Just follow the instructions.
If the deployment is done your app is up and running on Azure. It is really that easy.
It sets all up on Azure. A Redis is up and running. Your apps are running in Azure Container Apps and if you would have a SQL Server configured in .NET Aspire, it would also set up a SQL Azure for you
Just don't use preview versions of .NET. That won't run on Azure and it took me some time to figure out why my cloud native app is not running on Azure. The easiest way to not stumble into that issue is to create a global.json
and pin your solution to an SDK version of .NET that is supported on Azure.
Conclusion
This is just an introduction post about .NET Aspire. I hope it gives you a good overview of it.
I will definitely follow the releases of .NET Aspire and I'm really looking forward to using the final release for the development of real applications that will go into production.
I really like it and will - for sure - write more deep dive about it. I also did a talk at the .NET user group Basel and would also do it at your user group, if you like. I'm also open to conference talks.
Just one thing I would really like to have is the Aspire portal to be deployed as well. I think this will be super helpful to monitor applications in production. As far as I know, there are no plans yet to have this portal as a tool for production. On the other hand, if you don't properly secure this portal, it could be a really dangerous security risk and all the information that the portal provides is also available on the Azure portal. So there isn't a real need for that.
Do you want to learn more about .NET Aspire? Follow the docs that are super complete and also contain super helpful tutorials about all the built in components: https://learn.microsoft.com/de-de/dotnet/aspire/
In der Klasse System.Environment hat Microsoft in .NET 8.0 die Eigenschaft IsPrivilegedProcess hinzugefügt.
Developing desktop apps for Windows is quite complex in 2024. There are some “old school” frameworks like WPF or WinForms (or even older stuff) and there is this confusing UWP (but I think it’s dead).
The “modern stack” seems to be WinUI
- so let’s take a look.
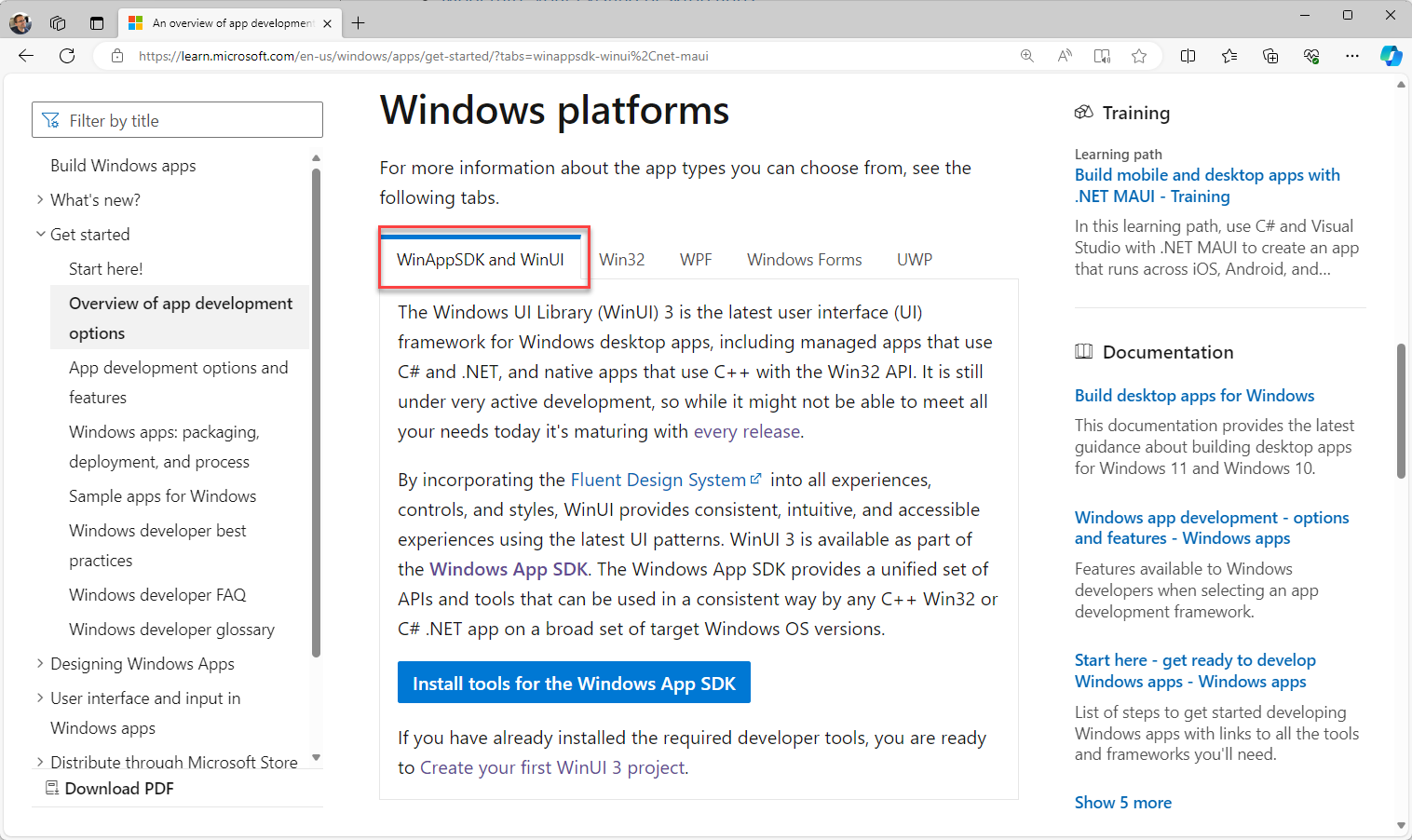
See here
What is WinUI?
WinUI is the “modern” version of WPF without the (dumb?) constraints from UWP. You can of course use your typical “Windows” programming languages (like C# or C++).
If you heard of UWP. The “Universal Windows Platform” was a good idea but failed, because - at least from my limited testing - the platform was very strict and you couldn’t do the same stuff that you can do with WPF/WinForms.
WinUI 1 and 2 were targeted at UWP (if I remember correctly) and with WinUI 3 Microsoft decided to lift those UWP constraints and with it we get a modern desktop stack based on the “known” XAML.
In summary:
WinUI 3 apps can be used for apps that run on Windows 11 and Windows 10 and can be distributed via the Microsoft Store and you can do the same crazy stuff that you love about WPF/WinForms.
Does anybody outside of Microsoft use WinUI?
WinUI is used in Windows 11, e.g. the settings or the new explorer - which is nice, but it would be good, if we found a non-Microsoft app that uses this tech, right?
Thankfully last week Apple decided to release Apple Music (and other apps) as a native Windows app and it seems (confirmed) like it was written with WinUI:
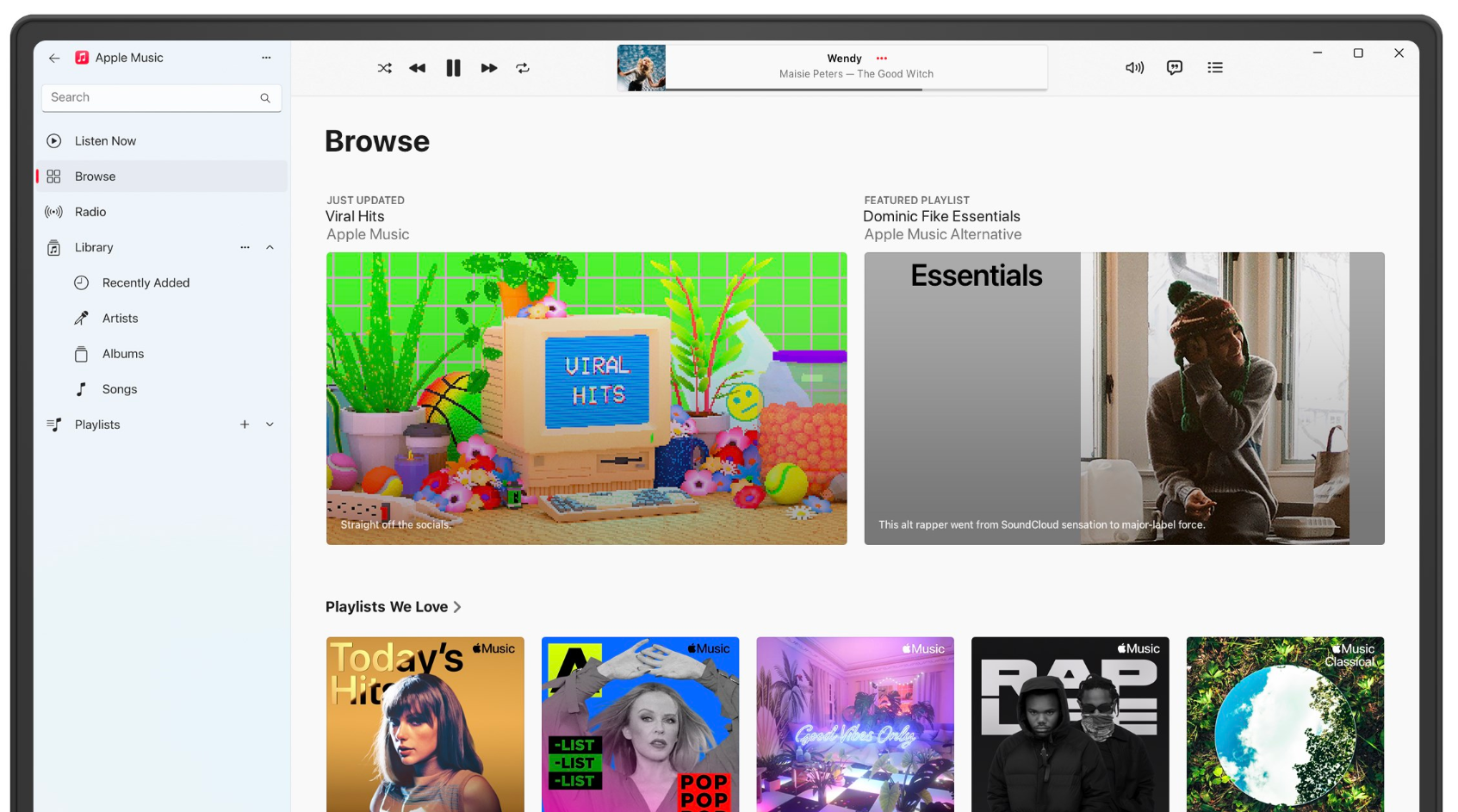
If Apple uses this tech, it seems “safe enough” for some exploration.
How to get started?
You will need Visual Studio 2022. Be aware, that even if you check all those desktop related workloads in the installer the WinUI 3 templates are still missing.
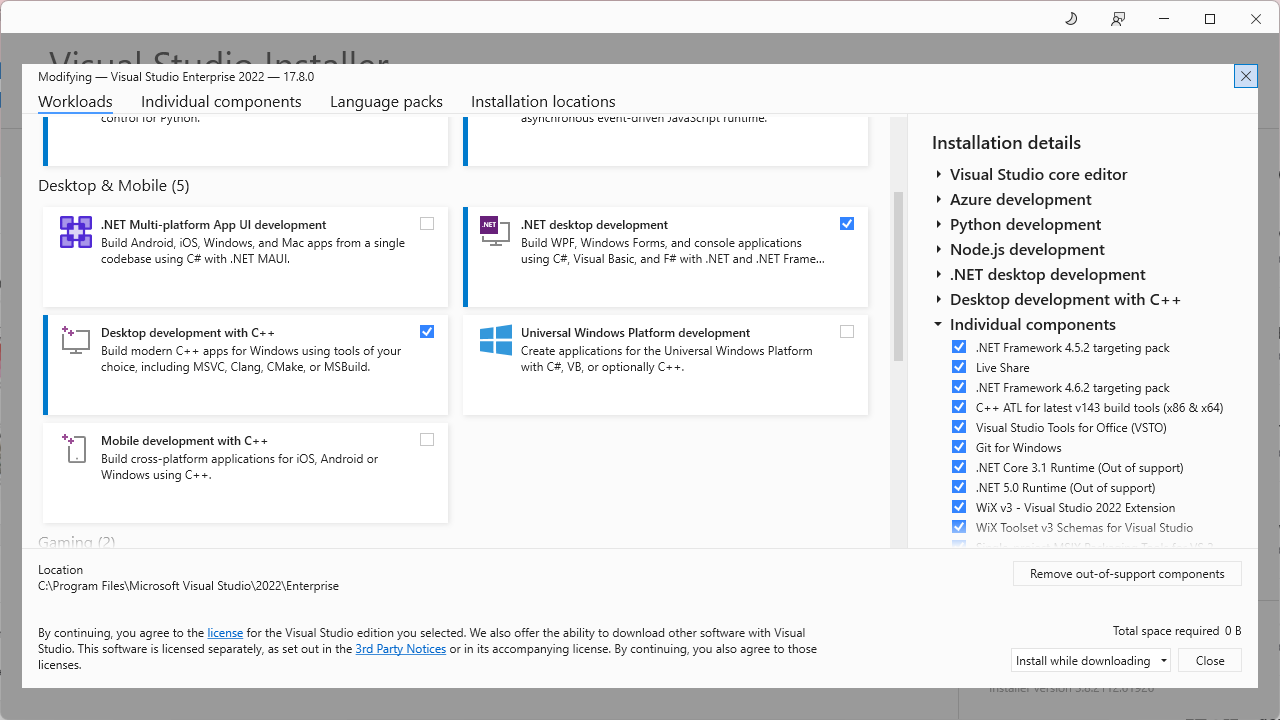
For the WinUI 3 templates you will need to install the Windows App SDK.
Visual Studio Templates
After the Windows App SDK is installed we finally have the templates in Visual Studio:
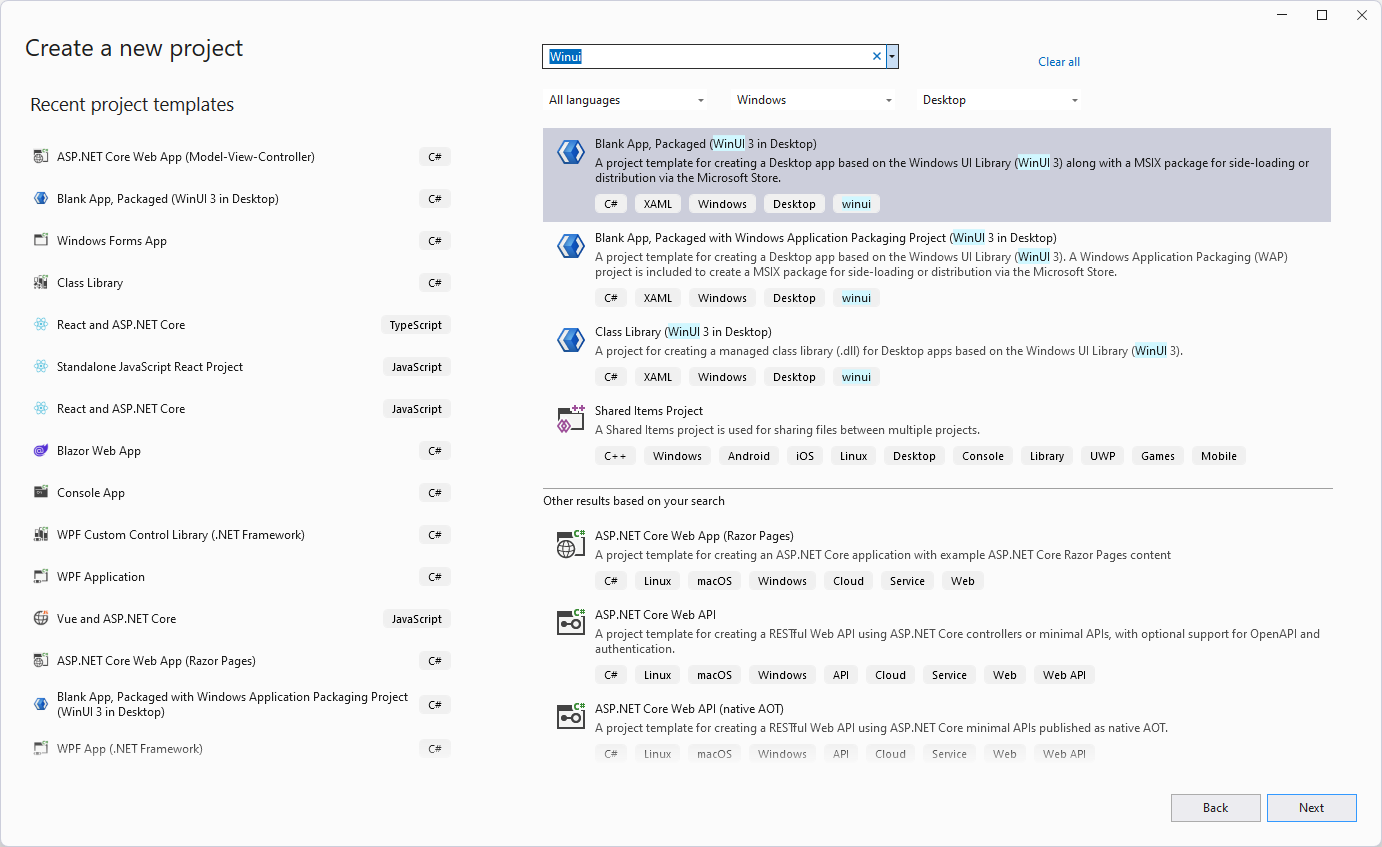
The default Blank App, Packaged (WinUI 3 in Desktop)
is… well… quite blank:
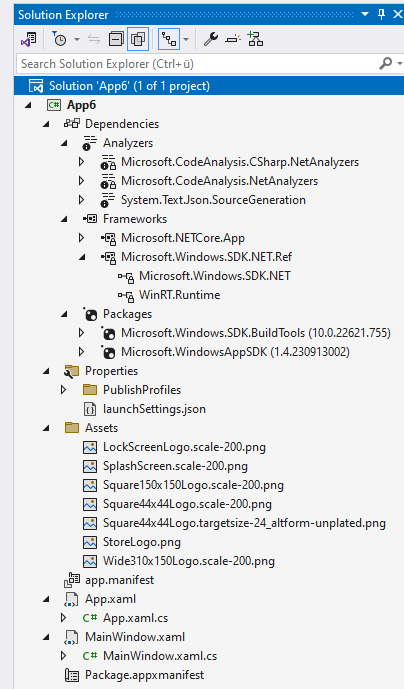
If you start the application, you will see this:
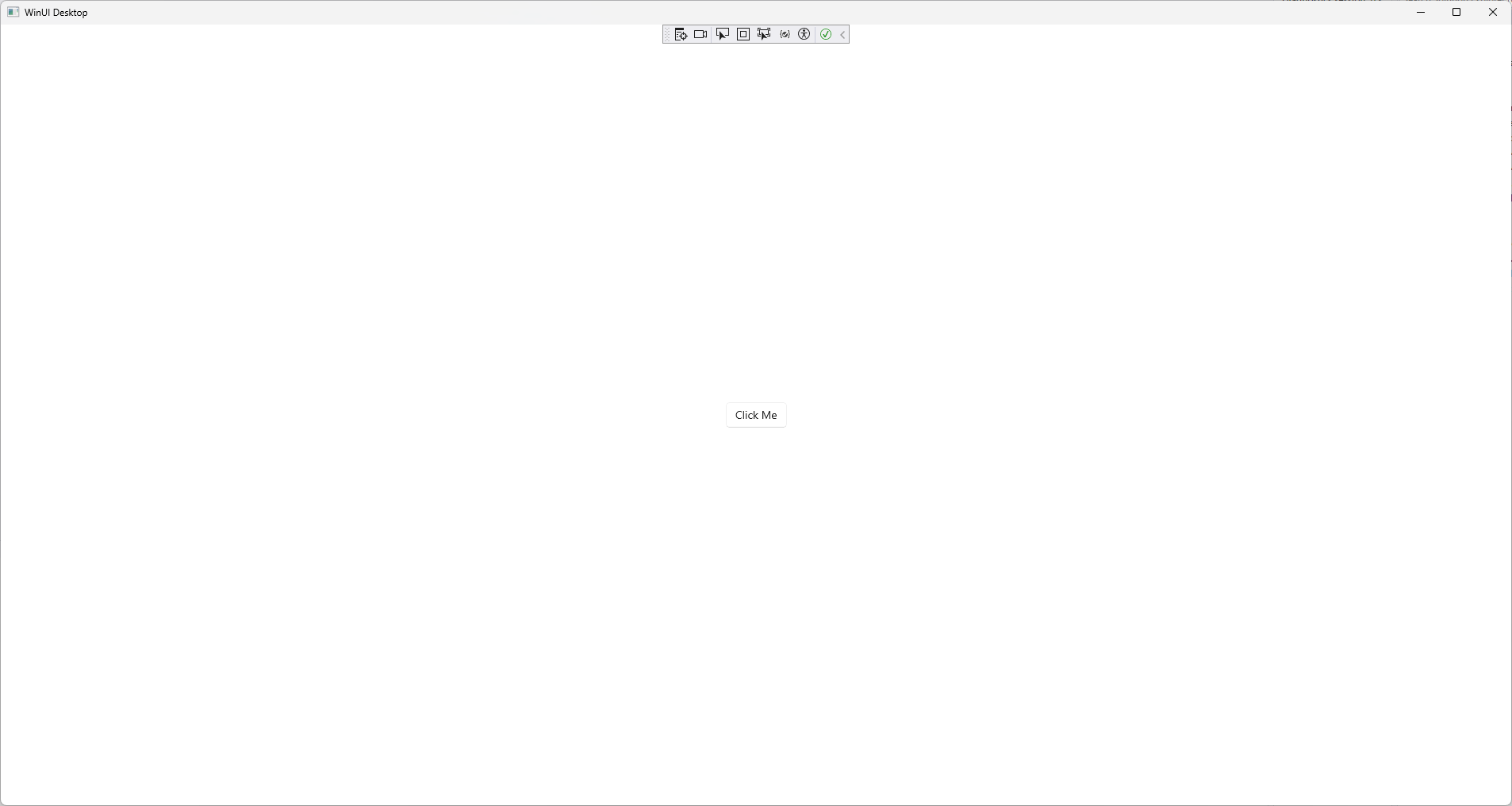
Packaged vs. Unpacked
If you check the toolbar, you will notice the App 6 (Package)
debug button. Packaged Apps
can access some Windows APIs (e.g. custom context menu extensions) that Unpackaged Apps
can’t. Unpackaged Apps
on the other hand act like WPF apps - e.g. they have a “normal” .exe
and can be distributed like any .exe
-file.
This documentation page should cover this topic.
Let’s say we want to have a “proper” myApp.exe
app, then the Unpackaged App
is the way to go. If you choose the App 6 (Unpackaged)
debug option you might see this weird error:
XamlCheckProcessRequirements();
Exception Unhandled:
System.DllNotFoundException: 'Unable to load DLL 'Microsoft.ui.xaml.dll' or one of its dependencies: The specified module could not be found. (0x8007007E)'
To fix this, you will need to add this to the .csproj
:
<PropertyGroup>
...
<WindowsPackageType>None</WindowsPackageType>
...
</PropertyGroup>
After that the debug button should start the application and you should be able to start the .exe
.
Samples
Ok, the most basic steps are done - now what?
To get a feeling about what is possible and what not, you should install the WinUI 3 Gallery app.
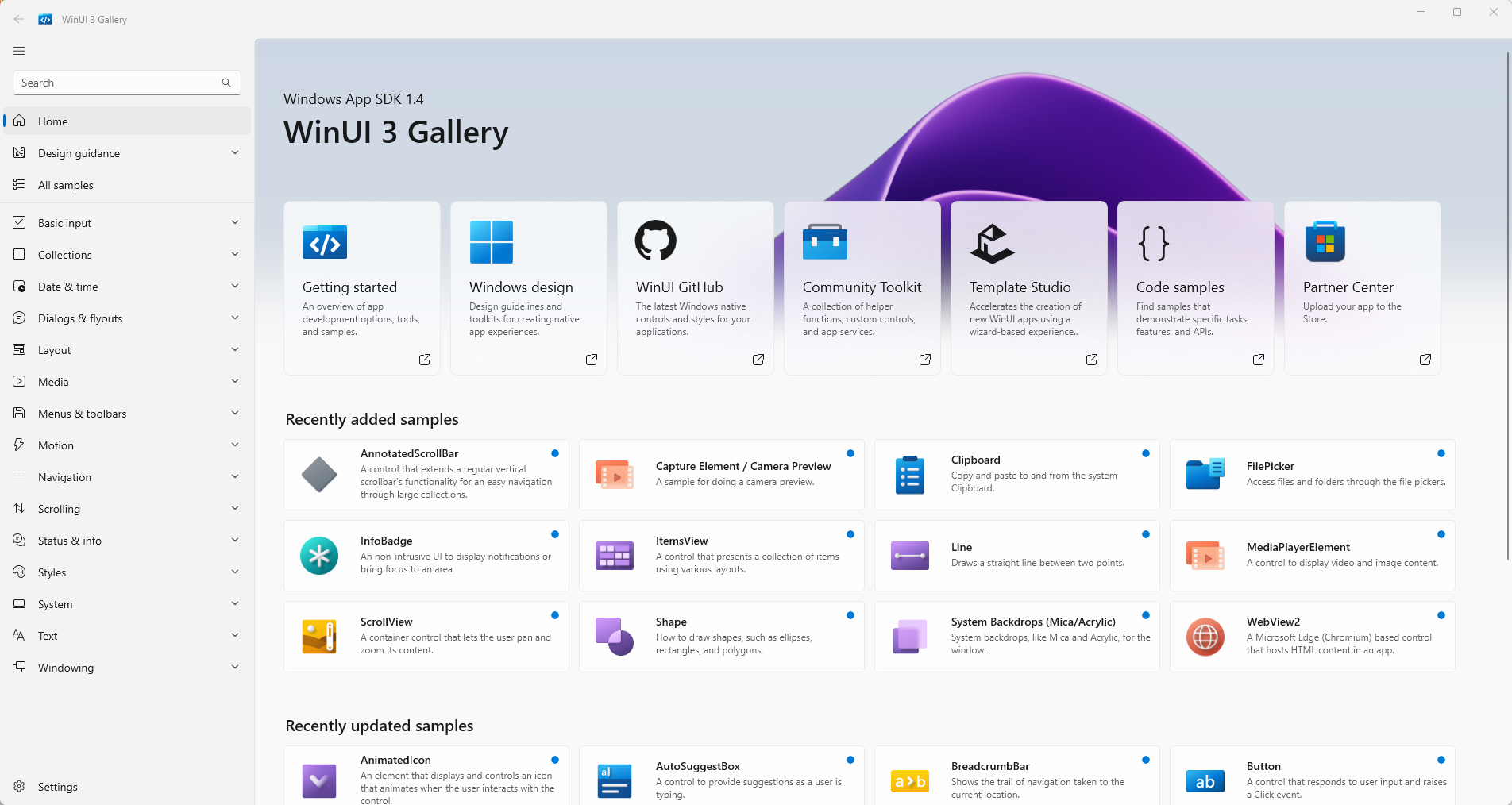
This application should give a some guidiance.
Hope this helps!
Note: I’m a beginner with WinUI 3 and just want to show other people the first few steps - if I miss something, just write me a comment! Thanks <3
In .NET 8.0 hat Microsoft die Annotationsklassen im Namensraum System.ComponentModel.DataAnnotations erweitert.
Der in C# 6.0 eingeführte Operator, der den Namen eines Bezeichners als String liefert, hatte bisher einige Einschränkungen, die in C# 12.0 entfallen.
Die Entwicklung von Web-UIs erfordert zu viele Frameworks und Tools, die zudem aufwendig und komplex zu integrieren sind. Könnte sich das im Jahr 2024 ändern?
Die aktuelle Version von Microsofts Programmiersprache C# erweitert sowohl reguläre Lambdas als auch Statement Lambdas.
In der aktuellen Version von C# lassen sich Methodenparameter als unveränderlich deklarieren.
If you’ve ever worked with the Windows operating system, especially in a programming context, you might have used the Process.Start(yourapp)
(e.g. Process.Start(Outlook)
) method in languages like C#.
This method is used to start a process - essentially to run an executable file. But have you ever stopped to think about how Windows knows where to find the executables you’re trying to run? Let’s dive into the inner workings of Windows and uncover this mystery.
Understanding the PATH Environment Variable
One of the first things that come into play is the PATH
environment variable. This variable is crucial for the operating system to locate the executables.
What is the PATH Variable?
The PATH
environment variable is a system-wide or user-specific setting that lists directories where executable files are stored. When you run a command in the command prompt or use Process.Start(...)
, Windows looks through these directories to find the executable file.
The PATH
environment variable can be viewed via the system settings:
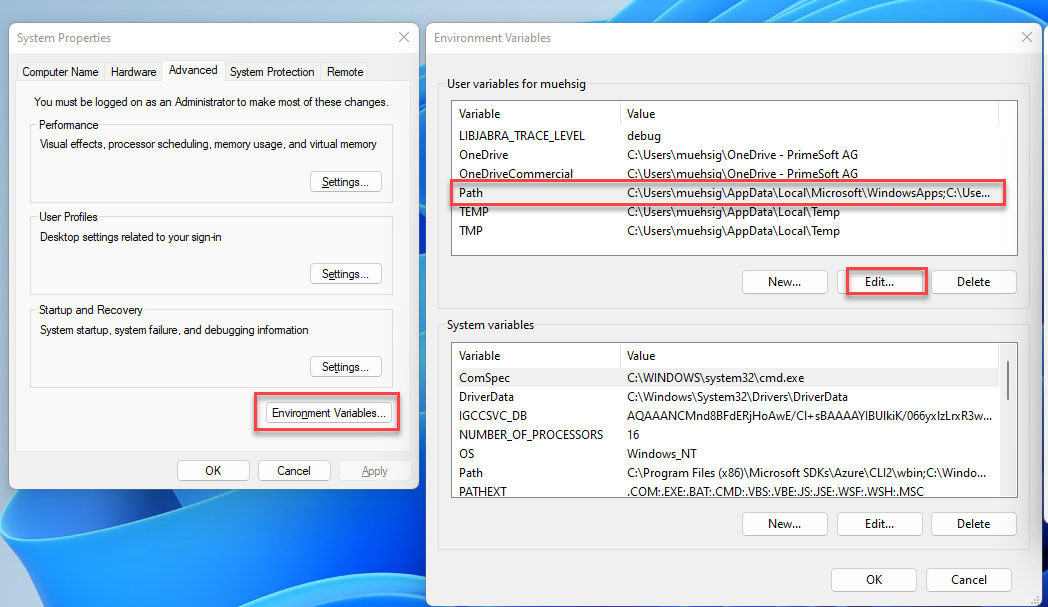
… there is also a nice editor now build into Windows for the PATH
environment variable:
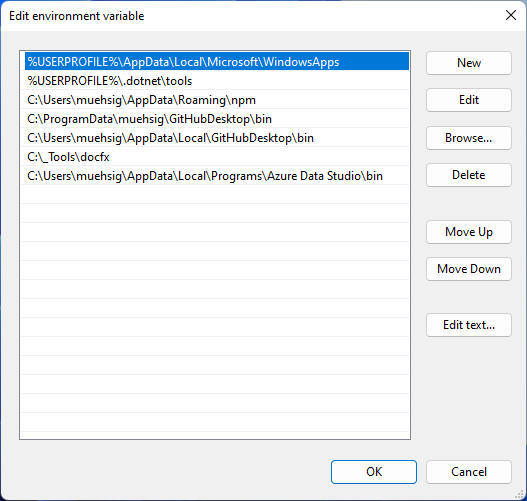
How Does PATH Work?
If the executable is not in the current directory, Windows searches through each directory specified in the PATH
variable. The order of directories in PATH
is important - Windows searches them in the order they are listed. If it finds the executable in one of these directories, it runs it.
However, the PATH
variable isn’t the only mechanism at play here.
The Role of App Paths in the Windows Registry
Another less-known but equally important component is the “App Paths” registry key. This key is located in HKEY_LOCAL_MACHINE\SOFTWARE\Microsoft\Windows\CurrentVersion\App Paths
.
Understanding App Paths
The App Paths
key is used to specify paths to specific applications. Each application can have its entry under the App Paths
key, which means that Windows can find and run these applications even if their directories are not listed in the PATH
variable.
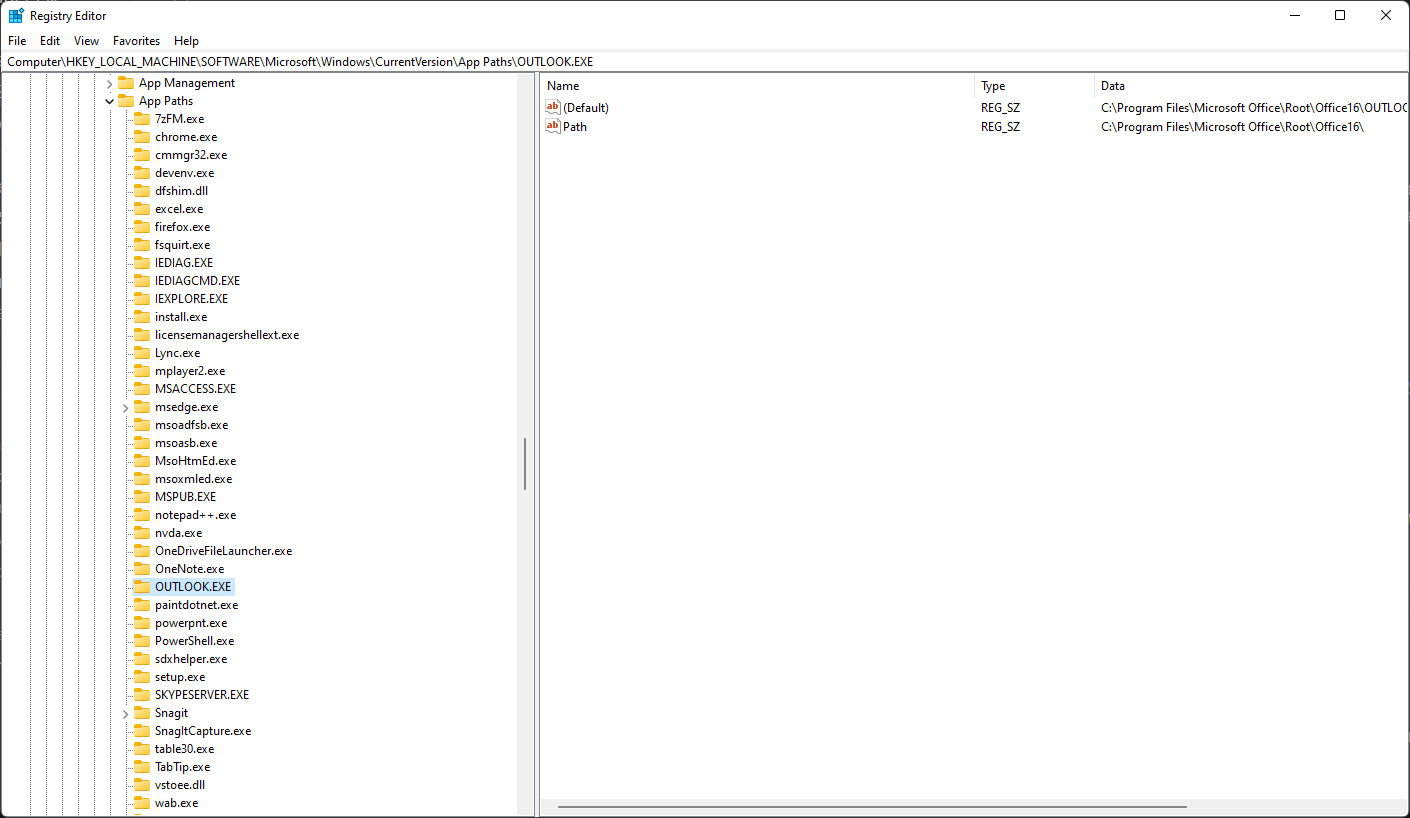
How Do App Paths Work?
When you use Process.Start(...)
and specify an application name like “OUTLOOK”, Windows first checks the App Paths registry key before it checks the PATH
variable. If it finds an entry for the application here, it uses this path to start the application. This is particularly useful for applications that are not in common directories or have multiple executables in different locations.
Conclusion
Both PATH
and App Paths
play significant roles. While PATH
is great for general-purpose directory searching (especially for system utilities and command-line tools), App Paths
is more specific and tailored for individual applications.
There are probably even more options out there besides PATH
and App Paths
- Windows is full of hidden gems like this 😉.
Fun fact: I only discovered App Paths
while debugging a problem. We use Process.Start(OUTLOOK)
to start Microsofts Outlook Client and I was wondering why this even works.
Hope this helps!
Die Vorträge in Ratingen behandeln die Neuerungen in der Syntax von C# 12.0 sowie in ASP.NET Core 8.0, Blazor 8.0 und Entity Framework Core 8.0.
If your .NET (“.NET Core”) program is running on a system that specifies strict proxy settings, you must either handle these settings in your application itself or use these environment variables.
Since I had this problem from time to time and the procedure was not 100% clear to me, I am now recording it here on the blog.
“DefaultProxy”
If you don’t specify any proxy, then the DefaultProxy
is used and depending on your operation system the following will be used:
(Copied from here)
For Windows: Reads proxy configuration from environment variables or, if those are not defined, from the user’s proxy settings.
For macOS: Reads proxy configuration from environment variables or, if those are not defined, from the system’s proxy settings.
For Linux: Reads proxy configuration from environment variables or, in case those are not defined, this property initializes a non-configured instance that bypasses all addresses.
The environment variables used for DefaultProxy initialization on Windows and Unix-based platforms are:
HTTP_PROXY: the proxy server used on HTTP requests.
HTTPS_PROXY: the proxy server used on HTTPS requests.
ALL_PROXY: the proxy server used on HTTP and/or HTTPS requests in case HTTP_PROXY and/or HTTPS_PROXY are not defined.
NO_PROXY: a comma-separated list of hostnames that should be excluded from proxying. Asterisks are not supported for wildcards; use a leading dot in case you want to match a subdomain. Examples: > NO_PROXY=.example.com (with leading dot) will match www.example.com, but will not match example.com. NO_PROXY=example.com (without leading dot) will not match www.example.com. This behavior might be > revisited in the future to match other ecosystems better.
Scenario: Web-App that needs external & “internal” Web-APIs
We often had the following problem:
Our web application needs to contact external services. This means, that we must use the proxy.
At the same time, our web application also wants to communicate with other web APIs on the same machine, but the proxy does not allow this (the proxy can’t return the request to the same machine - not sure why).
It should be noted that the “IIS account” or “Network Service” did NOT have a proxy setting itself, i.e. the “User Proxy Settings” were always empty.
Solution:
We used the following proxy settings and it worked:
ALL_PROXY = proxyserver.corp.acme.com
NO_Proxy = internalserver.corp.acme.com
Our web application and our internal web api were running on “internalserver.corp.acme.com”. Each request to external services were routed through the proxy and each “internal” request didn’t touch the proxy.
IE-Proxy Settings:
This solution should work fine on “Server-Environments”. If you have a desktop application, then the “Default Proxy” handling should do the trick. In some special cases the “IE proxy setting” handling might be needed. If you want to learn more about this, read this blogpost: How to use IE proxy settings with HttpClient.
Hope this helps!
Typen können in der aktuellen Version von C# durch das Schlüsselwort using einen alternativen Namen erhalten.
Das aktuelle Release der Programmiersprache C# vereinfacht das Initialisieren von Collections wie Listen.
Die Programmiersprache C# bietet in Version 12.0 die Möglichkeit, über eine Parameterliste hinter dem Typnamen einen Primärkonstruktor zu definieren.
Blazor bekommt in .NET 8.0 einen Auto-Rendering-Modus und Blazor Static-Server-Rendering.
Due to the regular calls for articles about the SOLID principles, I decided some time ago to offer this series in the form of a small book. This book can now be ordered (as a paperback and as an e-book) from all well-known bookshops and digital platforms. In addition to the SOLID principles, the book also addresses the KISS, DRY, LoD and YAGNI principles.
Of course, the previous articles are still available free of charge here on the blog. This means that everyone is free to decide whether they would prefer to read the articles online, on an e-book reader or as a printed book.
The price for the almost 100-page book is €6.99 for the printed version (ISBN-13: 9783757892227) and €4.99 for the e-book (ISBN-13: 9783758356148).
The 5 SOLID principles were presented in the previous posts. In addition to the SOLID principles, however, there are other principles that are also briefly presented here. What all these principles have in common is the goal of making software more maintainable and more reusable.
Don’t Repeat Yourself (DRY)
The DRY principle states (as the name suggests) that program code should not be duplicated unnecessarily. Instead, a function should be implemented only once and called at desired points in the program.
The DRY principle can help improve the maintainability of code, as it becomes easier to make changes to a function if it is implemented in only one place in the program code. In addition, the DRY principle can help reduce errors in the program, since duplicated code often leads to unexpected behaviour when a change is made in only one of the duplicated locations. Thus the DRY principle is an important principle in the software development, which can contribute to the improvement of the code quality.
Although the DRY principle is easy to understand and implement, it is probably the most disregarded principle. Because nothing is easier than to repeat source code by copy & paste. Especially when the time pressure is particularly high. Therefore, you should always try to implement shared functions in separate modules.
The following short example shows the application of the DRY principle. A PLC program receives different temperature values from several sensors. All temperature values are to be displayed in an HMI and written to a log file. To make the temperature values more readable, the formatting should be done in the PLC:
FUNCTION F_DisplayTemperature : STRING
VAR_INPUT
fSensorValue : LREAL;
bFahrenheit : BOOL;
END_VAR
IF (fSensorValue > 0) THEN
IF (bFahrenheit) THEN
F_DisplayTemperature := CONCAT('Temperature: ',
REAL_TO_FMTSTR(fSensorValue, 1, TRUE));
F_DisplayTemperature := CONCAT(F_DisplayTemperature, ' °C');
ELSE
F_DisplayTemperature := CONCAT('Temperature: ',
REAL_TO_FMTSTR(fSensorValue * 1.8 + 32, 1, TRUE));
F_DisplayTemperature := CONCAT(F_DisplayTemperature, ' °F');
END_IF
ELSE
F_DisplayTemperature := 'No sensor data available';
END_IF
In this example the function F_DisplayTemperature() is implemented only once. For the formatting of the temperature values this function is called at the desired places in the program. By avoiding duplicated code, the program becomes clearer and easier to read. If, for example, it is necessary to change the number of decimal places, this only has to be done in one place, namely in the function F_DisplayTemperature().
In addition to the use of functions, inheritance can also help to comply with the DRY principle by relocating a functionality in a base FB and using it by all derived FBs.
However, there may be cases in which the DRY principle should be deliberately violated. This is always the case if the readability of the source code is worsened by the use of DRY. Thus for the circle computation the formula for the circumference (U=2rπ) or for the area (A=r2π) is sufficiently readable. An outsourcing into separate functions does not increase the code quality, but only the dependence to further modules, in which the functions for the circle computation are. Instead, a global constant should be created for π and used in the calculations.
In summary, the DRY principle helps make program code cleaner and shorter by avoiding code duplication.
Law Of Demeter (LoD)
The Law of Demeter is another principle whose observance can significantly minimize the couplings between function blocks. The Law of Demeter specifies that only elements in the immediate vicinity should be accessed from a function block (or method or function). In concrete terms, this means that only accesses to the following elements are permitted:
- Variables of the own function block (everything between VAR/END_VAR)
- Methods/properties of the own function block
- Methods/properties of the function blocks that were created in the own function block
- Parameters passed to methods or function blocks (VAR_INPUT)
- Global constants or parameters contained in a parameter list
The Law of Demeter could therefore also be called: Don’t talk to strangers. Strangers are elements that are not directly present in the function block. In contrast, the own elements are called friends.
Also this principle originates from the 1980iger years, thus from the time, in which the object-oriented software development increased strongly in popularity. The name Demeter is to be led back on a software project of the same name, in which this principle was recognized for the first time (Demeter is in the Greek mythology the sister of Zeus and the Goddess of the agriculture). At the end of the 1980s, this principle was further elaborated by Ian Holland and Karl J. Lieberherr and published under the title Assuring Good Style for Object-Oriented Programs.
The following graphic is intended to illustrate the Law of Demeter in a little more detail:
FB_A contains an instance of FB_B (fbB). Therefore, FB_A can directly access the methods and properties of FB_B.
FB_B contains an instance of FB_C. Therefore, FB_B can access FB_C directly.
FB_B could offer a property or a method that returns the reference to FB_C (refC). Access from FB_A to the instance of FB_C via FB_B would thus theoretically be possible:
nValue := fbB.refC.nValue;
The instance on FB_C is created in FB_B. If FB_A accesses this instance directly, a fixed coupling between FB_A and FB_C is created. This fixed coupling can lead to problems in the care, maintenance and testing of the program. If FB_A is tested, not only FB_B must be present, but FB_C as well. A frequent violation of the Law of Demeter is therefore also helpful in the early detection of maintenance problems.
Even creating a corresponding local variable in which the reference to FB_C is stored does not solve the actual problem:
refC : REFERENCE TO FB_C;
refC REF= fbB.refC;
nValue := refC.nValue;
At first glance, these dependencies are not always apparent, as FB_C is accessed indirectly via FB_B.
Example
Here is a concrete example that illustrates the problem again and also offers a solution.
The function blocks FB_Building, FB_Floor, FB_Room and FB_Lamp represent the structure of a building and its lighting. The building consists of 5 floors, each containing 20 rooms and each room contains 10 lamps.
Each function block contains the corresponding instances of the underlying elements. The function blocks each provide a property that offers a reference to these elements. FB_Lamp contains the property nPowerConsumption, via which the current power consumption of the lamp is output.
A function is to be developed that determines the power consumption of all lamps in the building.
One solution could be to access each individual lamp via several nested loops and add up the power consumption:
FUNCTION F_CalcPowerConsumption : UDINT
VAR_INPUT
refBuilding : REFERENCE TO FB_Building;
END_VAR
VAR
nFloor, nRoom, nLamp : INT;
END_VAR
IF (NOT __ISVALIDREF(refBuilding)) THEN
F_CalcPowerConsumption := 0;
RETURN;
END_IF
FOR nFloor := 1 TO 5 DO
FOR nRoom := 1 TO 20 DO
FOR nLamp := 1 TO 10 DO
F_CalcPowerConsumption := F_CalcPowerConsumption + refBuilding
.refFloors[nFloor]
.refRooms[nRoom]
.refLamps[nLamp].nPowerConsumption;
END_FOR
END_FOR
END_FOR
The „diving‟ into the object structure down to each lamp seems somehow impressive. But this makes the function dependent on all function blocks, even those that are only indirectly addressed via a reference.
The access of refBuilding to refFloors does not violate the Law of Demeter, since refFloors is a direct property of FB_Building. However, all further accesses to the references have the consequence that our function also becomes dependent on the other function blocks.
If, for example, the structure of FB_Room or FB_Floor changes, the function for power consumption may also have to be adapted.
To comply with the Law of Demeter, each function block could offer a method (CalcPowerConsumption()) in which the power consumption is calculated. In each of these methods, the underlying method CalcPowerConsumption() is called:
The CalcPowerConsumption() method in FB_Building only accesses its own elements. In this case, it accesses the property refFloors to call the method CalcPowerConsumption() of FB_Floor:
METHOD CalcPowerConsumption : UDINT
VAR
nFloor : INT;
END_VAR
FOR nFloor := 1 TO 5 DO
CalcPowerConsumption := CalcPowerConsumption +
refFloors[nFloor].CalcPowerConsumption();
END_FOR
In CalcPowerConsumption() of FB_Floor, only FB_Room is accessed:
METHOD CalcPowerConsumption : UDINT
VAR
nRoom : INT;
END_VAR
FOR nRoom := 1 TO 20 DO
CalcPowerConsumption := CalcPowerConsumption +
refRooms[nRoom].CalcPowerConsumption();
END_FOR
Finally, the power consumption of all lamps in the room is calculated in FB_Room:
METHOD CalcPowerConsumption : UDINT
VAR
nLamp : INT;
END_VAR
FOR nLamp := 1 TO 10 DO
CalcPowerConsumption := CalcPowerConsumption +
refLamps[nLamp].nPowerConsumption;
END_FOR
The structure of the function F_CalcPowerConsumption() is thus much simpler:
FUNCTION F_CalcPowerConsumption : UDINT
VAR_INPUT
refBuilding : REFERENCE TO FB_Building;
END_VAR
IF (NOT __ISVALIDREF(refBuilding)) THEN
F_CalcPowerConsumption := 0;
RETURN;
END_IF
F_CalcPowerConsumption := refBuilding.CalcPowerConsumption();
After this adjustment, F_CalcPowerConsumption() is only dependent on FB_Building and its method CalcPowerConsumption(). How FB_Building calculates the power consumption in CalcPowerConsumption() is irrelevant for F_CalcPowerConsumption(). The structure of FB_Room or FB_Floor could change completely, F_CalcPowerConsumption() would not have to be adapted.
The first variant, in which all function blocks were iterated through, is very susceptible to changes. No matter which function block the structure changes, an adjustment of F_CalcPowerConsumption() would be necessary every time.
Sample 1 (TwinCAT 3.1.4024) on GitHub
However, it must be taken into account that nested structures do make sense. The Law of Demeter does not have to be applied here. It can be helpful to distribute the configuration data hierarchically over several structures in order to increase readability.
Keep It Simple, Stupid (KISS)
The KISS principle states that code should be as „simple‟ as possible so that it is as easy to understand as possible and thus effective to maintain. Here, „simple‟ is also to be understood as „plain‟. This means a simplicity that tries to leave out the unnecessary but still fulfils the customer’s requirements. By following the KISS principle, a system is:
- easy to understand
- easy to extend
- easy to maintain
If the requirement is to sort ten million records, using the bubblesort algorithm would be simple to implement, but the low speed of the algorithm will not meet the client’s requirements. Therefore, a solution must always be found that meets the customer’s required expectations, but whose implementation is as simple (plain) as possible.
Basically, two types of requirements are to be distinguished:
Functional requirement: The customer or stakeholder demands a specific feature. The exact requirements for this feature are then defined together with the customer and only then is it implemented. Functional requirements extend an application with clear functions (features) desired by the customer.
Non-functional requirements: A non-functional requirement is, for example, the splitting of an application into different modules or the provision of interfaces, e.g. to enable unit tests. Non-functional requirements are performance features that are not necessarily visible to the customer. However, these may be necessary so that the software system can be maintained and serviced.
The KISS principle is always about the non-functional requirements. The focus is on the „how‟. In other words, the question of how the required functions are achieved. The YAGNI principle, which is described in the following chapter, refers to the functional requirements. Here the focus is on the „what‟.
The KISS principle can be applied at several levels:
Formatting source code
Although the following source code is very compact, the KISS principle is violated here because it is difficult to understand and thus very error-prone:
IF(x<=RT[k-1](o[n+2*j]))THEN WT[j+k](l AND NOT S.Q);END_IF;
IF(x>RI[k+1](o[n+2*k]))THEN WO[j-k](l OR NOT S.Q);END_IF;
The source code should be formatted in such a way that the sequence is better recognised. Also, the identifiers for variables and functions should be chosen in such a way that their meaning is easier to understand.
Unnecessary source code
Source code that does not help to improve readability also violates the KISS principle:
bCalc := F_CalcFoo();
IF (bCalc = TRUE) THEN
bResult := TRUE;
ELSE
bResult := FALSE;
END_IF
Although the source code is well structured and the identifiers have been chosen so that their meaning is easier to recognise, the source code can be significantly reduced:
This one line is much easier to understand than the 6 lines before. The source code is „simpler‟, with the same range of functions.
Software design / software architecture
The design or structure of software can also violate the KISS principle. If, for example, a complete SQL database is used to store configuration data, although a text file would suffice, the KISS principle is also violated.
The division of a PLC programme into several CPU cores only makes sense if it also produces a practical benefit. In this case, appropriate mechanisms must be built into a PLC program to synchronise access to shared resources. These increase the complexity of the system considerably and should only be used if the application requires them.
I have deliberately placed the chapters on the KISS principle and the YAGNI principle at the end. From here, I would like to take a brief look back at the beginning of the series on the SOLID principles.
When introducing the SOLID principles, I occasionally pointed out the danger of overengineering. Abstractions should only be provided if they are necessary for the implementation of features.
To clarify this, I will use the example for the explanation of the SOLID principles again (see: IEC 61131-3: SOLID – The Dependency Inversion Principle).
There is a fixed dependency between the three lamp types and the controller. If the application is to be extended by another lamp type, it is necessary to adapt the programme at various points. By applying the Dependency Inversion Principle (DIP) and the Single Responsibility Principle (SRP), the programme became much more flexible. The integration of additional lamp types has been significantly simplified. However, the complexity of the programme was also significantly increased by these adjustments, as the UML diagram shows:
(abstract elements are displayed in italics)
Before additional levels of abstraction are realised by applying the SOLID principles, one should always critically question the extra effort involved.
The structure of the first variant is completely sufficient if the program is used exclusively in a project to this extent. The program is small enough to understand the structure of the software and to make small adjustments. The KISS principle was followed. No more complexity than necessary has been built in.
However, if the first variant is only an intermediate step, e.g. in the development of a comprehensive light management system, it is to be expected that the application will increase in complexity. It is also possible that at a later stage the development will have to be distributed among several people. The use of unit tests is another point that justifies the implementation of SOLID principles. Without decoupling the individual lamp types through interfaces, the use of unit tests is difficult or even impossible. Here, too, the KISS principle is not violated. The KISS principle must therefore always be considered in context.
You Ain’t Gonna Need It (YAGNI)
YAGNI stands for You Ain’t Gonna Need It and also means You will not need it. It means that in software development you should only implement the features that are needed. No functions or features should be implemented, which might be needed someday.
In contrast to the KISS principle, which always focuses on the non-functional requirements, the YAGNI principle focuses on the functional requirements.
When developing software, it can be tempting to implement additional features without a concrete requirement. This can be the case, for example, if features are implemented during development without consulting the customer, in the firm belief that the customer will demand them later.
Referring to our example above, the YAGNI principle would be violated if the operating hours recording were implemented (see: IEC 61131-3: SOLID – The Interface Segregation Principle), although this was not requested by the customer.
If it is determined during development that a particular feature could be useful, it should only be implemented after consultation with the customer. Otherwise, a system will gradually receive more and more source code for features that no one needs.
This example makes it clear once again that all the principles described so far are not fixed rules or even laws. However, the principles are a powerful tool for improving the code quality of software.
In den vorherigen Posts wurden die 5 SOLID-Prinzipien vorgestellt. Neben den SOLID-Prinzipien gibt es noch weitere Prinzipien, von denen ich einige ebenfalls kurz vorgestellen möchte. All diese Prinzipen haben das gemeinsame Ziel, die Wartbarkeit und die Wiederverwendbarkeit von Software zu verbessern.
Don’t Repeat Yourself (DRY)
Das DRY-Prinzip besagt (wie der Name schon vermuten lässt), dass man Programmcode nicht unnötig duplizieren sollte. Stattdessen sollte eine Funktion nur einmal implementiert und an gewünschten Stellen im Programm aufgerufen werden.
Das DRY-Prinzip kann helfen, die Wartbarkeit von Code zu verbessern, da es einfacher wird, Änderungen an einer Funktion vorzunehmen, wenn sie nur an einer Stelle im Programmcode implementiert wurde. Außerdem kann das DRY-Prinzip dazu beitragen, Fehler im Programm zu reduzieren, da duplizierter Code oft zu unerwarteten Verhaltensweisen führt, wenn eine Änderung nur an einer der duplizierten Stellen vorgenommen wird. Somit ist das DRY-Prinzip ein wichtiger Grundsatz in der Softwareentwicklung, welcher zur Verbesserung der Codequalität beitragen kann.
Obwohl das DRY-Prinzip einfach zu verstehen und umzusetzen ist, ist es wahrscheinlich das am meisten missachtete Prinzip. Denn nichts ist einfacher, als Quellcode durch Copy & Paste zu wiederholen. Gerade dann, wenn der Zeitdruck besonders hoch ist. Deshalb sollte man sich immer bemühen, gemeinsam genutzte Funktionen in separate Module zu implementieren.
Das folgende kurze Beispiel zeigt die Anwendung des DRY-Prinzips. Ein SPS-Programm erhält von mehreren Sensoren unterschiedliche Temperaturwerte. Alle Temperaturwerte sollen in einem HMI angezeigt und in eine Log-Datei geschrieben werden. Damit die Temperaturwerte besser lesbar sind, soll die Formatierung in der SPS erfolgen:
FUNCTION F_DisplayTemperature : STRING
VAR_INPUT
fSensorValue : LREAL;
bFahrenheit : BOOL;
END_VAR
IF (fSensorValue > 0) THEN
IF (bFahrenheit) THEN
F_DisplayTemperature := CONCAT('Temperature: ', REAL_TO_FMTSTR(fSensorValue, 1, TRUE));
F_DisplayTemperature := CONCAT(F_DisplayTemperature, ' °C');
ELSE
F_DisplayTemperature := CONCAT('Temperature: ', REAL_TO_FMTSTR(fSensorValue * 1.8 + 32, 1, TRUE));
F_DisplayTemperature := CONCAT(F_DisplayTemperature, ' °F');
END_IF
ELSE
F_DisplayTemperature := 'No sensor data available';
END_IF
In diesem Beispiel wird die Funktion F_DisplayTemperature() nur einmal implementiert. Für die Formatierung der Temperaturwerte wird diese Funktion an den gewünschten Stellen im Programm aufgerufen. Durch das Vermeiden von dupliziertem Code wird das Programm übersichtlicher und einfacher zu lesen. Ist es z.B. notwendig die Anzahl der Nachkommerstellen zu verändern, so muss dieses nur an einer Stelle, nämlich in der Funktion F_DisplayTemperature(), erfolgen.
Neben den Einsatz von Funktionen kann auch die Vererbung helfen das DRY-Prinzip einzuhalten, indem eine Funktionalität in einen Basis-FB verlagert und von allen abgeleiteten FBs verwendet wird.
Es kann aber Fälle geben, in denen das DRY-Prinzip bewusst verletzt werden sollte. Dieses ist immer dann der Fall, wenn sich durch den Einsatz von DRY die Lesbarkeit des Quellcode verschlechtert. So ist für die Kreisberechnung die Formel für den Umfang (U=2rπ) oder für die Fläche (A=r2π) ausreichend lesbar. Eine Auslagerung in separate Funktionen erhöht nicht die Codequalität, sondern nur die Abhängigkeit zu weiteren Modulen, in denen sich die Funktionen für die Kreisberechnung befinden. Stattdessen sollte für π eine globale Konstante angelegt und in den Berechnungen verwendet werden.
Zusammenfassend lässt sich sagen, dass das DRY-Prinzip dazu beiträgt, das Programmcode sauberer und kürzer wird, indem es die Duplizierung von Code vermeidet.
Law Of Demeter (LoD)
Das Law of Demeter ist ein weiteres Prinzip, dessen Beachtung die Kopplungen zwischen Funktionsblöcken deutlich minimieren kann. Das Law of Demeter legt fest, dass aus einem Funktionsblock (bzw. Methode oder Funktion) nur auf Elemente in unmittelbarer Nähe zugegriffen werden sollte. Konkret bedeutet dieses, dass nur Zugriffe auf die folgenden Elemente erlaubt sind:
- Variablen des eigenen Funktionsblocks (alles zwischen VAR/END_VAR)
- Methoden/Eigenschaften des eigenen Funktionsblocks
- Methoden/Eigenschaften der Funktionsblöcke die im eigenen Funktionsblock angelegt wurden
- Parameter die an Methoden oder Funktionsblöcke übergeben wurden (VAR_INPUT)
- Globale Konstanten oder Parameter die in einer Parameterliste enthalten sind
Das Law of Demeter könnte somit auch heißen: Don’t talk to strangers. Als Strangers (Fremde) werden hierbei die Elemente bezeichnet, die nicht unmittelbar in dem Funktionsblock vorhanden sind. Im Gegensatz dazu, werden die eigenen Elemente Friends (Freunde) genannt.
Auch dieses Prinzip stammt aus den 1980iger Jahren, also aus der Zeit, in der die objektorientierte Softwareentwicklung stark an Popularität zugenommen hat. Der Name Demeter ist auf ein gleichnamiges Softwareprojekt zurückzuführen, in dem dieses Prinzip erstmal erkannt wurde (Demeter ist in der griechischen Mythologie die Schwester von Zeus und die Göttin der Landwirtschaft). Ende der 1980iger Jahre wurde dieses Prinzip von Ian Holland und Karl J. Lieberherr weiter ausgearbeitet und unter dem Titel Assuring Good Style for Object-Oriented Programs veröffentlicht.
Die folgende Grafik soll das Law of Demeter etwas genauer verdeutlichen:
In FB_A ist eine Instanz von FB_B (fbB) enthalten. Deshalb kann FB_A direkt auf die Methoden und Eigenschaften von FB_B zugreifen.
FB_B enthält eine Instanz von FB_C. Deshalb kann FB_B direkt auf FB_C zugreifen.
FB_B könnte eine Eigenschaft oder eine Methode anbieten, welche die Referenz auf FB_C zurückgibt (refC). Ein Zugriff aus FB_A auf die Instanz von FB_C über FB_B wäre somit theoretisch möglich:
nValue := fbB.refC.nValue;
Die Instanz auf FB_C wird in FB_B angelegt. Wenn FB_A auf diese Instanz direkt zugreift, entsteht eine feste Kopplung zwischen FB_A und FB_C. Diese feste Kopplung kann zu Problemen bei der Pflege, Wartung und dem Testen des Programms führen. Wird FB_A getestet, so muss nicht nur FB_B vorhanden sein, sondern auch FB_C. Ein häufiges Verletzen des Law of Demeter ist somit auch hilfreich bei der Früherkennung von Wartungsproblemen.
Auch das Anlegen einer entsprechenden lokalen Variablen, in der die Referenz auf FB_C abgelegt wird, löst das eigentliche Problem nicht:
refC : REFERENCE TO FB_C;
refC REF= fbB.refC;
nValue := refC.nValue;
Auf dem ersten Blick sind diese Abhängigkeiten nicht immer zu erkennen, da der Zugriff auf FB_C indirekt über FB_B erfolgt.
Beispiel
Hierzu ein konkretes Beispiel, welches das Problem nochmal verdeutlicht und auch einen Lösungsansatz anbietet.
Mit den Funktionsblöcken FB_Building, FB_Floor, FB_Room und FB_Lamp wird die Struktur eines Gebäudes und dessen Beleuchtung abgebildet. Das Gebäude besteht aus 5 Etagen, in der sich jeweils 20 Räume befinden und jeder Raum enthält 10 Lampen.
In jedem Funktionsblock sind die entsprechenden Instanzen der darunterliegenden Elemente enthalten. Die Funktionsblöcke stellen jeweils eine Eigenschaft zur Verfügung, welche eine Referenz auf diese Elemente anbietet. FB_Lamp enthält die Eigenschaft nPowerConsumption, über der die aktuelle Leistungsaufnahme der Lampe ausgegeben wird.
Es soll eine Funktion entwickelt werden, welche die Leistungsaufnahme aller Lampen in dem Gebäude ermittelt.
Ein Lösungsansatz könnte darin bestehen, dass über mehrere verschachtelte Schleifen auf jede einzelne Lampe zugegriffen und die Leistungsaufnahme addiert wird:
FUNCTION F_CalcPowerConsumption : UDINT
VAR_INPUT
refBuilding : REFERENCE TO FB_Building;
END_VAR
VAR
nFloor, nRoom, nLamp : INT;
END_VAR
IF (NOT __ISVALIDREF(refBuilding)) THEN
F_CalcPowerConsumption := 0;
RETURN;
END_IF
FOR nFloor := 1 TO 5 DO
FOR nRoom := 1 TO 20 DO
FOR nLamp := 1 TO 10 DO
F_CalcPowerConsumption := F_CalcPowerConsumption + refBuilding
.refFloors[nFloor]
.refRooms[nRoom]
.refLamps[nLamp].nPowerConsumption;
END_FOR
END_FOR
END_FOR
Das „Eintauchen‟ in die Objektstruktur bis hinunter zu jeder Lampe wirkt schon irgendwie beeindruckend. Doch dadurch ist die Funktion abhängig von allen Funktionsblöcken, auch von denen, die nur indirekt über eine Referenz angesprochen werden.
Der Zugriff von refBuilding auf refFloors verstößt nicht gegen das Law of Demeter, da refFloors eine direkte Eigenschaft von FB_Building ist. Alle weiteren Zugriffe auf die Referenzen haben aber zur Folge, dass unsere Funktion auch von den anderen Funktionsblöcken abhängig wird.
Ändert sich z.B. die Struktur von FB_Room oder FB_Floor, so muss evtl. auch die Funktion zur Leistungsaufnahme angepasst werden.
Um das Law of Demeter einzuhalten, könnte jeder Funktionsblock eine Methode anbieten (CalcPowerConsumption()), in welcher die Leistungsaufnahme berechnet wird. In jeder dieser Methoden, wird wiederrum die darunter liegende Methode CalcPowerConsumption() aufgerufen:
Die Methode CalcPowerConsumption() in FB_Building greift nur auf die eigenen Elemente zu. In diesem Fall auf die Eigenschaft refFloors, um darüber die Methode CalcPowerConsumption() von FB_Floor aufzurufen:
METHOD CalcPowerConsumption : UDINT
VAR
nFloor : INT;
END_VAR
FOR nFloor := 1 TO 5 DO
CalcPowerConsumption := CalcPowerConsumption + refFloors[nFloor].CalcPowerConsumption();
END_FOR
In CalcPowerConsumption() von FB_Floor wird wiederrum nur auf FB_Room zugegriffen:
METHOD CalcPowerConsumption : UDINT
VAR
nRoom : INT;
END_VAR
FOR nRoom := 1 TO 20 DO
CalcPowerConsumption := CalcPowerConsumption + refRooms[nRoom].CalcPowerConsumption();
END_FOR
Zuletzt wird in FB_Room die Leistungsaufnahme aller Lampen in dem Raum berechnet:
METHOD CalcPowerConsumption : UDINT
VAR
nLamp : INT;
END_VAR
FOR nLamp := 1 TO 10 DO
CalcPowerConsumption := CalcPowerConsumption + refLamps[nLamp].nPowerConsumption;
END_FOR
Der Aufbau der Funktion F_CalcPowerConsumption() gestaltet sich dadurch deutlich einfacher:
FUNCTION F_CalcPowerConsumption : UDINT
VAR_INPUT
refBuilding : REFERENCE TO FB_Building;
END_VAR
IF (NOT __ISVALIDREF(refBuilding)) THEN
F_CalcPowerConsumption := 0;
RETURN;
END_IF
F_CalcPowerConsumption := refBuilding.CalcPowerConsumption();
F_CalcPowerConsumption() ist nach dieser Anpassung nur noch abhängig von FB_Building und dessen Methode CalcPowerConsumption(). Wie FB_Building in CalcPowerConsumption() die Leistungsaufnahme berechnet, ist für F_CalcPowerConsumption() ohne Bedeutung. Der Aufbau von FB_Room oder FB_Floor könnte sich komplett ändern, F_CalcPowerConsumption() müsste nicht angepasst werden.
Die erste Variante, in der durch alle Funktionsblöcke iteriert wurde, ist sehr anfällig gegenüber Änderungen. Egal bei welchem Funktionsblock sich der Aufbau ändert, eine Anpassung von F_CalcPowerConsumption() wäre jedes Mal notwendig.
Beispiel 1 (TwinCAT 3.1.4024) auf GitHub
Allerdings ist zu berücksichtigen, dass verschachtelte Strukturen durchaus Sinn ergeben. Hier muss das Law of Demeter nicht anwendet werden. So kann es hilfreich sein, die Konfigurationsdaten über mehrere Strukturen hierarchisch zu verteilen, um so die Lesbarkeit zu erhöhen.
Keep It Simple, Stupid (KISS)
Das KISS-Prinzip besagt, dass Code so „simple‟ wie möglich sein sollte, damit dieser möglichst einfach zu verstehen und somit effektiv zu warten ist. Hierbei sollte „simple‟ mit „schlicht‟ übersetzt werden. Damit ist eine Schlichtheit gemeint, die versucht Unnötiges wegzulassen aber weiterhin die Anforderungen des Kunden zu erfüllen. Durch die Beachtung des KISS-Prinzips wird ein System:
- einfach zu verstehen
- einfach zu erweitern
- einfach zu pflegen
Besteht die Anforderung darin zehn Millionen Datensätze zu sortieren, so wäre die Verwendung des Bubblesort-Algorithmus zwar einfach in der Umsetzung, doch wird die geringe Geschwindigkeit des Algorithmus nicht den Anforderungen des Kunden entsprechen. Es muss also immer eine Lösung gefunden werden, die den geforderten Erwartungen des Kunden entspricht und deren Umsetzung aber möglichst einfach (schlicht) ist.
Grundsätzlich sind zwei Arten von Anforderungen zu unterscheiden:
Funktionale Anforderung: Der Kunde bzw. Stakeholder fordert ein bestimmtes Leistungsmerkmal. Gemeinsam mit dem Kunden werden dann die genauen Anforderungen für dieses Leistungsmerkmal festgelegt und erst danach wird dieses implementiert. Funktionale Anforderungen erweitern eine Anwendung um eindeutige, von dem Kunden gewünschte, Funktionen (Leistungsmerkmale).
Nicht funktionale Anforderungen: Eine nicht funktionale Anforderung ist z.B. das Aufteilen einer Anwendung auf verschiedene Module oder das Vorsehen von Schnittstellen, um z.B. Unit-Tests zu ermöglichen. Nicht funktionale Anforderungen sind Leistungsmerkmale, die für den Kunden nicht unbedingt sichtbar sind. Diese können aber notwendig sein, damit das Softwaresystem gepflegt und gewartet werden kann.
Bei dem KISS-Prinzip geht es immer um die nicht funktionalen Anforderungen. Das „Wie‟ steht im Mittelpunkt. Also die Frage, wie die geforderten Funktionen erreicht werden. Das YAGNI-Prinzip, welches im folgenden Kapitel beschrieben wird, bezieht sich auf die funktionalen Anforderungen. Hier steht das „Was‟ im Mittelpunkt.
Das KISS-Prinzip kann auf mehrere Ebene angewendet werden:
Formatierung Quellcode
Der folgende Quellcode ist zwar sehr kompakt, doch wird hier das KISS-Prinzip verletzt, da dieser nur schwer zu verstehen und somit sehr fehleranfällig ist:
IF(x<=RT[k-1](o[n+2*j]))THEN WT[j+k](l AND NOT S.Q);END_IF;
IF(x>RI[k+1](o[n+2*k]))THEN WO[j-k](l OR NOT S.Q);END_IF;
Der Quellcode sollte so formatiert werden, dass der Ablauf besser erkannt wird. Auch sollten die Bezeichner für Variablen und Funktionen so gewählt werden, dass deren Bedeutung leichter zu verstehen ist.
Unnötiger Quellcode
Quellcode, der nicht dazu beiträgt, die Lesbarkeit zu verbessern, verletzt ebenfalls gegen das KISS-Prinzip:
bCalc := F_CalcFoo();
IF (bCalc = TRUE) THEN
bResult := TRUE;
ELSE
bResult := FALSE;
END_IF
Der Quellcode ist zwar gut strukturiert, auch wurden die Bezeichner so gewählt damit die Bedeutung leichter zu erkennen ist, doch kann der Quellcode deutlich reduziert werden:
Diese eine Zeile ist deutlich einfacher zu verstehen, wie die 6 Zeilen zuvor. Der Quellcode ist „schlichter‟, bei gleichem Funktionsumfang.
Softwaredesign / Softwarearchitektur
Auch das Design oder die Struktur einer Software kann gegen das KISS-Prinzip verstoßen. Wird z.B. für das Abspeichern von Konfigurationsdaten eine komplette SQL-Datenbank eingesetzt, obwohl eine Textdatei ausreichen würde, so wird ebenfalls das KISS-Prinzip verletzt.
Das Aufteilen eines SPS-Programms auf mehrere CPU-Cores ist nur dann sinnvoll, wenn es auch einen praktischen Nutzen hervorbringt. In einem SPS-Programm müssen in diesem Fall entsprechende Mechanismen eingebaut werden, um den Zugriff auf gemeinsame Ressourcen zu synchronisieren. Diese erhöhen die Komplexität des Systems erheblich und sollten nur dann zum Einsatz kommen, wenn die Anwendung dieses auch erfordert.
Ganz bewusst habe ich die Kapitel zu dem KISS-Prinzip und zu dem YAGNI-Prinzip an das Ende gesetzt. Von hier aus möchte ich nochmal einen kurzen Rückblick auf den Anfang der Serie über die SOLID-Prinzipien werfen.
Bei der Vorstellung der SOLID-Prinzipien habe ich gelegentlich auf die Gefahr des Overengineering hingewiesen. Abstraktionen sollten nur dann vorgesehen werden, wenn diese für die Umsetzung von Features notwendig sind.
Um dieses zu verdeutlichen, will ich das Beispiel für die Erklärung der SOLID-Prinzipien noch einmal verwenden (siehe: IEC 61131-3: SOLID – Das Dependency Inversion Principle).
Zwischen den drei Lampentypen und dem Controller besteht eine feste Abhängigkeit. Soll die Anwendung um einen weiteren Lampentyp erweitert werden, so ist es notwendig das Programm an verschiedenen Stellen anzupassen. Durch das Anwenden des Dependency Inversion Principle (DIP) und des Single Responsibility Principle (SRP) wurde das Programm deutlich flexibler. Das Integrieren von zusätzlichen Lampentypen wurde dadurch signifikant vereinfacht. Aber auch die Komplexität des Programms wurde durch diese Anpassungen deutlich größer, wie das UML-Diagramm zeigt:
(abstrakte Elemente werden in kursiver Schriftart dargestellt)
Bevor zusätzliche Abstraktionsebenen durch die Anwendung der SOLID-Prinzipien realisiert werden, sollte man den Mehraufwand immer kritisch hinterfragen.
Die erste Variante ist vom Aufbau vollkommen ausreichend, wenn das Programm in diesem Umfang ausschließlich in einem Projekt eingesetzt wird. Das Programm ist klein genug, um den Aufbau der Software zu verstehen und um kleine Anpassungen vorzunehmen. Das KISS-Prinzip wurde befolgt. Es wurde nicht mehr Komplexität als notwendig eingebaut.
Ist die erste Variante allerdings nur ein Zwischenschritt, z.B. bei der Entwicklung eines umfangreichen Lichtmanagementsystem, so ist damit zu rechnen, dass die Anwendung an Komplexität noch zunehmen wird. Auch ist es möglich, dass zu einem späteren Zeitpunkt die Entwicklung auf mehrere Personen verteilt werden muss. Der Einsatz von Unit-Tests ist ein weiterer Punkt, der die Umsetzung der SOLID-Prinzipien rechtfertigt. Ohne die Entkopplung der einzelnen Lampentypen durch Schnittstellen, ist der Einsatz von Unit-Tests nur schwer bzw. gar nicht möglich. Auch hier wird das KISS-Prinzip nicht verletzt. Das KISS-Prinzip muss somit immer im Kontext betrachtet werden.
You Ain’t Gonna Need It (YAGNI)
YAGNI steht für You Ain’t Gonna Need It und bedeutet frei übersetzt Du wirst es nicht brauchen. Es besagt, dass man in der Softwareentwicklung nur die Leistungsmerkmale realisieren sollte, die benötigt werden. Es sollen keine Funktionen oder Features implementiert werden, die vielleicht irgendwann einmal gebraucht werden könnten.
Im Gegensatz zu dem KISS-Prinzip, bei dem es immer um die nicht funktionalen Anforderungen geht, liegt der Fokus bei dem YAGNI-Prinzip auf den funktionalen Anforderungen.
Bei der Entwicklung von Software kann die Versuchung groß sein, zusätzliche Leistungsmerkmale ohne konkrete Anforderung zu implementieren. Das kann z.B. dann der Fall sein, wenn während der Entwicklung Leistungsmerkmale ohne Absprache mit dem Kunden implementiert werden, in dem festen Glauben, dass der Kunde diese später noch fordern wird.
Bezogen auf unser obiges Beispiel, wird das YAGNI-Prinzip dann verletzt, wenn man die Betriebsstundenerfassung implementieren würde (siehe: IEC 61131-3: SOLID – Das Interface Segregation Principle), obwohl dieses vom Kunden nicht gefordert wurde.
Wird während der Entwicklung festgestellt, dass ein bestimmtes Leistungsmerkmal sinnvoll sein könnte, so sollte die Implementierung erst nach Absprache mit dem Kunden erfolgen. Ansonsten erhält ein System nach und nach immer mehr Quellcode für Leistungsmerkmale, die niemand benötigt.
Durch dieses Beispiel wird noch einmal deutlich, dass alle bisher beschriebenen Prinzipien keine festen Regeln oder gar Gesetze sind. Die Prinzipien sind aber ein mächtiges Werkzeug, um die Codequalität von Software zu verbessern.
Wieder mal eine tolle Geschichte wie Kunden auf uns als Softwarehersteller sauer werden, weil Microsoft ein nicht funktionierendes Update veröffentlicht.
Die Story:
- Wir nutzen intern COM für sehr viele Objekte, um unsere eigene Software via VB-Script zu steuern.
- Wir haben auch die Möglichkeit Controls vom Typ WebView2 anzulegen.
- Am 07.12. veröffentlichte Microsoft für den WebView2 den Build 120.
- Unsere Software benutzt im Allgemeinen „Evergreen“, d.h. es wird immer die aktuelle WebView2 ohne eigne Installation benutzt.
Effekt:
Seit dem Update kann man nach dem, ein WebView2 Fenster zerstört wurde, keine COM Class Factory in unserem Programm aufrufen.
Intern scheint das WebView2 CoSuspendClassObjects aufzurufen wenn das Control zerstört wird. Die Folge unser IMessageFilter springt an und es kommt ein Dialog, der auf einen nicht reagierenden COM Server hinweist.
Der nicht reagierende COM-Server ist unsere eigene Anwendung… 
Toll! 
Einziger für uns möglicher Workaround für uns ist leider, die alte Version 119 auf jedem Client lokal zu installieren. Dann über einen Registry Eintrag (HKEY_LOCAL_MACHINE\SOFTWARE\Policies\Microsoft\Edge\WebView2\BrowserExecutableFolder) den Aufruf von der aktuellen Version umzubiegen.
Netterweise kann man das für jede Anwendung separat steuern.
Details zum Nachlesen auf GitHub.
Nachtrag: Der Bug verschwand mit dem Update 120.0.2210.77 in der evergreen Version. Bei mir wurde der Fix am Montag den 18.12.2023 automatisch installiert.
Copyright © 2017 Martin Richter
Dieser Feed ist nur für den persönlichen, nicht gewerblichen Gebrauch bestimmt. Eine Verwendung dieses Feeds bzw. der hier veröffentlichten Beiträge auf anderen Webseiten bedarf der ausdrücklichen Genehmigung des Autors.
(Digital Fingerprint: bdafe67664ea5aacaab71f8c0a581adf)
Leidvoll musste ich erleben, dass ein Visual Studio 2022 Rollback auf die ältere vormals installierte Version leider auch einige VS-Extensions deinstalliert. Bzw. diese gehen verloren.
Man sollte also einen Rollback mit Vorsicht verwenden. Er eignet sich also nicht einfach und schnell ein Problem zu umgehen. Es sind einige Nacharbeiten nötig.
Copyright © 2017 Martin Richter
Dieser Feed ist nur für den persönlichen, nicht gewerblichen Gebrauch bestimmt. Eine Verwendung dieses Feeds bzw. der hier veröffentlichten Beiträge auf anderen Webseiten bedarf der ausdrücklichen Genehmigung des Autors.
(Digital Fingerprint: bdafe67664ea5aacaab71f8c0a581adf)
In der letzten Zeit habe ich regelmäßig die aktuellsten Visual-Studio 2022 installiert. Ich muss ehrlich sagen, dass ich seit VS-2029 nicht einmal schlechte Erfahrungen gemacht habe.
Das hat sich mit dem heutigen Tag geändert
!
Nach der Installation des Updates ging keine Step-Debug-Funktion mehr (Step-In, Step-Over, Step-Out, etc.) im Mixed Mode Debugging. Native Mode Debugging scheint zu gehen.
Egal was man macht man bekommt den Fehler:
Unable to step. Operation not supported. Unknown error: 0x8ede0018.
In der Developer Community für VS ist dieser Bug auch bereits bekannt und angeblich gibt es einen Fix. Der ist aber noch nicht öffentlich.
Leider arbeite ich an einigen C++/CLI Modulen und benötige den Mixed-Mode.
Das erste mal habe im Visual Studio Installer einen Rollback versucht. Leider hat der Rollback meine Extension zum Teil deinstalliert. Mein Visual Assist von Whole Tomato war auf einmal nicht mehr vorhanden. Toll… 
Nachtrag: Das Problem ist in der Version 17.8.4 behoben, die am 10.01.2024 veröffentlicht wurde.
Copyright © 2017 Martin Richter
Dieser Feed ist nur für den persönlichen, nicht gewerblichen Gebrauch bestimmt. Eine Verwendung dieses Feeds bzw. der hier veröffentlichten Beiträge auf anderen Webseiten bedarf der ausdrücklichen Genehmigung des Autors.
(Digital Fingerprint: bdafe67664ea5aacaab71f8c0a581adf)